Evaluation of Deep Neural Network ProSPr for Accurate Protein Distance Predictions on CASP14 Targets
INTERNATIONAL JOURNAL OF MOLECULAR SCIENCES(2021)
摘要
The field of protein structure prediction has recently been revolutionized through the introduction of deep learning. The current state-of-the-art tool AlphaFold2 can predict highly accurate structures; however, it has a prohibitively long inference time for applications that require the folding of hundreds of sequences. The prediction of protein structure annotations, such as amino acid distances, can be achieved at a higher speed with existing tools, such as the ProSPr network. Here, we report on important updates to the ProSPr network, its performance in the recent Critical Assessment of Techniques for Protein Structure Prediction (CASP14) competition, and an evaluation of its accuracy dependency on sequence length and multiple sequence alignment depth. We also provide a detailed description of the architecture and the training process, accompanied by reusable code. This work is anticipated to provide a solid foundation for the further development of protein distance prediction tools.
更多查看译文
关键词
protein,prediction,contact,distance,deep learning,alphafold,ProSPr,CASP,dataset,retrainable
AI 理解论文
溯源树
样例
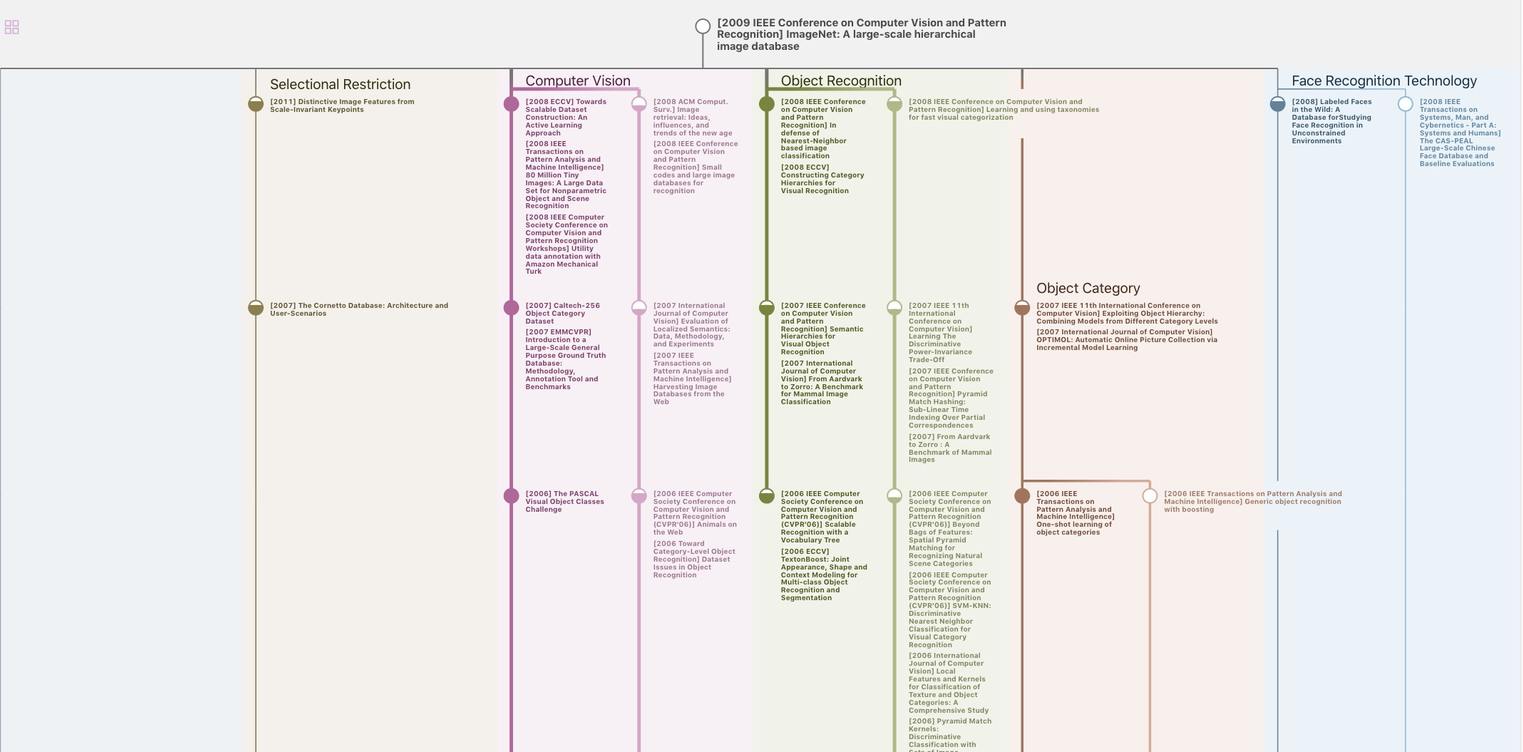
生成溯源树,研究论文发展脉络
Chat Paper
正在生成论文摘要