IoT-Based PV Array Fault Detection and Classification Using Embedded Supervised Learning Methods
ENERGIES(2022)
摘要
Faults on individual modules within a photovoltaic (PV) array can have a significant detrimental effect on the power efficiency and reliability of the entire PV system. In addition, PV module faults can create risks to personnel safety and fire hazards if they are not detected quickly. As IoT hardware capabilities increase and machine learning frameworks mature, better fault detection performance may be possible using low-cost sensors running machine learning (ML) models that monitor electrical and thermal parameters at an individual module level. In this paper, to evaluate the performance of ML models that are suitable for embedding in low-cost hardware at the module level, eight different PV module faults and their impacts on PV module output are discussed based on a literature review and simulation. The faults are emulated and applied to a real PV system, allowing the collection and labelling of panel-level measurement data. Then, different ML methods are used to classify these faults in comparison to the normal condition. Results confirm that NN obtain 93% classification accuracy for seven selected classes.
更多查看译文
关键词
photovoltaic system, PV faults, edge computing, machine learning, IOT, fault detection techniques, fault classification
AI 理解论文
溯源树
样例
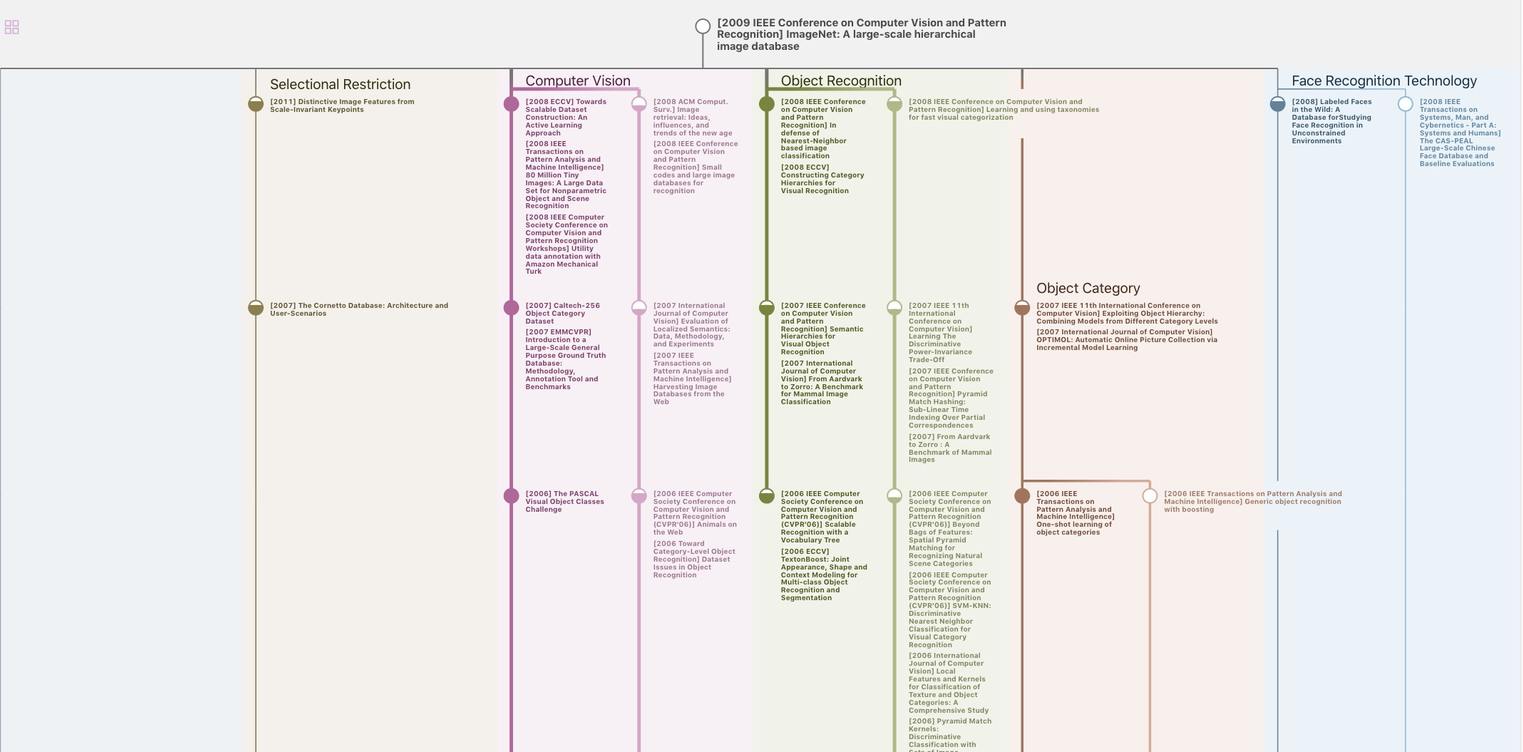
生成溯源树,研究论文发展脉络
Chat Paper
正在生成论文摘要