Global estimates of 500 m daily aerodynamic roughness length from MODIS data
ISPRS Journal of Photogrammetry and Remote Sensing(2022)
摘要
Aerodynamic roughness length (z0m) is a key parameter in the characterization of land surface turbulent heat fluxes and widely used in many surface and climate-related process models. The global products of time series of z0m at finer spatio-temporal resolution, however, have never been publicly available. Here we presented a practical method for global estimates of 500 m daily z0m with a combination of machine learning techniques, wind profile equation, observations from 273 sites and MODIS remote sensing data. Results showed that the random forest (RF) model outperformed the deep neural network (DNN) and convolutional neural network (CNN) models, and it could well reproduce the magnitude and temporal variability of daily z0m at almost all sites for all land cover types. In the validation of the RF-estimated daily z0m with the in-situ observations, the root mean square error (RMSE) varied between 0.02 m and 0.09 m, the mean absolute error (MAE) varied between 0.01 m and 0.05 m and the coefficient of determination (R2) was 1 for medium-to-high canopy shrublands, savannas and forests; for short-canopy croplands, grasslands and wetlands, the RMSE and MAE were 0.02 m and 0.01 m, respectively, and the R2 varied between 0.94 and 1. Compared to the Climate Forecast System Version 2 (CFSv2, 0.3°/monthly) and ECMWF Reanalysis v5 (ERA5, 0.25°/monthly) products in 2019, the RF-estimated z0m was found to have the similar global spatial pattern but significantly larger temporal variability, and it also showed a higher and lower global mean of z0m over forests and non-forests, respectively. The RF-estimated z0m displayed a higher temporal variability but a similar global spatial pattern of this variability compared to the CFSv2, whereas the ERA5 z0m product exhibited almost no temporal variability except for grasslands and croplands. This study is beneficial for improving the simulation of the momentum, water and energy transfer between land and atmosphere and helping boost the development of high-resolution land surface models and Earth system models.
更多查看译文
关键词
Aerodynamic roughness length,Machine learning,MODIS,Evapotranspiration
AI 理解论文
溯源树
样例
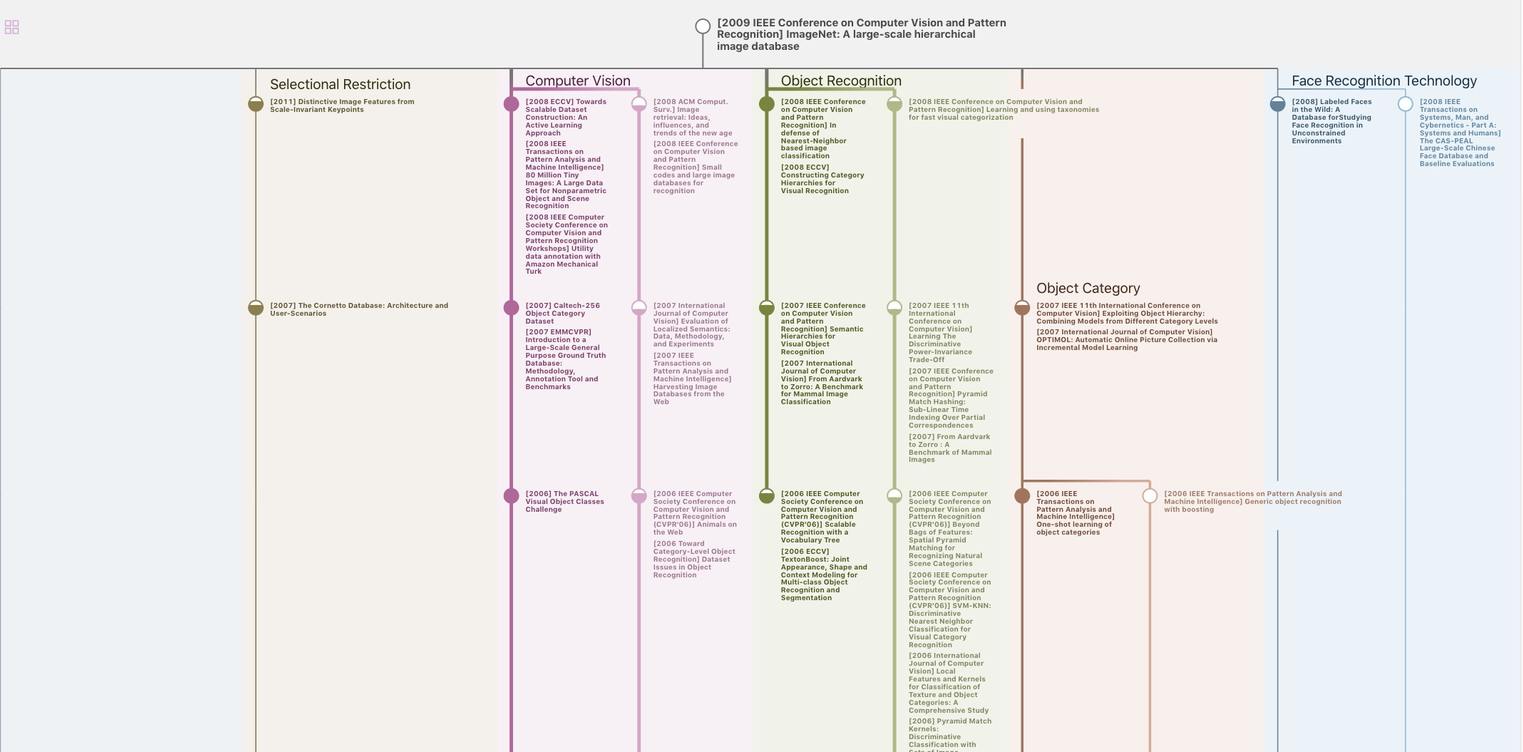
生成溯源树,研究论文发展脉络
Chat Paper
正在生成论文摘要