An Annotation Schema for the Detection of Social Bias in Legal Text Corpora
INFORMATION FOR A BETTER WORLD: SHAPING THE GLOBAL FUTURE, PT I(2022)
摘要
The rapid advancement of artificial intelligence in recent years has led to an increase in its use in legal contexts. At the same time, a growing body of research has expressed concerns that AI trained on large datasets may learn and model undesirable social biases. In this paper, we investigate the extent to which such social biases are inherent in a real-world legal corpus. We train a word2vec word embedding model on case law data and find evidence that NLP methods make undesirable distinctions between legally equivalent entities that vary only by race. Since legal AI applications that model such distinctions risk perpetuating these inequalities when used, we argue that the development of such applications must incorporate a means to detect and mitigate such biases. To this end, we propose an annotation schema that identifies and categorizes deviations from legal equivalence, so that debiasing may be more systematically incorporated into legal AI development. Future directions for research are discussed.
更多查看译文
关键词
Artificial intelligence, Information ethics, Machine learning, Natural language processing
AI 理解论文
溯源树
样例
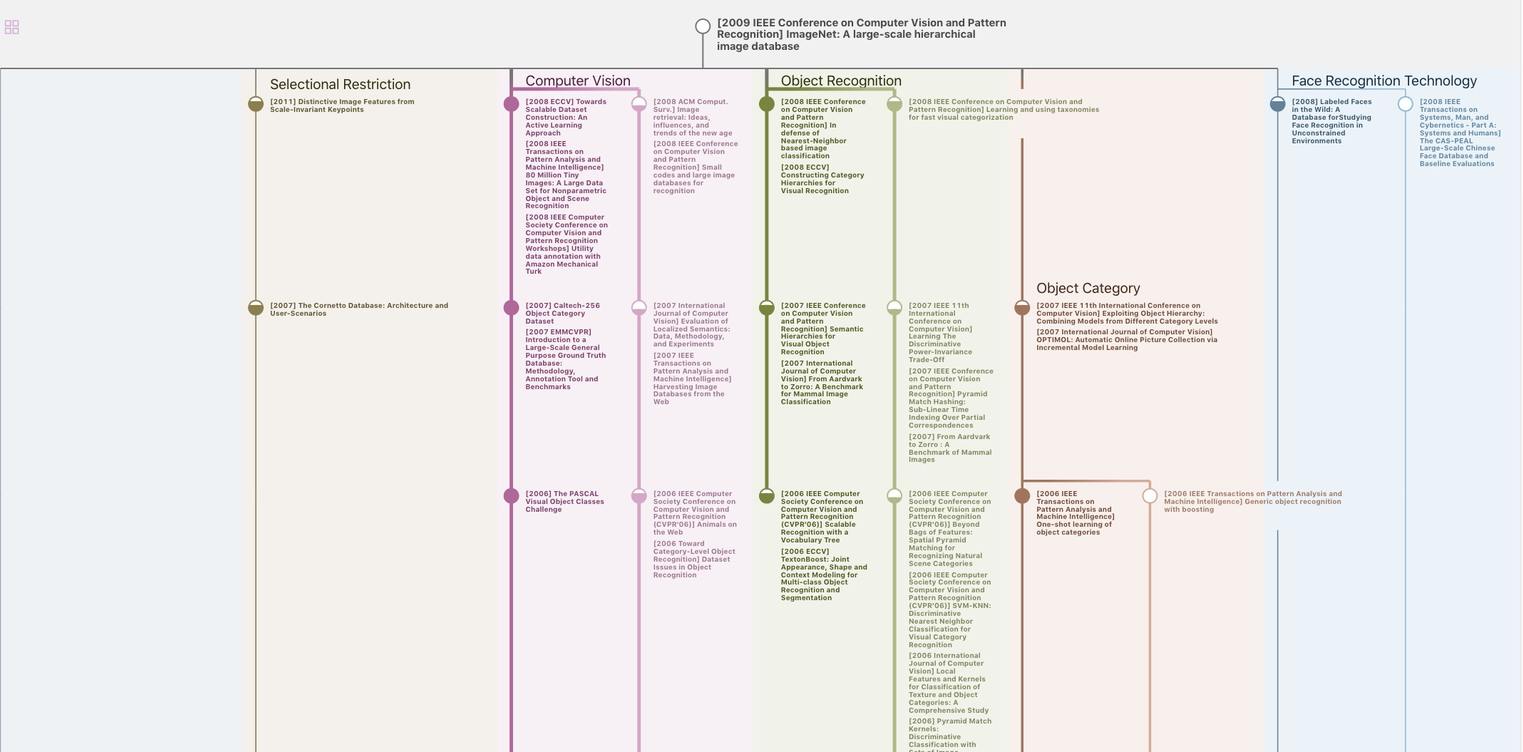
生成溯源树,研究论文发展脉络
Chat Paper
正在生成论文摘要