Novel Biomarkers and Machine Learning Models for the Prediction of 30-Day Readmission Following Pediatric Congenital Heart Surgery
CIRCULATION(2021)
摘要
Introduction: Hospital readmissions after congenital heart surgery (CHS) are common in pediatric patients and are linked to higher risks of complications, mortality, and increased treatment costs. Accurate prediction of 30-day readmission after CHS could help clinicians identify high risk patients and enable tailored care. Hypothesis: Novel biomarkers alone enhance prediction of readmission risk using XGBoost model and augmenting clinical features to the Society of Thoracic Surgeons (STS)-risk model improves predictions of 30-day readmissions. Methods: The prospective cohort included children of 18 years or younger who underwent congenital heart surgery at Johns Hopkins Hospital. Of the 162 patients, 159 survived discharge and were therefore included in the study. We used pre- and post-operative biomarkers ST2, Galectin-3, NT-proBNP and GFAP as features for the biomarker model, covariates from the STS CHS Database Mortality Risk Model for the STS-risk model and augmenting clinical features to STS-risk variables for clinical model. We implemented the XGBoost model and compared the biomarker model and clinical model performance to the STS-risk model. Results: Readmissions within 30 days of surgery occurred among 9% of pediatric patients. Using XGBoost, the STS-risk model derived an AUROC of 0.978 (95% CI: 0.955-1.0). The biomarker model performed better than the STS-risk model with AUROC of 0.982 (95% CI: 0.959-1.0, p=0.8). The augmented clinical model outperformed the STS-risk model with an AUROC of 0.997 (95% CI: 0.99-1, p=0.1). Conclusions: Novel biomarkers alone perform better compared to the STS- risk model in predicting 30-day readmission risk. XGBoost model improves risk prediction when adding additional clinical features to the existing STS-risk model which outperformed the STS-risk model. Depending on healthcare systems clinical resources and data availability, an optimized XGBoost model can improve the readmission risk prediction outcome.
更多查看译文
AI 理解论文
溯源树
样例
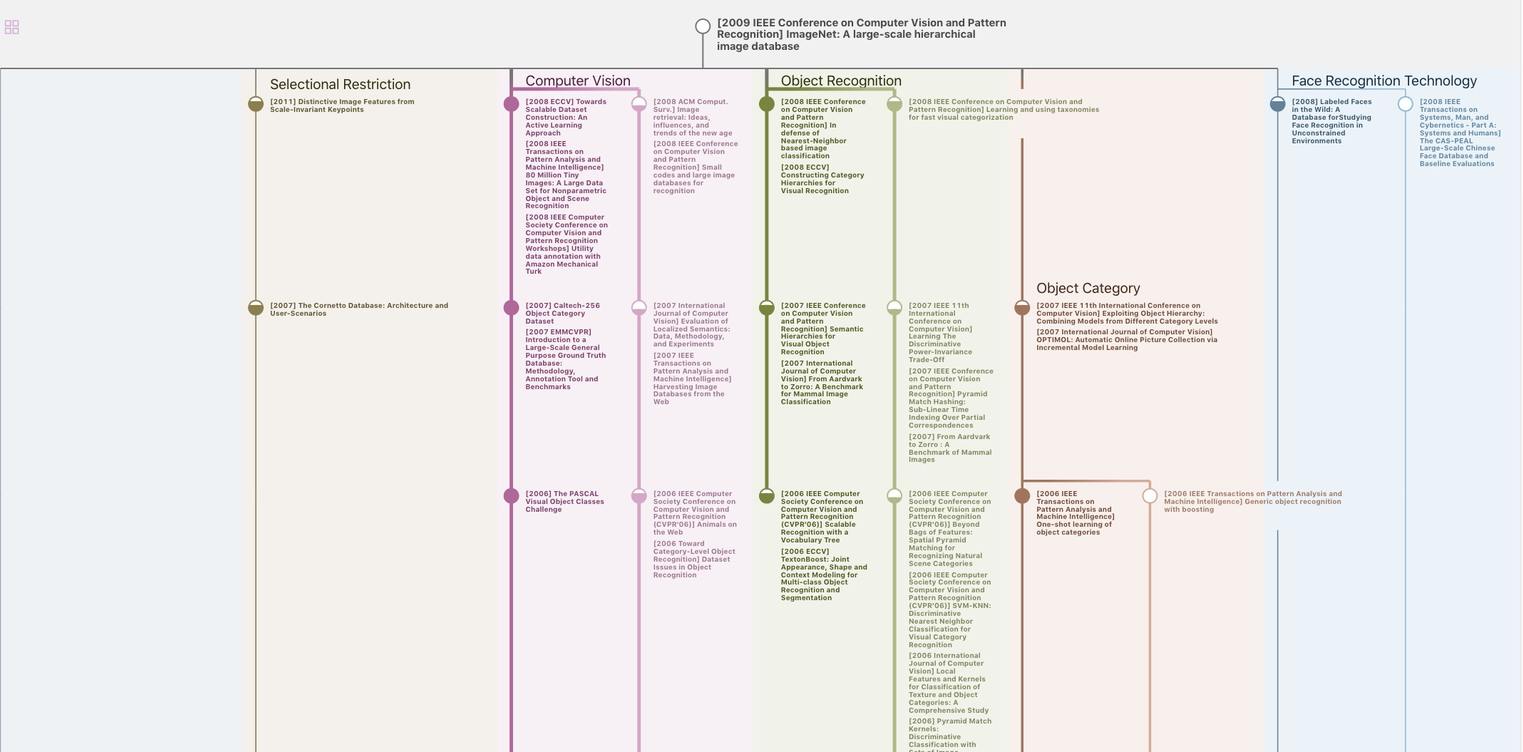
生成溯源树,研究论文发展脉络
Chat Paper
正在生成论文摘要