Robust multi-view non-negative matrix factorization for clustering
DIGITAL SIGNAL PROCESSING(2022)
摘要
Non-negative matrix factorization (NMF) has attracted much attention for multi-view clustering due to its good theoretical and practical values. Although existing multi-view NMF methods have achieved satisfactory performance to some extent, there still exist the following problems: 1) most existing methods only consider the first-order relationship to construct the similarity matrix, which cannot fully explore the neighborhood information; 2) most existing methods only consider the common information shared by different views, and the complementary information contained in different views is often ignored. To tackle the above-mentioned shortcomings, we present a novel multi-view clustering approach, called robust multi-view NMF (RMNMF). Specifically, in RMNMF, we consider the high-order relationship among features, where the first-order and second-order relationship is utilized to obtain an optimal neighborhood structure. Also, we introduce the Hilbert-Schmidt independence criterion (HSIC) to fully explore the complementary information in different views. Extensive experimental results on several benchmark datasets prove the effectiveness of our proposed approach. (C)& nbsp;2022 Elsevier Inc. All rights reserved.
更多查看译文
关键词
NMF, Multi-view learning, Graph Laplacian, Clustering
AI 理解论文
溯源树
样例
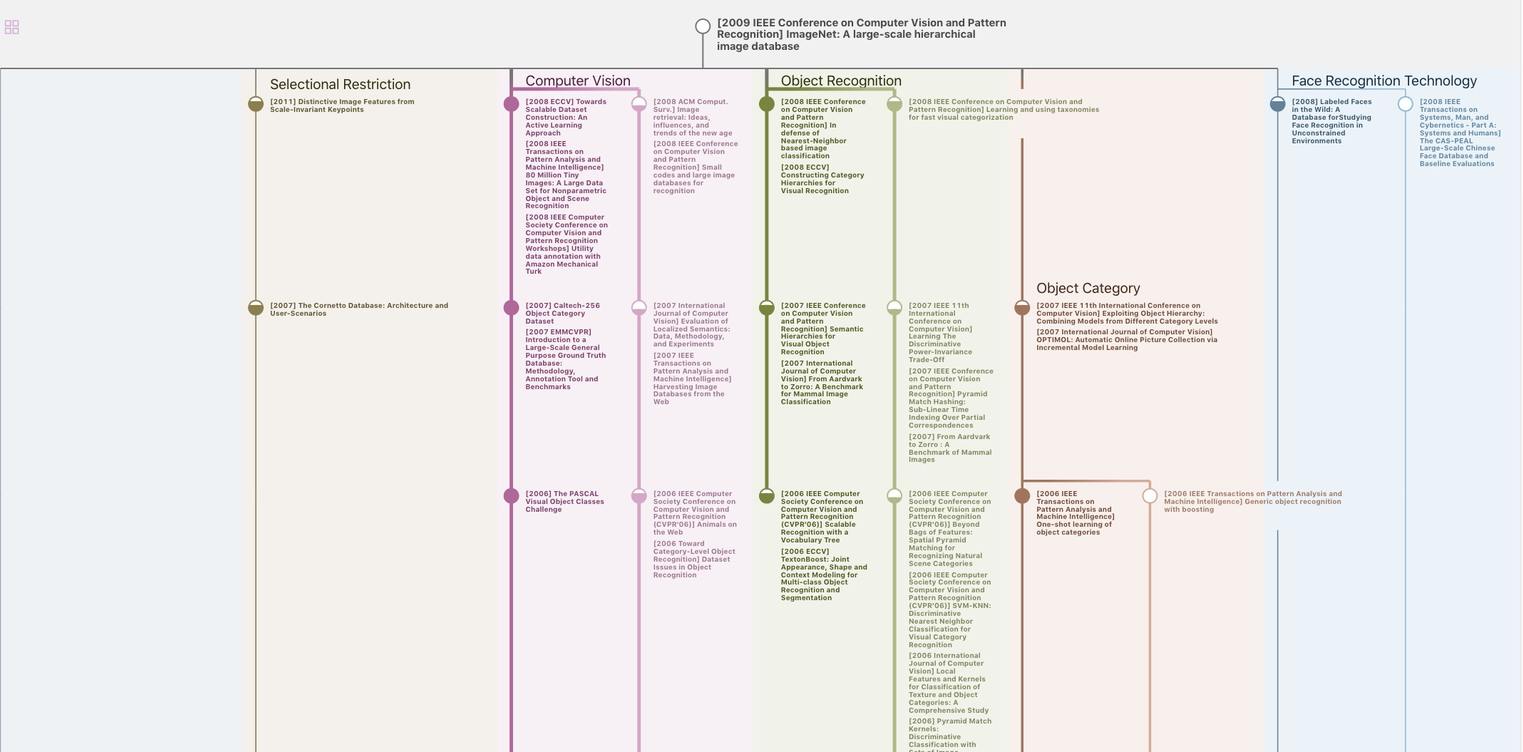
生成溯源树,研究论文发展脉络
Chat Paper
正在生成论文摘要