A Robust Malware Detection Approach for Android System Based on Ensemble Learning
UBIQUITOUS SECURITY(2022)
摘要
As the number of mobile devices which is based on the Android system continues to grow rapidly, it becomes a primary target for security exploitation through undesirable malicious apps (malware) being unwittingly downloaded, which is often due to negligent user behavior patterns that grant unnecessary permissions to malicious apps or simply malware evolving to be sophisticated enough to bypass systematic detection. There have been numerous attempts to use machine learning to capture an application's malicious behavior focusing on features deemed to be germane to high security risks, but most of them typically focus only on a single algorithm, which is not representative of a huge family of ensemble techniques. In this paper, we develop an ensemble learning based malware detection approach for the Android system. To validate the performance of the proposed approach, we have conducted some experiments on the real world Android app dataset, which contains 3618 features that are initially obtained from the static, dynamic and ICC analyses. We then select 567 important features through feature selection. The overall detection accuracy is 97.73%, accompanied by a high 97.66% F-1 score that reflects a high relationship between precision (97.06%) and recall (98.28%). The experimental results clearly show that the ensemble learning based malware detection approach could effectively identify malware for the Android system.
更多查看译文
关键词
Android, Security, Malware, Machine learning, Ensemble learning
AI 理解论文
溯源树
样例
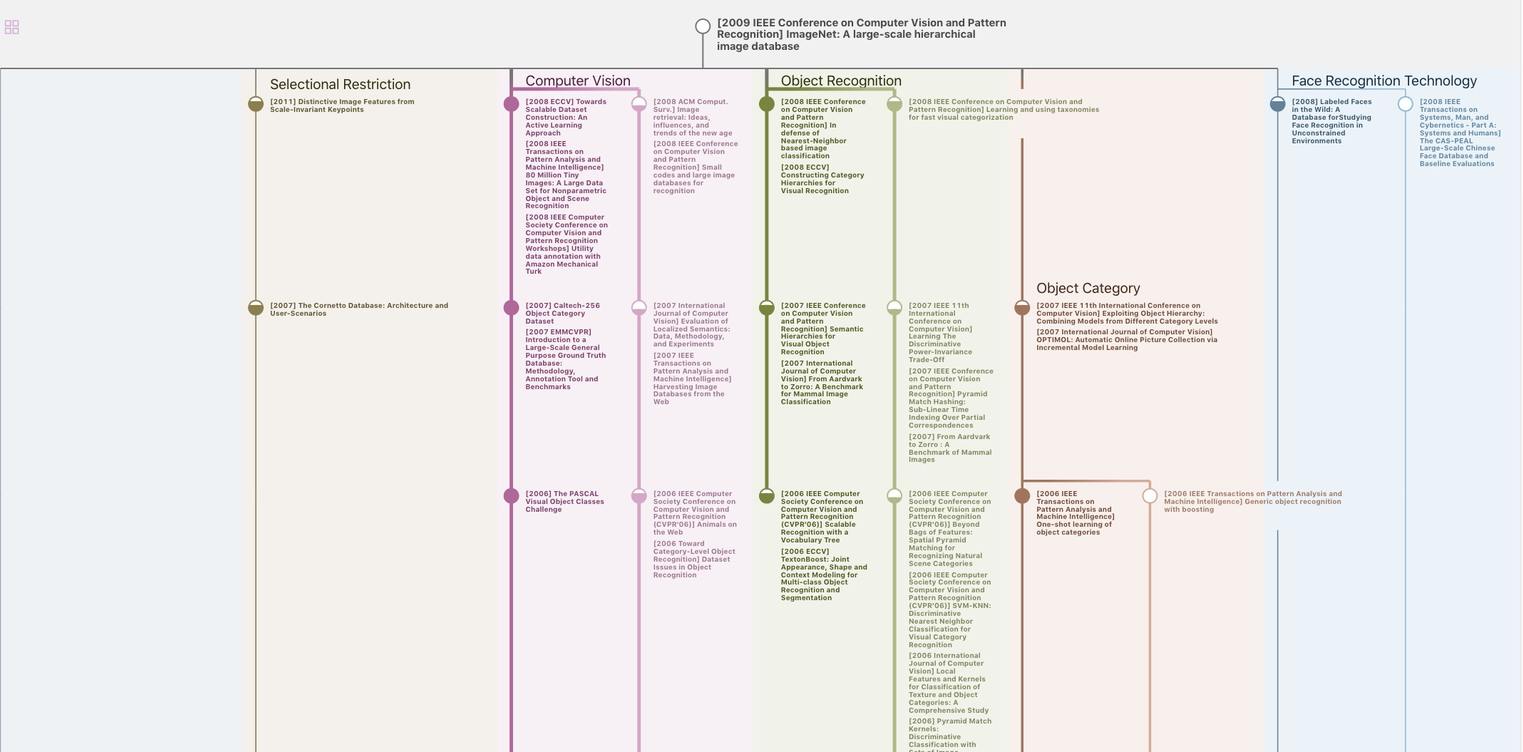
生成溯源树,研究论文发展脉络
Chat Paper
正在生成论文摘要