Online Shape Modeling of Resident Space Objects Through Implicit Scene Understanding
JOURNAL OF AEROSPACE INFORMATION SYSTEMS(2022)
摘要
Neural networks have become state-of-the-art computer vision tools for tasks that learn implicit representations of geometrical scenes. This paper proposes a two-part network architecture that exploits a view-synthesis network to understand a context scene and a graph convolutional network to generate a shape body model of an object within the field of view of a spacecraft's optical navigation sensors. Once the first part of the network's architecture understands the spacecraft's environment, it can generate images from novel observations. The second part uses a multiview set of images to construct a 3D graph-based representation of the object. The proposed network pipeline produces shape models with accuracies that compete with state-of-the-art methods currently used for missions to small bodies. The network pipeline can be trained for multi-environment missions. Moreover, the onboard implementation may be more cost-effective than the current state-of-the-art.
更多查看译文
关键词
Resident Space Object,Neural Networks,Computer Vision,Sensors
AI 理解论文
溯源树
样例
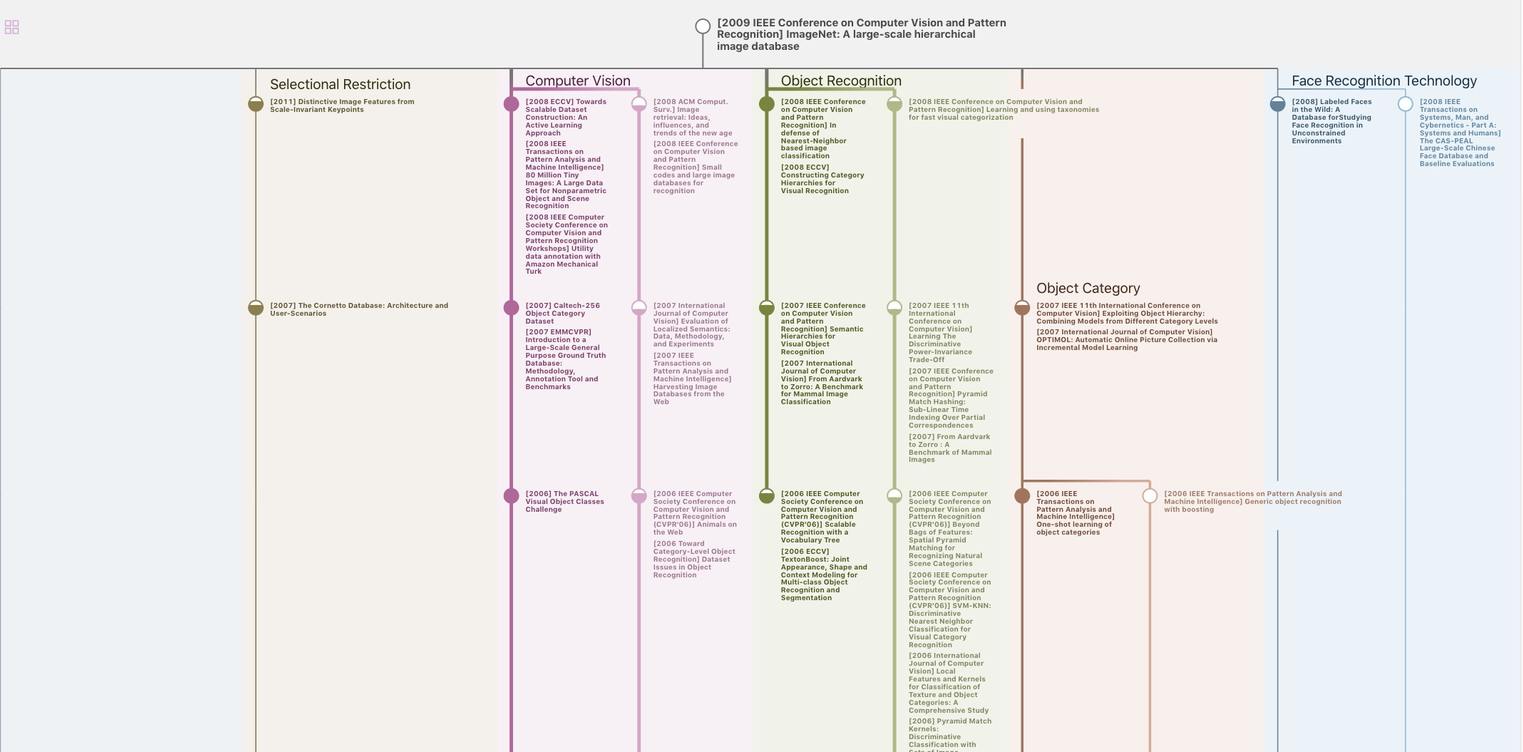
生成溯源树,研究论文发展脉络
Chat Paper
正在生成论文摘要