Efficient Prediction of Court Judgments Using an LSTM plus CNN Neural Network Model with an Optimal Feature Set
MATHEMATICS(2022)
摘要
As the amount of historical data available in the legal arena has grown over time, industry specialists are driven to gather, compile, and analyze this data in order to forecast court case rulings. However, predicting and justifying court rulings while using judicial facts is no easy task. Currently, previous research on forecasting court outcomes using small experimental datasets yielded a number of unanticipated predictions utilizing machine learning (ML) models and conventional methodologies for categorical feature encoding. The current work proposes forecasting court judgments using a hybrid neural network model, namely a long short-term memory (LSTM) network with a CNN, in order to effectively forecast court rulings using historic judicial datasets. By prioritizing and choosing features that scored the highest in the provided legal data set, only the most pertinent features were picked. After that, the LSTM+CNN model was utilized to forecast lawsuit verdicts. In contrast to previous related experiments, this composite model's testing results were promising, showing 92.05 percent accuracy, 93 percent precision, 94 percent recall, and a 93 percent F1-score.
更多查看译文
关键词
court judgment prediction, judicial data, deep learning, neural networks, feature selection
AI 理解论文
溯源树
样例
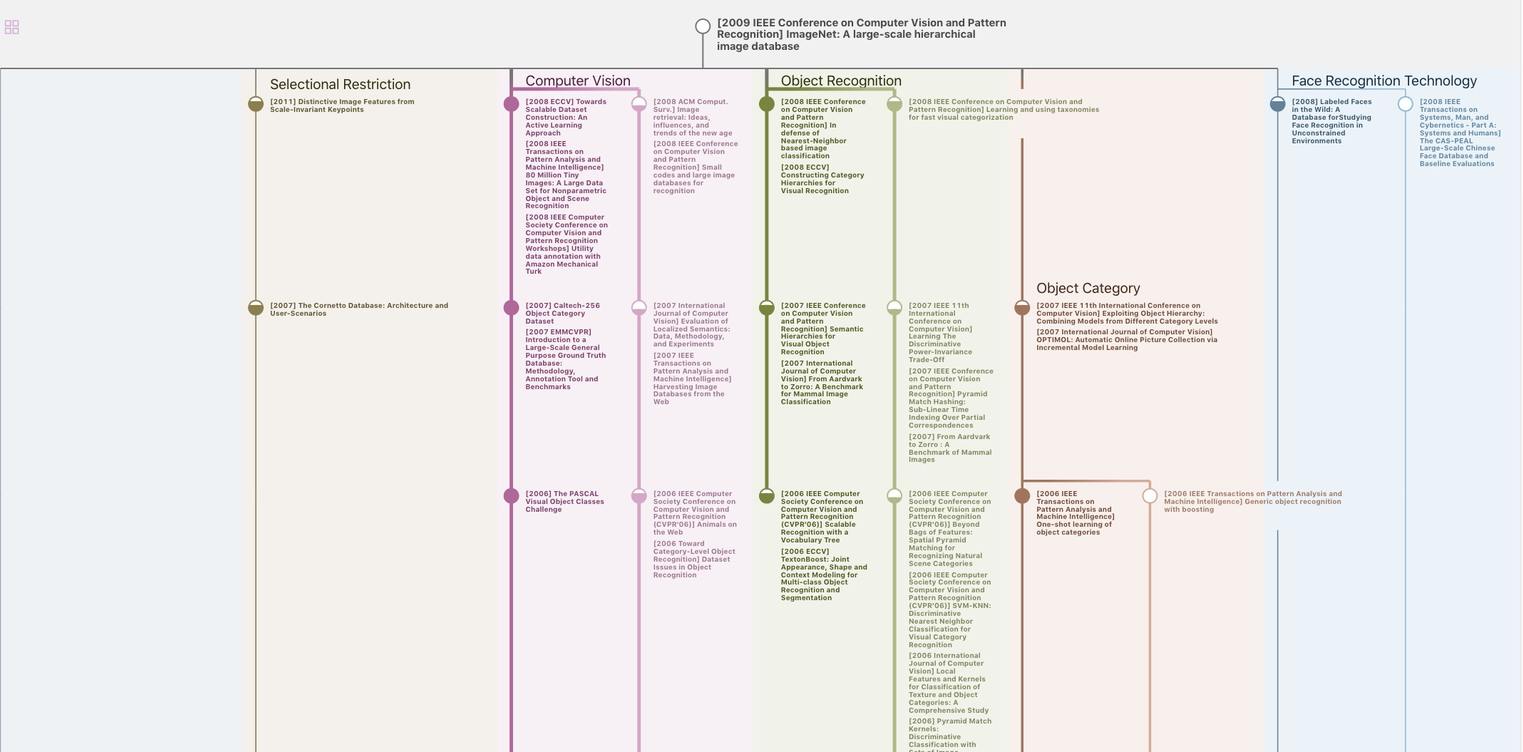
生成溯源树,研究论文发展脉络
Chat Paper
正在生成论文摘要