Prediction intervals for all of M future observations based on linear random effects models
STATISTICA NEERLANDICA(2022)
摘要
In many pharmaceutical and biomedical applications such as assay validation, assessment of historical control data, or the detection of anti-drug antibodies, the calculation and interpretation of prediction intervals (PI) is of interest. The present study provides two novel methods for the calculation of prediction intervals based on linear random effects models and restricted maximum likelihood (REML) estimation. Unlike other REML-based PI found in the literature, both intervals reflect the uncertainty related with the estimation of the prediction variance. The first PI is based on Satterthwaite approximation. For the other PI, a bootstrap calibration approach that we will call quantile-calibration was used. Due to the calibration process this PI can be easily computed for more than one future observation and based on balanced and unbalanced data as well. In order to compare the coverage probabilities of the proposed PI with those of four intervals found in the literature, Monte Carlo simulations were run for two relatively complex random effects models and a broad range of parameter settings. The quantile-calibrated PI was implemented in the statistical software R and is available in the predint package.
更多查看译文
关键词
anti-drug antibody, assay qualification, bootstrap calibration, historical control data, Satterthwaite approximation
AI 理解论文
溯源树
样例
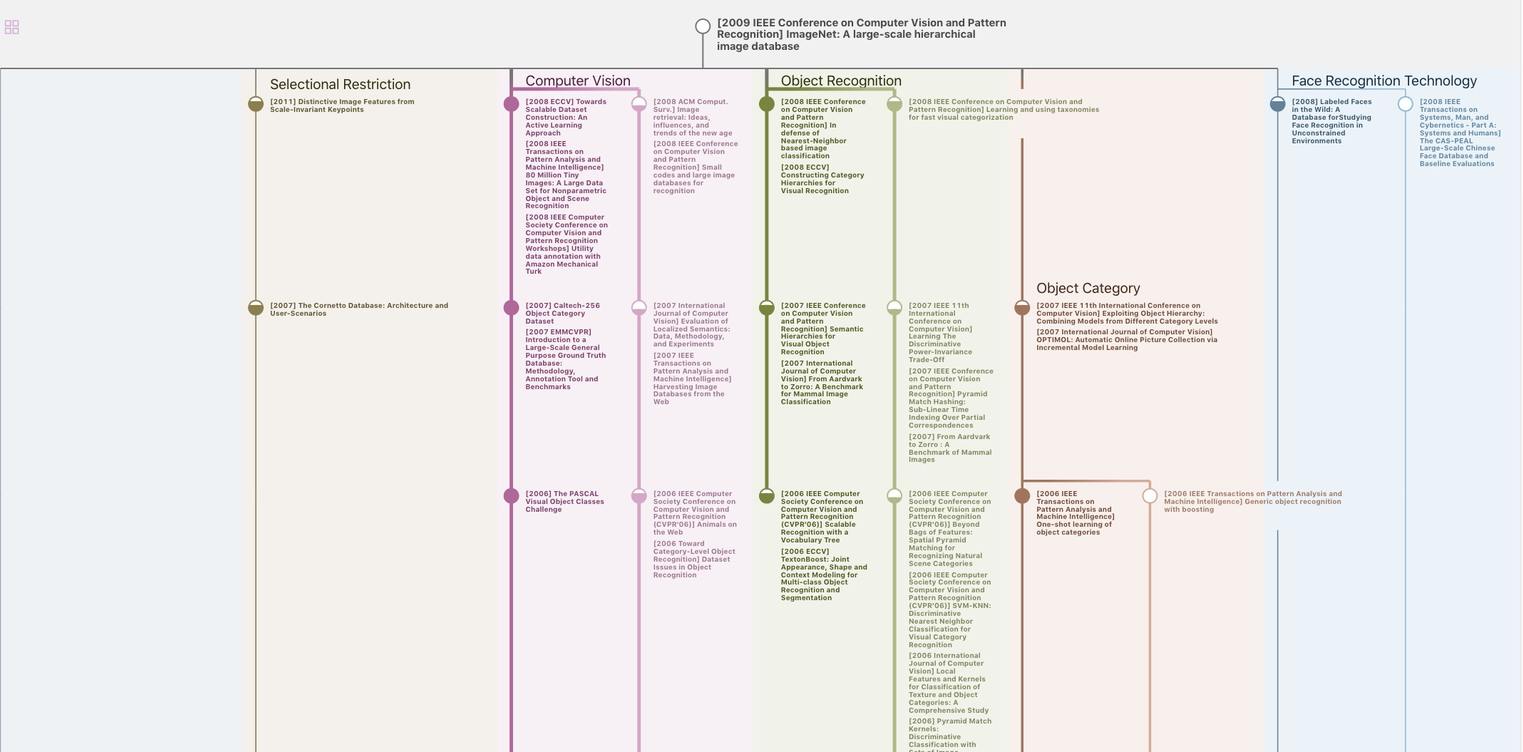
生成溯源树,研究论文发展脉络
Chat Paper
正在生成论文摘要