Kernel Principal Component Analysis for Structural Health Monitoring and Damage Detection of an Engineering Structure Under Operational Loading Variations
JOURNAL OF FAILURE ANALYSIS AND PREVENTION(2021)
摘要
This paper highlights kernel principal component analysis (KPCA) in distinguishing damage-sensitive features from the effects of liquid loading on frequency response. A vibration test is performed on an aircraft wing box incorporated with a liquid tank that undergoes various tank loading. Such experiment is established as a preliminary study of an aircraft wing that undergoes operational load change in a fuel tank. The operational loading effects in a mechanical system can lead to a false alarm as loading and damage effects produce a similar reduction in the vibration response. This study proposes a non-nonlinear transformation to separate loading effects from damage-sensitive features. Based on a baseline data set built from a healthy structure that undergoes systematic tank loading, the Gaussian parameter is measured based on the distance of the baseline data set to various damage states. As a result, both loading and damage features expand and are distinguished better. For novelty damage detection, Mahalanobis square distance (MSD) and Monte Carlo-based threshold are applied. The main contribution of this project is the nonlinear PCA projection to understand the dynamic behavior of the wing box under damage and loading influences and to differentiate both effects that arise from the tank loading and damage severities.
更多查看译文
关键词
Kernel PCA,Mahalanobis squared distance,Euclidean distance,Vibration-based damaged detection,Structural health monitoring
AI 理解论文
溯源树
样例
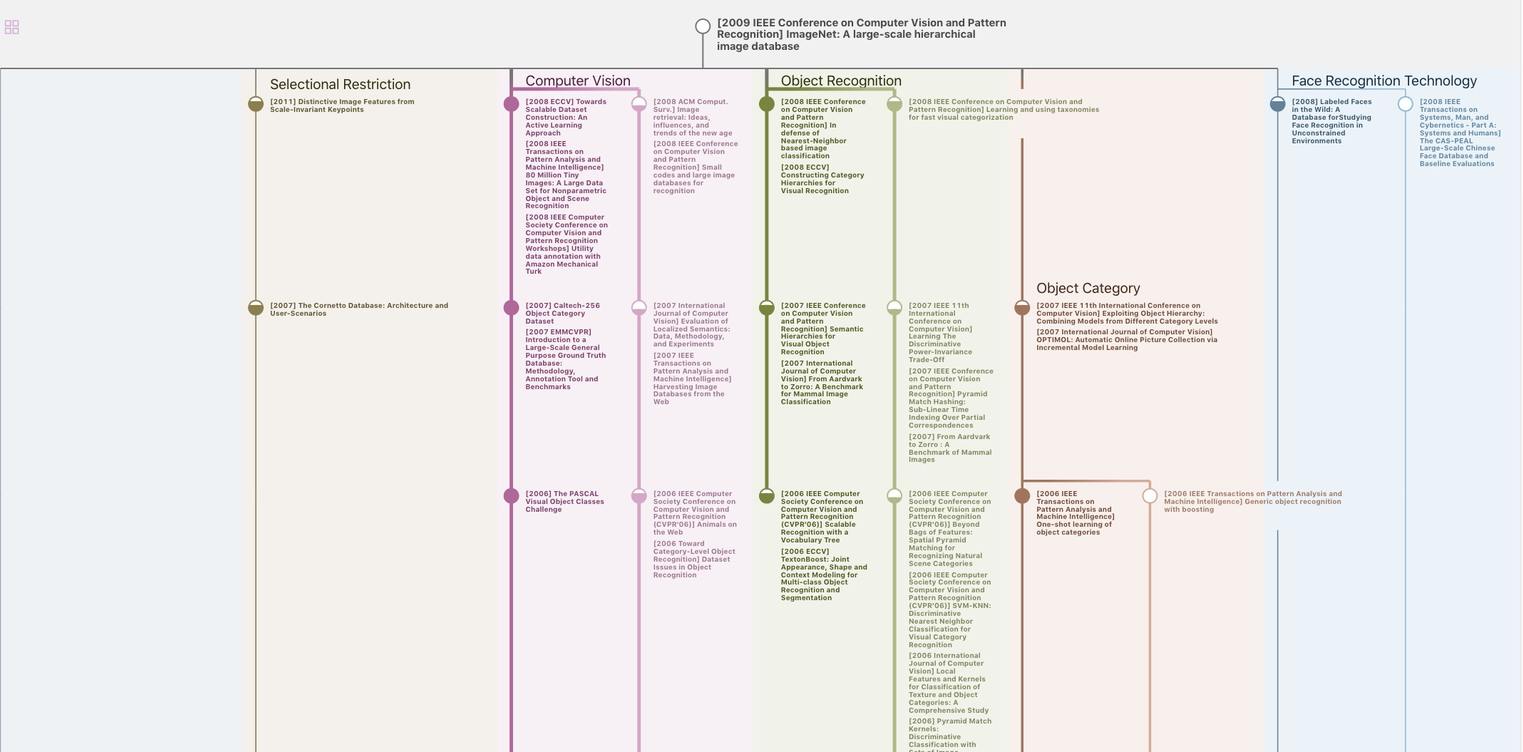
生成溯源树,研究论文发展脉络
Chat Paper
正在生成论文摘要