Automated training of location-specific edge models for traffic counting
COMPUTERS & ELECTRICAL ENGINEERING(2022)
摘要
Deep neural networks are the state of the art for various machine learning problems dealing with large amounts of rich sensor data. It is often desirable to evaluate these models on edge devices instead of relying on cloud computing. In this paper, we perform traffic counting using surveillance cameras. Edge computing is required as only aggregated counts should leave the device and not the privacy sensitive video frames. Unfortunately, only small object detection models are suited for edge devices which results in sub-optimal performance. We introduce location specific models that are each trained for one specific camera. The model does not need to generalize to other locations. We show that smaller specialized models can outperform large general purpose models. We propose an automated way to train these small models without human intervention. We experimentally show that we can achieve a similar counting accuracy with 5x fewer parameters than state-of-the-art techniques.
更多查看译文
关键词
Deep learning, Edge computing, Traffic counting, Knowledge transfer, Distributed machine learning
AI 理解论文
溯源树
样例
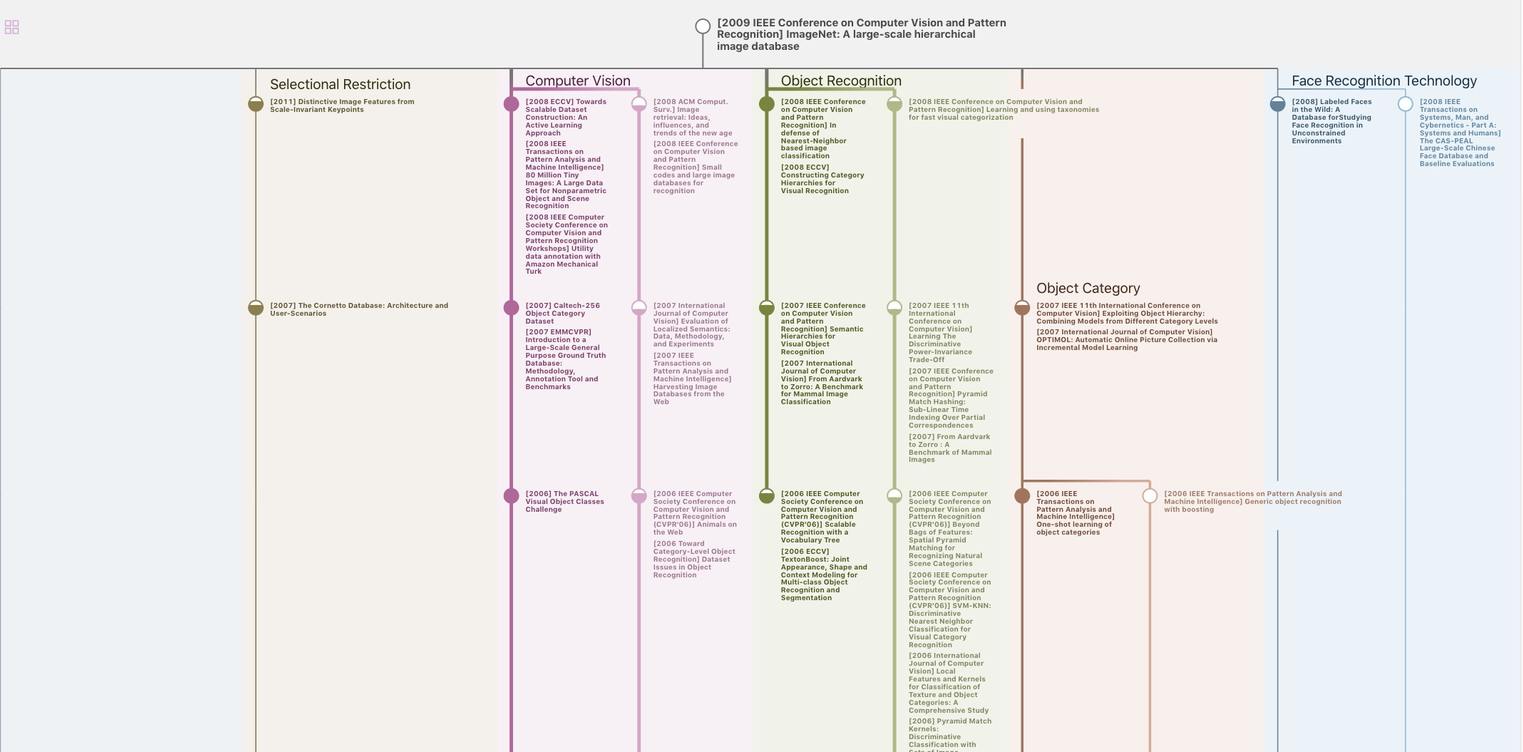
生成溯源树,研究论文发展脉络
Chat Paper
正在生成论文摘要