Experimentally Proven Bilateral Blur on SRCNN for Optimal Convergence
ADVANCED NETWORK TECHNOLOGIES AND INTELLIGENT COMPUTING, ANTIC 2021(2022)
摘要
Image super-resolution is a contentious issue in developing computer vision applications such as satellite imaging, graphics industry, medical diagnostics, and real-time scene monitoring for security and safety. CNN-based image super-resolution algorithms are the simplest and most resource-efficient in deep learning. The original SRCNN framework, on the other hand, incorporated Gaussian blur, which takes a long time to converge. This is not only computationally expensive, but it also extends the training time. In light of this, we retrained original SRCNN utilizing widely available blurring techniques and observed that the bilateral filter beats the Gaussian filter at optimal convergence. Our goal is to preserve the original SRCNN features while reducing training time and computational resources and hence, speed up the architecture with optimum number of epochs. This is, to the best of our knowledge, the first study of its kind, and no other study has implemented this alternative blurring training on SRCNN and ranked the best of them for image super-resolution.
更多查看译文
关键词
Blurring techniques, Image quality metrics, Super-resolution, SRCNN
AI 理解论文
溯源树
样例
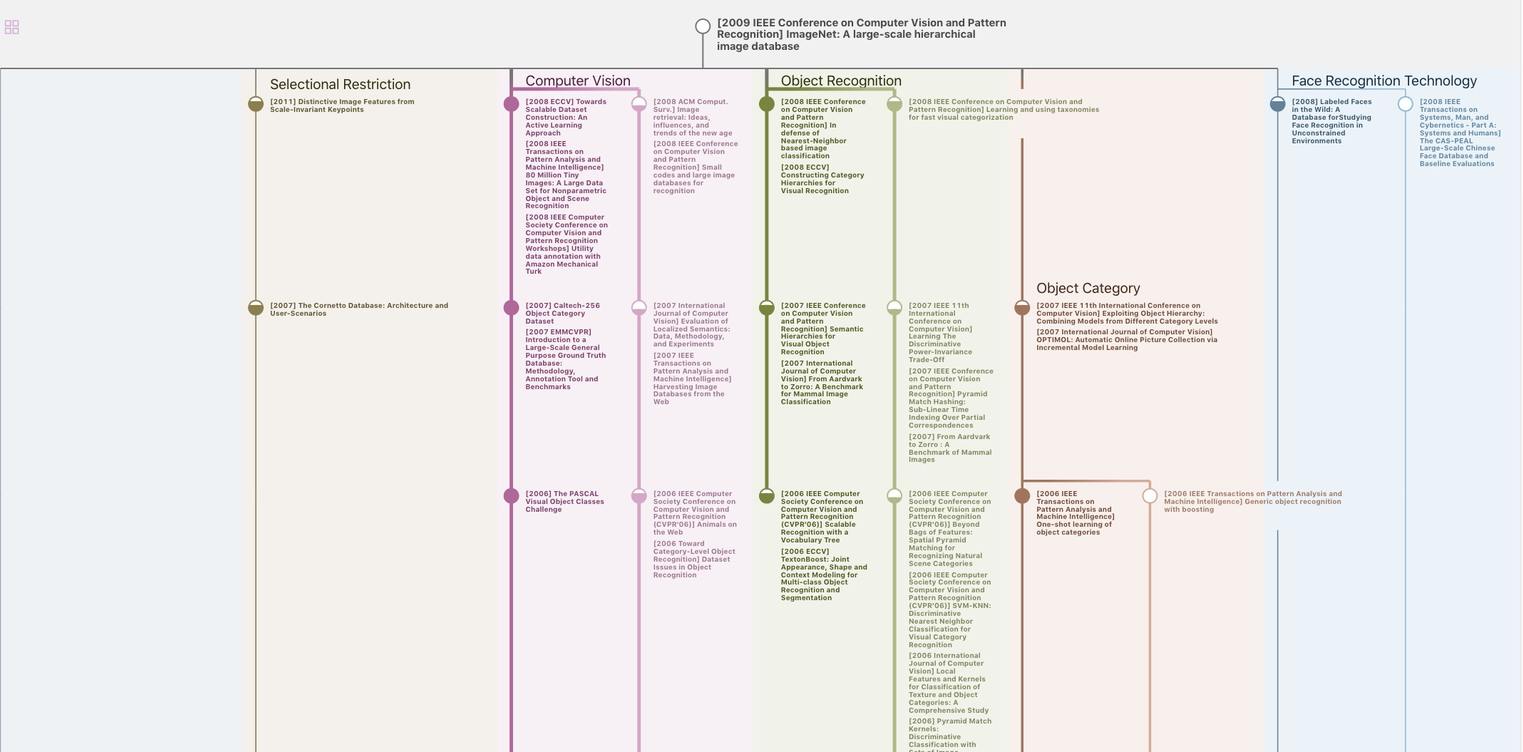
生成溯源树,研究论文发展脉络
Chat Paper
正在生成论文摘要