Multi-IMU Based Alternate Navigation Frameworks: Performance & Comparison for UAS
IEEE ACCESS(2022)
摘要
On-board state estimation is a persistent challenge to fielding unmanned aerial systems (UAS), particularly when global positioning system (GPS) measurements are not available. The dominant estimation tool remains the extended Kalman filter (EKF) applied to an inertial measurement unit (IMU). The growing availability of low-cost commercial-grade IMUs raises questions about how to best improve sensor readings into an estimate when measurements are available from multiple IMUs. This paper evaluates four different approaches to attitude estimation from multiple IMU measurements and their application in high dynamic motion. The four approaches are fusion of measurements (virtual IMU), fusion of state estimates (Federated KF), feedback fusion state estimate (Feedback Federated KF), and an EKF design incorporating the additional measurements (Augmented KF); these correspond to fusion before, within, or after state estimation. The performance of the approaches are quantified for varying IMU number theoretically and experimentally. The experiments use on-board autopilot hardware implementations of the estimators during maneuvers in a motion capture volume and flights on-board an Unmanned Aerial Systems (UAS) implementation, with the peak and root-mean-square (RMS) errors used to quantify accuracy. The RMS error results indicate that the feedback federated Kalman Filter using five IMUs returns 38% compared to general federated Kalman Filter using 37% accuracy improvement over a single IMU. This improvement compares to a 19% improvement for virtual IMU and 9% improvement for the Augmented KF respectively. These results indicate that both the Federated KF approach achieves the lowest RMS error relative to the virtual IMU and augmented KF approaches and inform the design of multi-IMU UAS pose estimators.
更多查看译文
关键词
Kalman filters, State estimation, Sensor fusion, Noise measurement, Measurement units, Software algorithms, Licenses, State estimation, Kalman filters, unmanned aerial systems, inertial navigation, quaternions, Bortz estimator, multi-IMU, inertial measurement unit, autopilot, attitude estimation
AI 理解论文
溯源树
样例
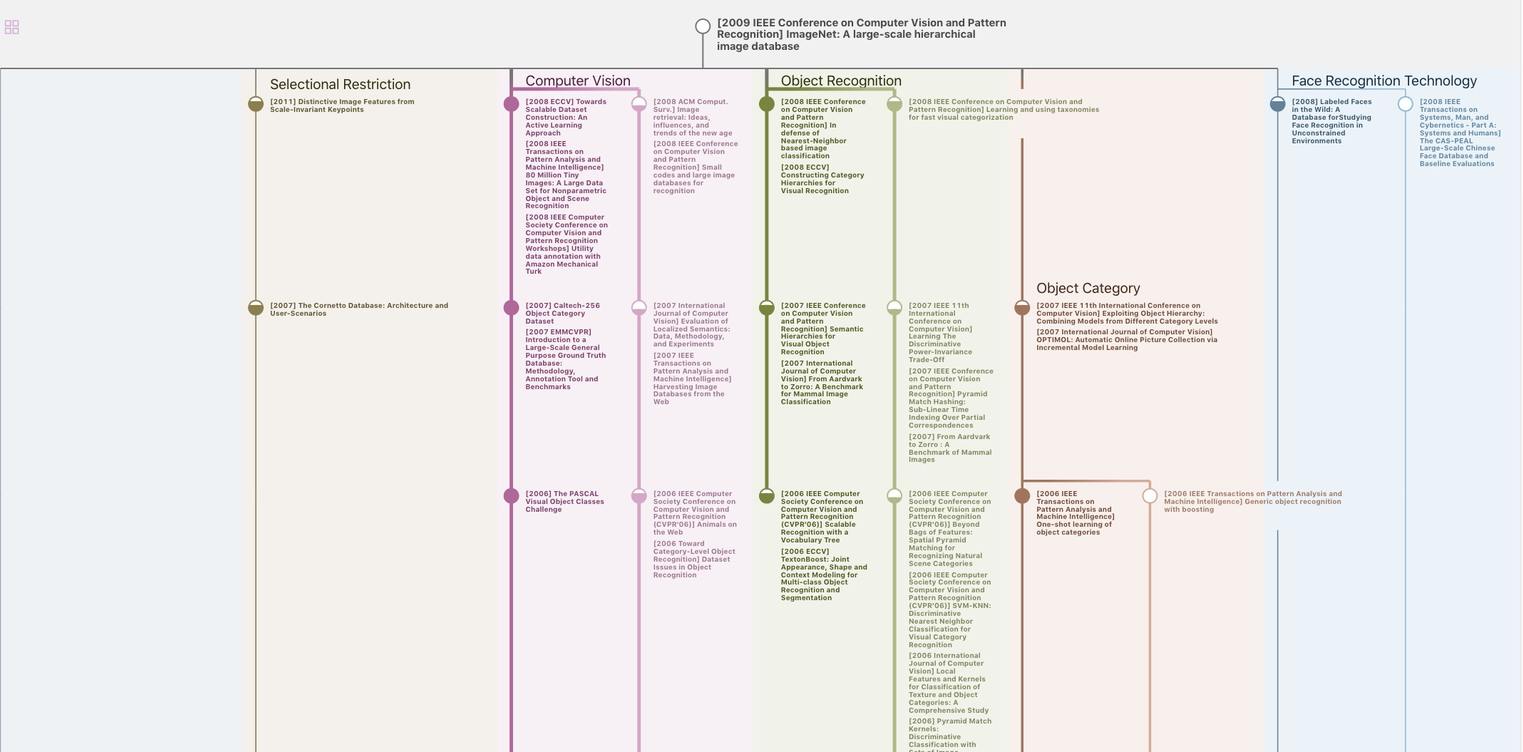
生成溯源树,研究论文发展脉络
Chat Paper
正在生成论文摘要