Deep Learning Based Anomaly Detection for Muti-dimensional Time Series: A Survey
CYBER SECURITY, CNCERT 2021(2022)
摘要
Multi-dimensional time series are multiple sets of variables collected in chronological order, which are the results of observing a certain potential process according to a given sampling rate. It also has the ability to describe space and time and is widely used in many fields such as system state anomaly detection. However, multi-dimensional time series have problems such as dimensional explosion and data sparseness, as well as complex pattern features such as periods and trends. Such characteristics lead to rule-based anomaly detection methods suffer from poor detection effects. In the big data scenario, deep learning method begins to be applied to anomaly detection tasks for multi-dimensional time series due to its wide coverage and strong learning ability. This work first summarizes the definitions of anomaly detection for multi-dimensional time series and the challenges it faces. Related methods are sorted out, and then the deep learning-based method is emphasized. The existing work and its advantages and disadvantages are summarized. Finally, the shortcomings of the existing algorithms are clarified and the future research direction is explored.
更多查看译文
关键词
Anomaly detection, Muti-dimensional time series, Machine learning, Deep learning
AI 理解论文
溯源树
样例
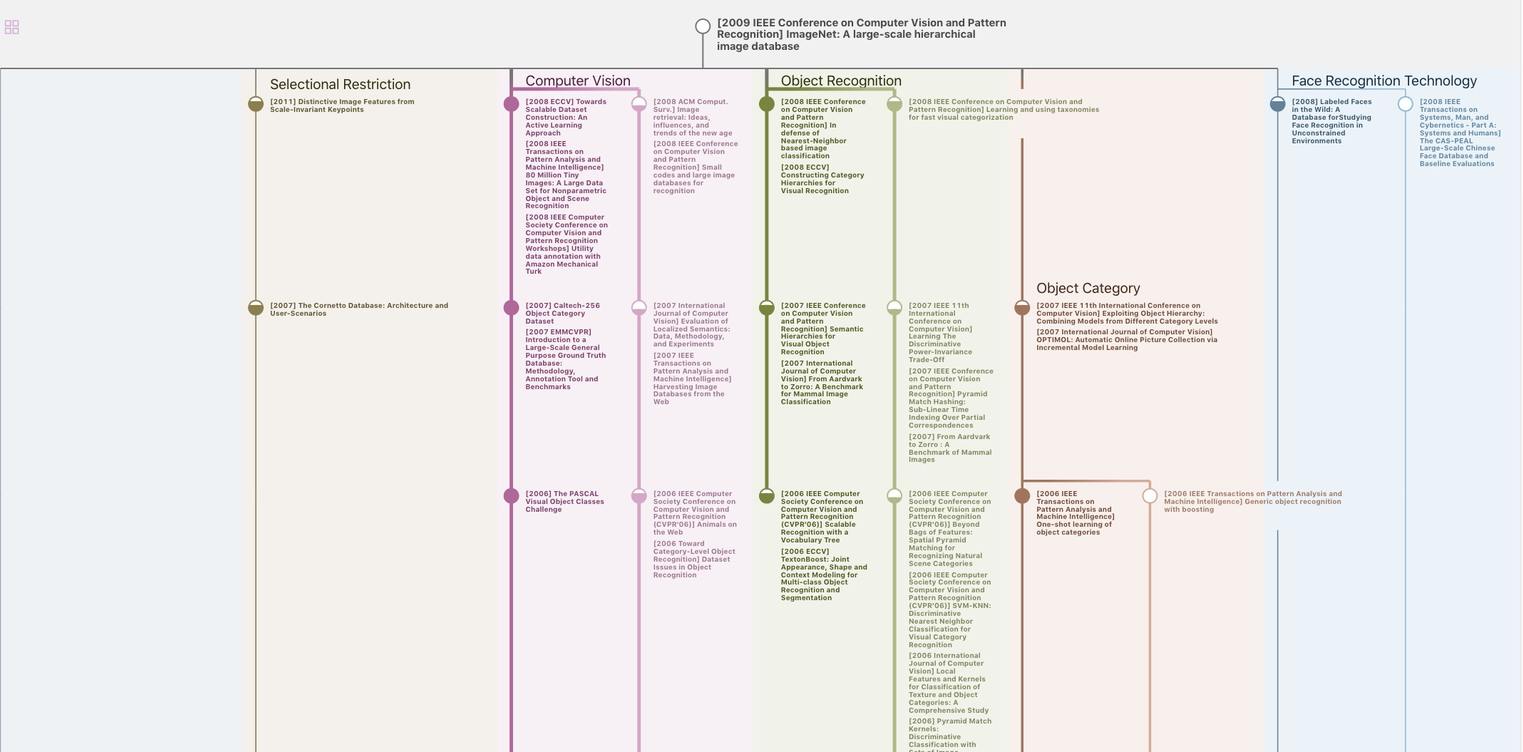
生成溯源树,研究论文发展脉络
Chat Paper
正在生成论文摘要