High Order Deep Neural Network for Solving High Frequency Partial Differential Equations
COMMUNICATIONS IN COMPUTATIONAL PHYSICS(2022)
摘要
This paper proposes a high order deep neural network (HOrderDNN) for solving high frequency partial differential equations (PDEs), which incorporates the idea of "high order" from finite element methods (FEMs) into commonly-used deep neural networks (DNNs) to obtain greater approximation ability. The main idea of HOrderDNN is introducing a nonlinear transformation layer between the input layer and the first hidden layer to form a high order polynomial space with the degree not exceeding p, followed by a normal DNN. The order p can be guided by the regularity of solutions of PDEs. The performance of HOrderDNN is evaluated on high frequency function fitting problems and high frequency Poisson and Helmholtz equations. The results demonstrate that: HOrderDNNs(p > 1) can efficiently capture the high frequency information in target functions; and when compared to physics-informed neural network (PINN), HOrderDNNs(p > 1) converge faster and achieve much smaller relative errors with same number of trainable parameters. In particular, when solving the high frequency Helmholtz equation in Section 3.5, the relative error of PINN stays around 1 with its depth and width increase, while the relative error can be reduced to around 0.02 as p increases (see Table 5).
更多查看译文
关键词
subject Deep neural network, high order methods, high frequency PDEs
AI 理解论文
溯源树
样例
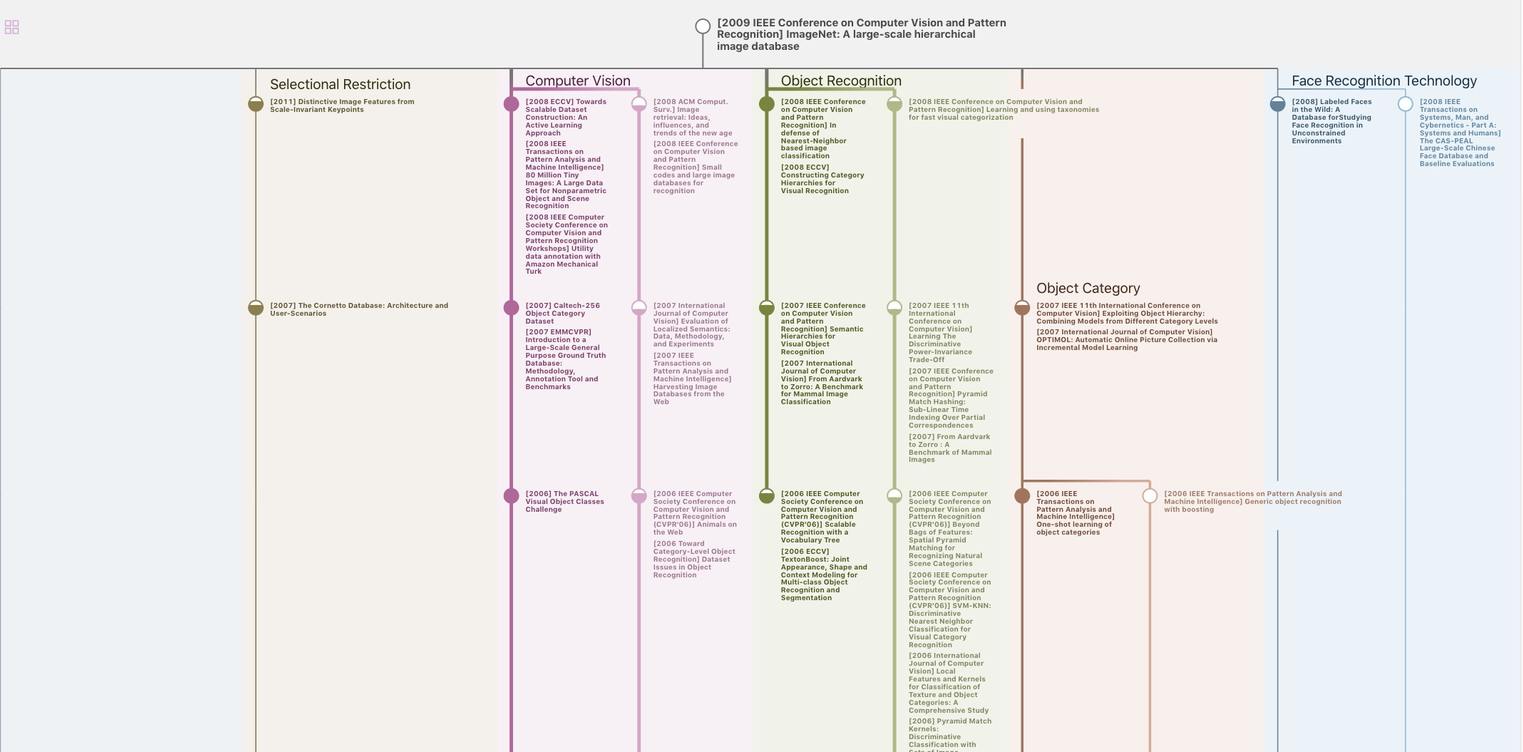
生成溯源树,研究论文发展脉络
Chat Paper
正在生成论文摘要