Quality detection and classification for ultrasonic welding of carbon fiber composites using time-series data and neural network methods
JOURNAL OF MANUFACTURING SYSTEMS(2021)
摘要
Ultrasonic welding is a novel and efficient technique for joining carbon fiber composites in the automotive in-dustry. Weld quality detection and classification is important to its adoption and deep neural network models are a promising method for this purpose. However, it is difficult to collect the large volume of data needed to train these models with laboratory experiments due to the cost of the materials and cost of weld experiments. Using a limited set of experimental data, a copula multivariate Monte Carlo simulation is proposed to generate large data sets of time-series process signals with similar statistical distributions as the experimental data. The experimental data and simulated data are used to train Bayesian regularized neural network (BRNN) and convolutional neural network (CNN) models to predict weld quality classifications in ultrasonic welding. The results show that BRNN and CNN have similar classification accuracy. But CNN has an advantage in training efficiency compared with BRNN. Both neural-network-based methods were found to be more accurate than support vector machine and k-nearest neighbor methods, when applied to both features extracted from signals and full time-series-based process signals.
更多查看译文
关键词
Ultrasonic welding, Carbon fiber reinforced polymers, Time-series process signals, Multivariate Monte Carlo simulation, Weld quality classification, Bayesian regularized neural network, Convolutional neural network
AI 理解论文
溯源树
样例
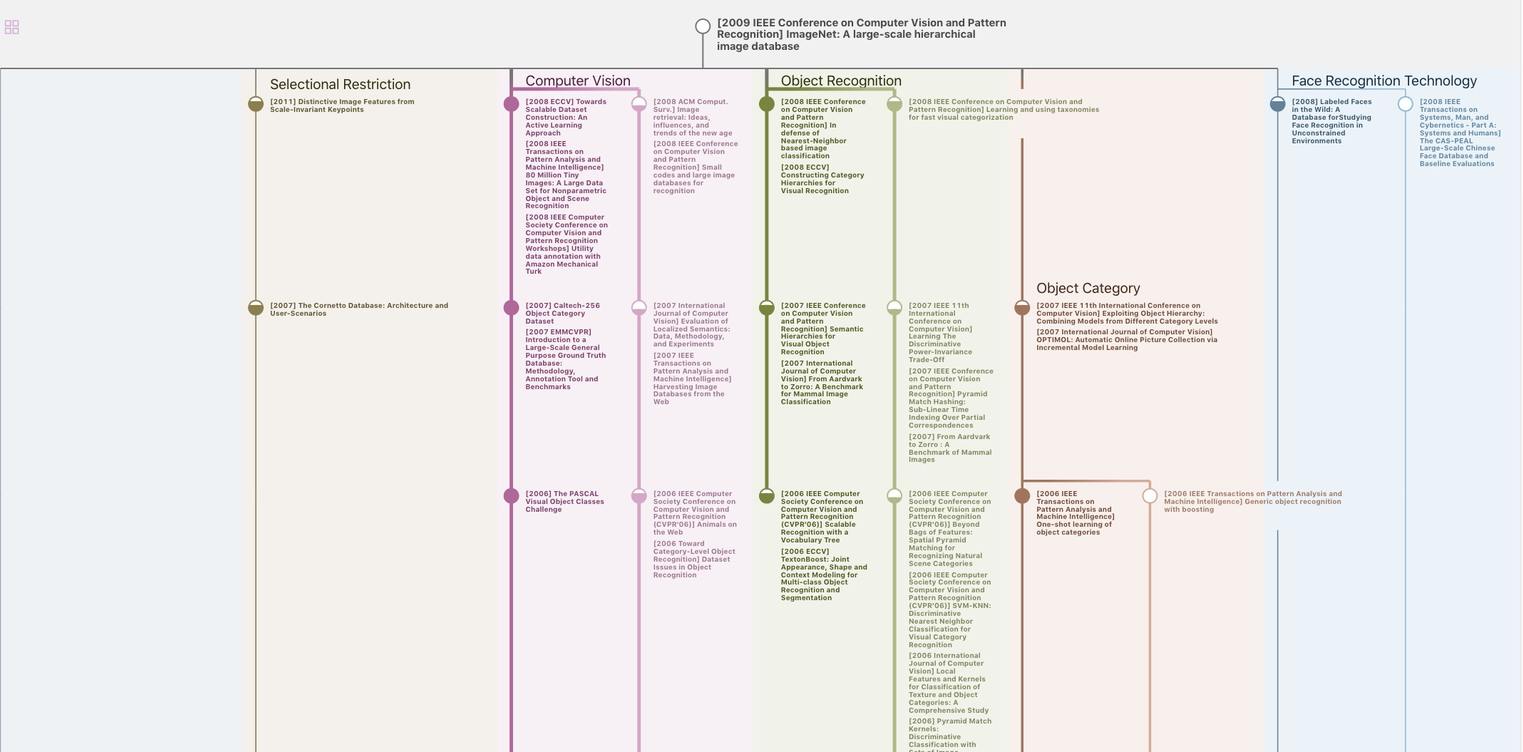
生成溯源树,研究论文发展脉络
Chat Paper
正在生成论文摘要