Unsupervised labelling of remote sensing images based on force field clustering
JOURNAL OF INTELLIGENT & FUZZY SYSTEMS(2022)
摘要
Remote sensing image segmentation provides technical support for decision making in many areas of environmental resource management. But, the quality of the remote sensing images obtained from different channels can vary considerably, and manually labeling a mass amount of image data is too expensive and inefficiently. In this paper, we propose a point density force field clustering (PDFC) process. According to the spectral information from different ground objects, remote sensing superpixel points are divided into core and edge data points. The differences in the densities of core data points are used to form the local peak. The center of the initial cluster can be determined by the weighted density and position of the local peak. An iterative nebular clustering process is used to obtain the result, and a proposed new objective function is used to optimize the model parameters automatically to obtain the global optimal clustering solution. The proposed algorithm can cluster the area of different ground objects in remote sensing images automatically, and these categories are then labeled by humans simply.
更多查看译文
关键词
Remote sensing, core data, nebular clustering, parameter optimization, objective function
AI 理解论文
溯源树
样例
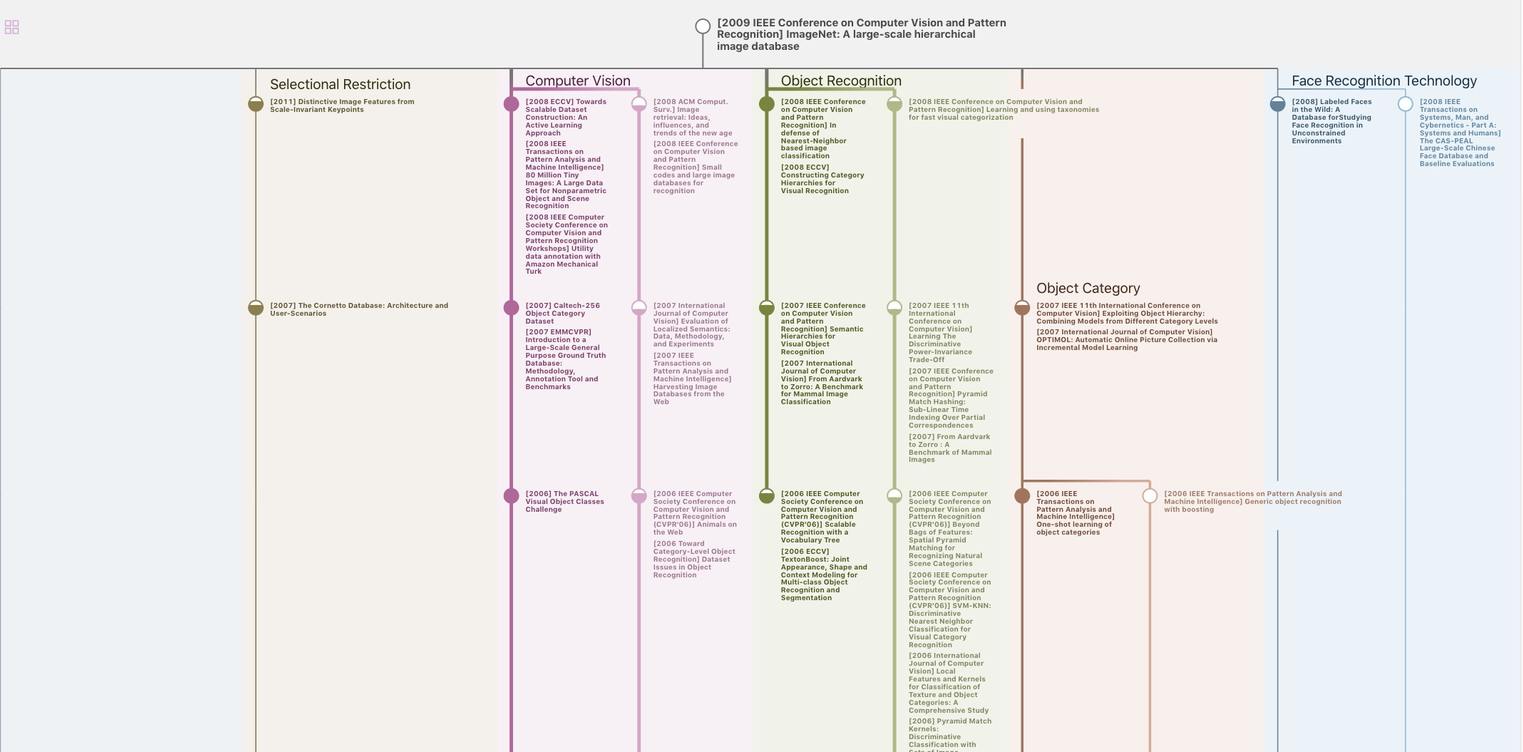
生成溯源树,研究论文发展脉络
Chat Paper
正在生成论文摘要