Defect recognition in line-space patterns aided by deep learning with data augmentation
JOURNAL OF MICRO-NANOPATTERNING MATERIALS AND METROLOGY-JM3(2021)
摘要
Background: Finding optimal processing conditions to reduce defectivity is a major challenge in high-resolution lithographic tools such as directed self-assembly and extreme ultraviolet lithography. Aim: We aim to develop an efficient automated method that can detect defects and identify their types and locations, allowing for assessing the performance of lithographic processing conditions more easily. Approach: We propose a deep learning approach using an object recognition neural network for the classification and detection of defects in scanning electron microscopy (SEM) images featuring line-space patterns. We optimized our network by exploring its design variables and applied it on a case with limited numbers of SEM images available for training the network. Results: With an optimized network and data augmentation strategies (flipping and mixing images from simulations), it was possible to achieve a significant increase in the performance of the network. Transferability of the network was also proven when applied on a more diverse dataset of SEM images gathered from selected publications. Conclusions: The outcome of our work shows that the amalgamation of an optimal network design and augmentation strategies performs satisfactorily for defectivity analysis and is generic for data not constrained to fixed settings. (c) The Authors. Published by SPIE under a Creative Commons Attribution 4.0 International License. Distribution or reproduction of this work in whole or in part requires full attribution of the original publication, including its DOI. [DOI: 10.1117/1.JMM.20.4.041203]
更多查看译文
关键词
lithography, neural networks, defects, line-space patterns, data augmentation
AI 理解论文
溯源树
样例
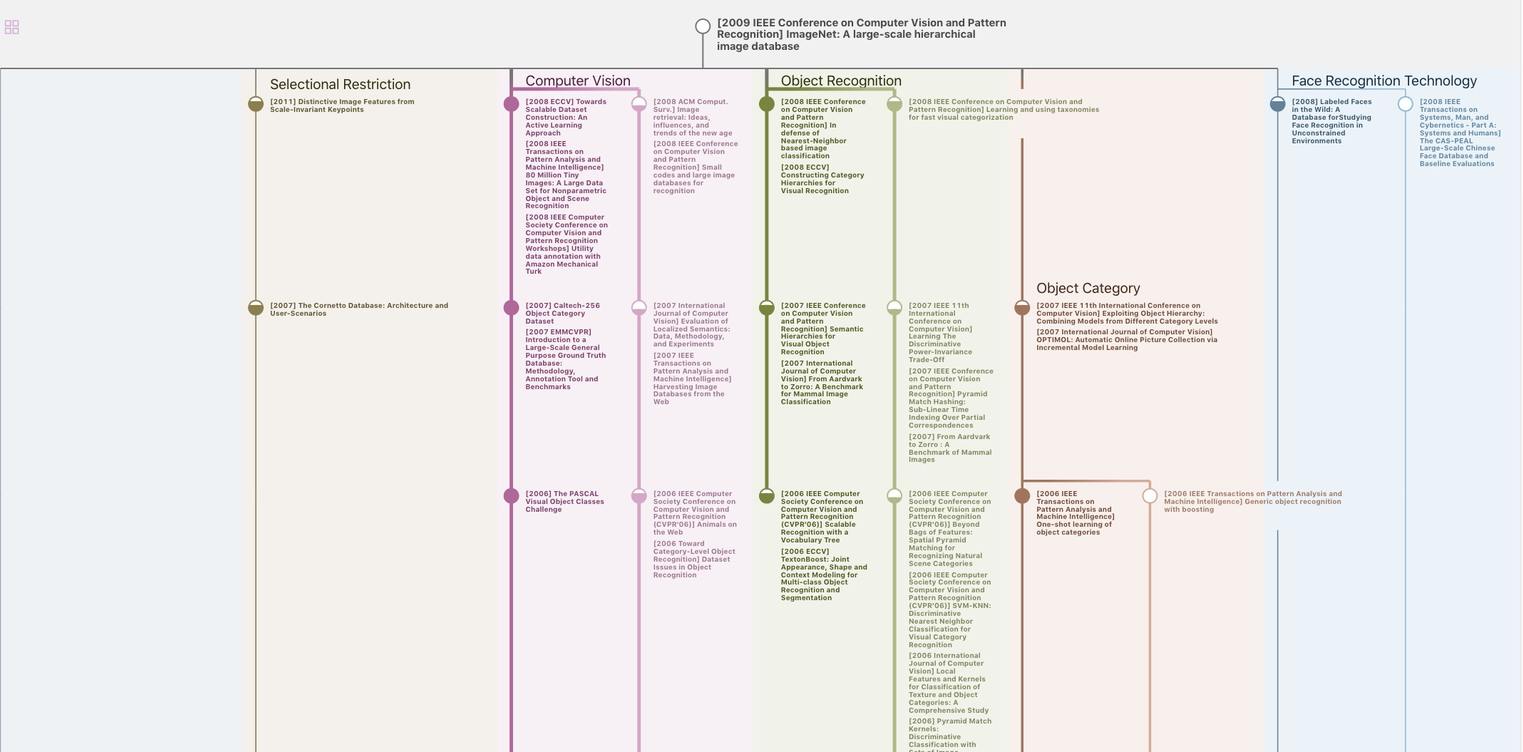
生成溯源树,研究论文发展脉络
Chat Paper
正在生成论文摘要