Exploring the Influence of Focal Loss on Transformer Models for Imbalanced Maintenance Data in Industry 4.0
IFAC PAPERSONLINE(2021)
摘要
Harnessing data from historical maintenance databases may be challenging, as they tend to rely on text data provided by operators. Thus, they often include acronyms, jargon, typos, and other irregularities that complicate the automated analysis of such reports. Furthermore, maintenance datasets may present highly imbalanced distributions: some situations happen more often than others, which hinders the effective application of classic Machine Learning (ML) models. Hence, this paper explores the use of a recent Deep Learning (DL) architecture called Transformer, which has provided cutting-edge results in Natural Language Processing (NLP). To tackle the class imbalance, a loss function called Focal Loss (FL) is explored. Results suggests that when all the classes are equally important, the FL does not improve the classification performance. However, if the objective is to detect the minority class, the FL achieves the best performance, although by degrading the detection capacity for the majority class. Copyright (C) 2021 The Authors.
更多查看译文
关键词
Artificial Intelligence, Natural Language Processing, Predictive Maintenance, Imbalanced Classification, Deep Learning, Transformers, Transfer Learning.
AI 理解论文
溯源树
样例
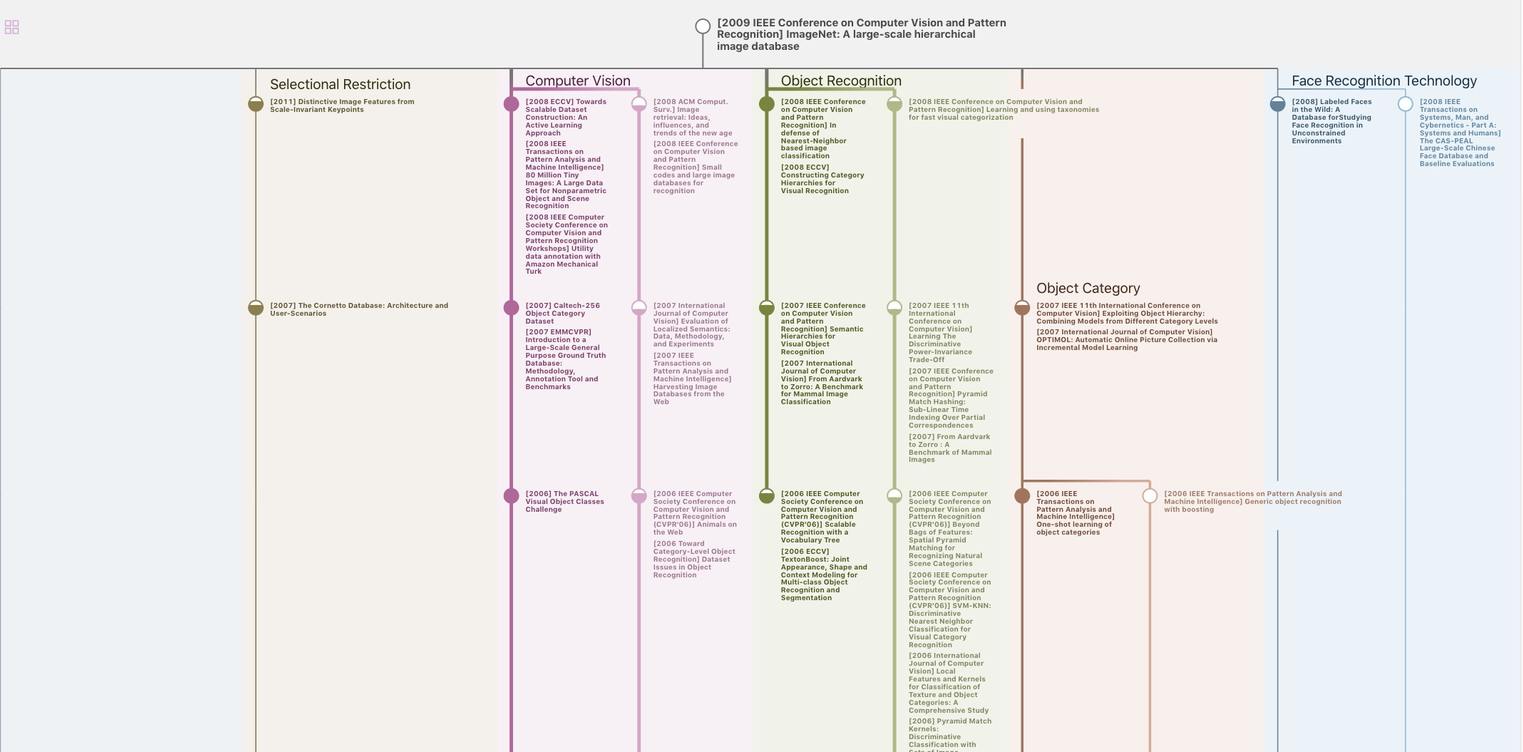
生成溯源树,研究论文发展脉络
Chat Paper
正在生成论文摘要