Smooth tensor robust principal component analysis with application to color image recovery
DIGITAL SIGNAL PROCESSING(2022)
摘要
In this work, we study the problem of tensor robust principal component analysis (TRPCA). The core idea is to decompose a given tensor into the superposition of a low-rank and sparse tensor and to recover each component individually. The key to the TRPCA approach is to efficiently characterize the low-rankness of the underlying tensor. Recently, the tensor train rank (TT) based on an augmentation technique, which transforms a lower-order tensor to a higher-order one, has successfully been used to characterize the low-rankness prior. However, the TT rank-based augmentation technique leads to visible block artifacts. Moreover, using only the TT rank may generally be insufficient to capture local information in data. Therefore, in this paper, we consider a novel model for the TRPCA model based on an edge-preserving smoothing prior to recover a low-rank tensor corrupted by sparse noise. The proposed model is named smooth-TRPCA. On the theoretical side, we adapt the alternating direction method of multipliers to solve the minimization problem. The superiority of our model is numerically reported via several experiments conducted on colors images. (c) 2022 Elsevier Inc. All rights reserved.
更多查看译文
关键词
TRPCA, Sparsity prior, TT rank, Tensor augmentation technique, Image recovery, Total variation
AI 理解论文
溯源树
样例
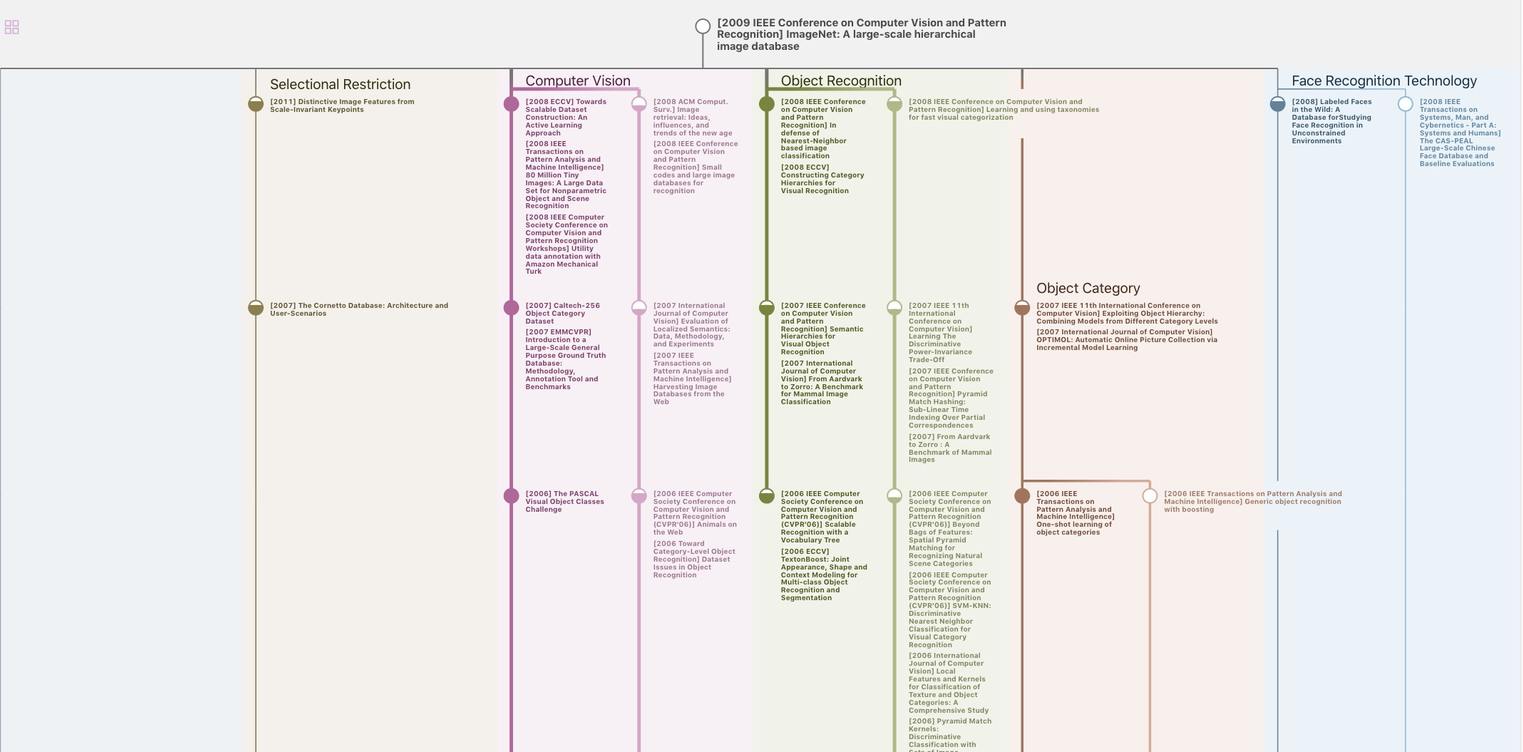
生成溯源树,研究论文发展脉络
Chat Paper
正在生成论文摘要