Neutron/gamma pulse shape discrimination using short-time frequency transform
Analog Integrated Circuits and Signal Processing(2022)
摘要
In this paper, a digital pulse shape discrimination (PSD) method is proposed to distinguish between neutron and gamma events in radiation detectors. Machine learning is employed for classifying the radiation type according to the feature values that represent the pulse shape parameters. To consider both time and frequency parameters of the pulse signal, the short-time frequency transform is employed for the feature extraction process. The proposed approach utilizes the principal component analysis, for dimensionality reduction, where only the most significant components are selected for the classification procedure. The logistic regression model is chosen, among many classifiers, to provide a discrimination parameter through which performance comparison with conventional PSD techniques can be easily applied. To evaluate the performance of the proposed algorithm, the figure of merit and the classification accuracy are calculated at different signal to noise ratios and energy levels. The effectiveness of the proposed approach is validated through a performance comparison with state-of-the art methods and traditional PSD techniques, like charge comparison, pulse gradient analysis, and frequency gradient analysis. Experimental results show that the proposed technique introduces better discrimination capability than that of the other methods.
更多查看译文
关键词
Digital pulse shape discrimination, Short-time frequency transform, Principal component analysis, Logistic regression
AI 理解论文
溯源树
样例
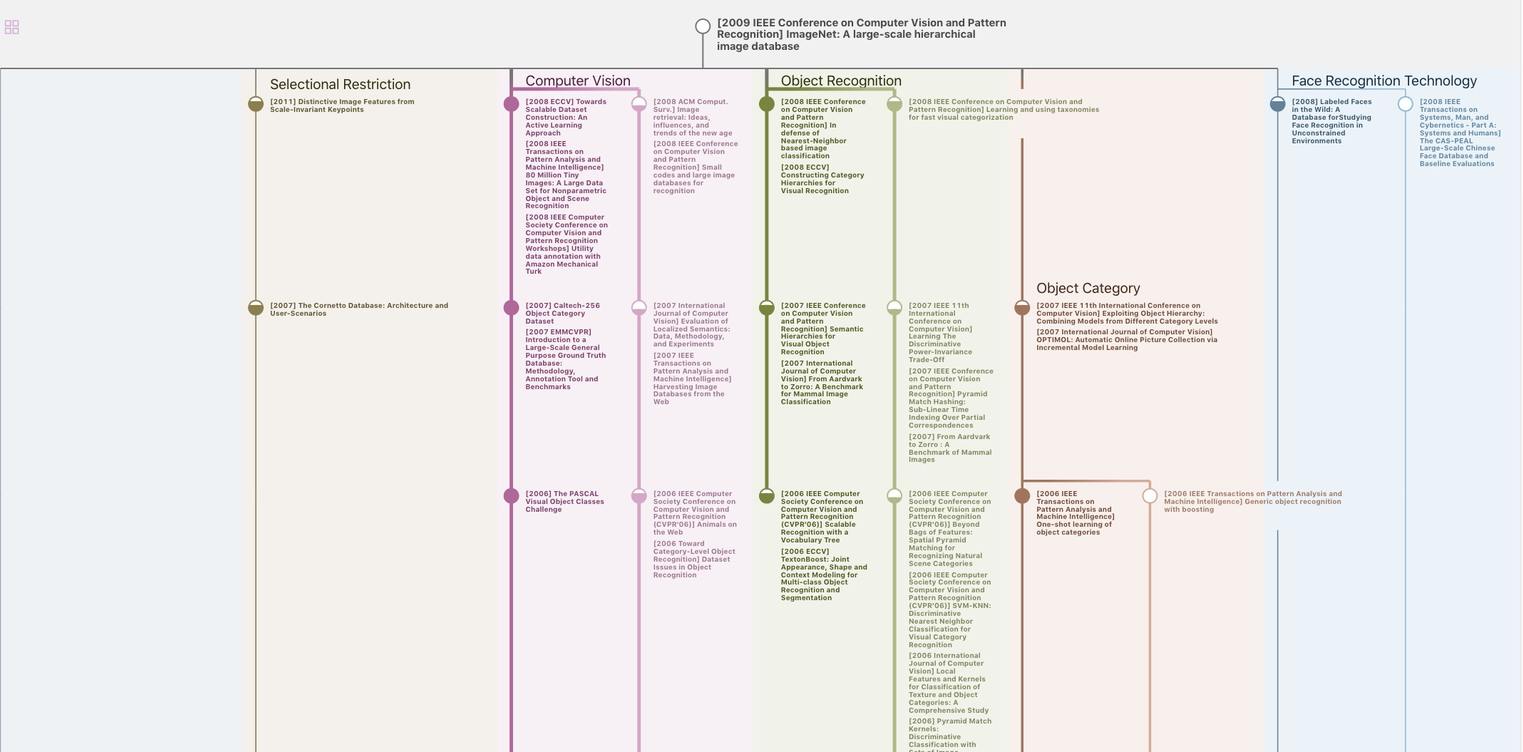
生成溯源树,研究论文发展脉络
Chat Paper
正在生成论文摘要