MSM: A Method of Multi-Neighborhood Sampling Matching for Entity Alignment
INTELLIGENT AUTOMATION AND SOFT COMPUTING(2022)
摘要
The heterogeneity of knowledge graphs brings great challenges to entity alignment. In particular, the attributes of network entities in the real world are complex and changeable. The key to solving this problem is to expand the neighborhoods in different ranges and extract the neighborhood information efficiently. Based on this idea, we propose Multi-neighborhood Sampling Matching Network (MSM), a new KG alignment network, aiming at the structural heterogeneity challenge. MSM constructs a multi-neighborhood network representation learning method to learn the KG structure embedding. It then adopts a unique sampling and cosine cross-matching method to solve different sizes of neighborhoods and distinct topological structures in two entities. To choose the right neighbors, we apply a down-sampling process to select the most informative entities towards the central target entity from its one-hop and two-hop neighbors. To verify the effectiveness of matching this neighborhood with any neighborhood in the corresponding node, we give a cosine cross-graph neighborhood matching method and conduct detailed research and analysis on three entity matching data sets, which proves the effectiveness of MSM.
更多查看译文
关键词
Entity alignment, representation learning, heterogeneous network
AI 理解论文
溯源树
样例
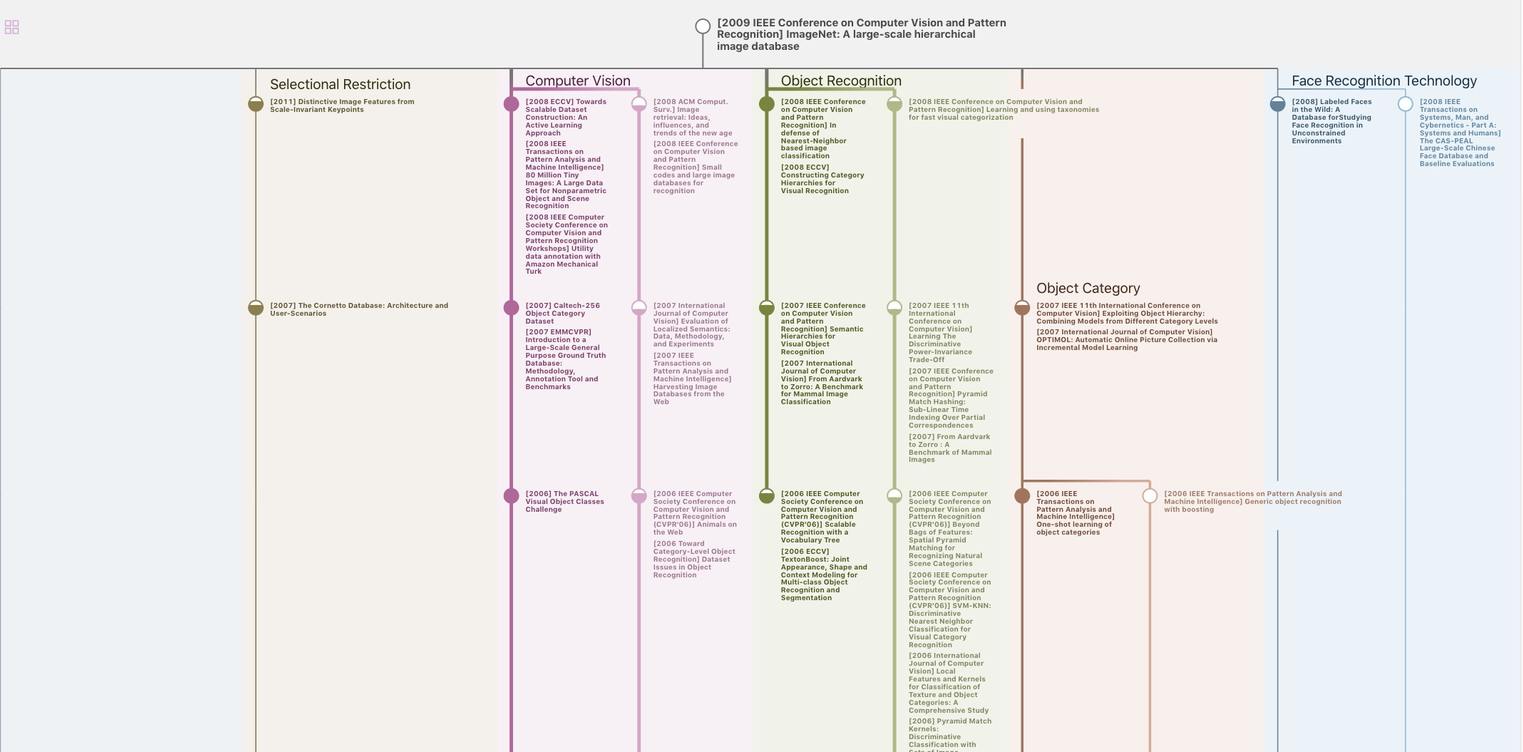
生成溯源树,研究论文发展脉络
Chat Paper
正在生成论文摘要