Machine learning with model selection to predict TOC from mineralogical constituents: case study in the Sichuan Basin
INTERNATIONAL JOURNAL OF ENVIRONMENTAL SCIENCE AND TECHNOLOGY(2022)
摘要
The total organic carbon content from rock samples is the fundamental quantitative and qualitative indicator of the existing organic matter in a reservoir. Generally, it is calculated manually through the analysis of rock samples of origin. However, this procedure demands time and resources since it depends on samples obtained from several intervals of wells in source rocks. Consequently, efforts on research have been conducted to assist this task. Machine learning approaches arise as an alternative to producing estimates for total organic carbon grounded on data well logs and stratigraphic analysis. Given this context, the present paper proposes using machine learning techniques to automate total organic carbon estimation. In order to provide flexibility to the model, a grid search procedure was combined with cross-validation to perform the model selection. This computational approach allows finding models that produced the best generalization capacity. Three methods were applied: Support Vector Machines, Extreme Learning Machine, and Ridge Regression. The proposed methodology was validated on core samples of the shale gas field YuDongNan area, Sichuan Basin. The Support Vector Machine method outperformed the other methods in several metrics analyzed, producing accurate predictions, showing that the approach present in this paper can be used as a surrogate model to assist geologists and petrologists in estimating total organic carbon values.
更多查看译文
关键词
Total organic carbon, Machine learning, Grid search, Support vector machines, Extreme learning machines
AI 理解论文
溯源树
样例
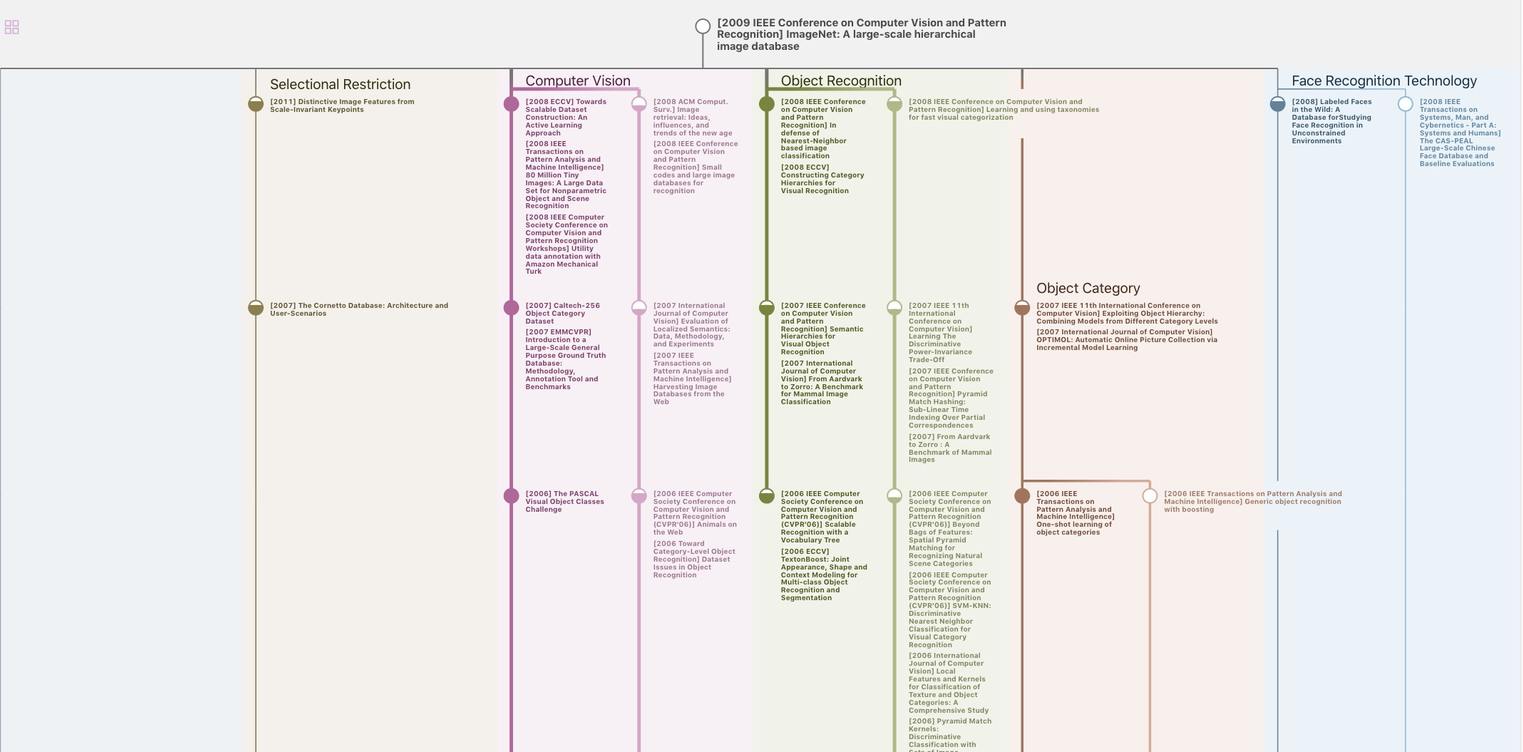
生成溯源树,研究论文发展脉络
Chat Paper
正在生成论文摘要