Efficient image segmentation based on deep learning for mineral image classification
ADVANCED POWDER TECHNOLOGY(2021)
摘要
Mineral image segmentation plays a vital role in the realization of machine vision based intelligent ore sorting equipment. However, the existing image segmentation methods still cannot effectively solve the problem of adhesion and overlap between mineral particles, and the segmentation performance of small and irregular particles still needs to be improved. To overcome these bottlenecks, we propose a deep learning based image segmentation method to segment the key areas in mineral images using morphological transformation to process mineral image masks. This investigation explores four aspects of the deep learning-based mineral image segmentation model, including backbone selection, module configuration, loss function construction, and its application in mineral image classification. Specifically, referring to the designs of U-Net, FCN, Seg Net, PSP Net, and DeepLab Net, this experiment uses different backbones as Encoder to building ten mineral image segmentation models with different layers, structures, and sampling methods. Simultaneously, we propose a new loss function suitable for mineral image segmentation and compare CNNs-based segmentation models' training performance under different loss functions. The experiment results show that the proposed mineral image segmentation has excellent segmentation performance, effectively solves adhesion and overlap between adjacent particles without affecting the classification accuracy. By using the Mobile Net as backbone, the PSP Net and DeepLab can achieve a high segmentation performance in mineral image segmentation tasks, and the 15 x 15 is the most suitable size for erosion element structure to process the mask images of the segmentation models. (c) 2021 The Society of Powder Technology Japan. Published by Elsevier B.V. and The Society of Powder Technology Japan. All rights reserved.
更多查看译文
关键词
Deep learning, Convolutional neural networks, Mineral image segmentation
AI 理解论文
溯源树
样例
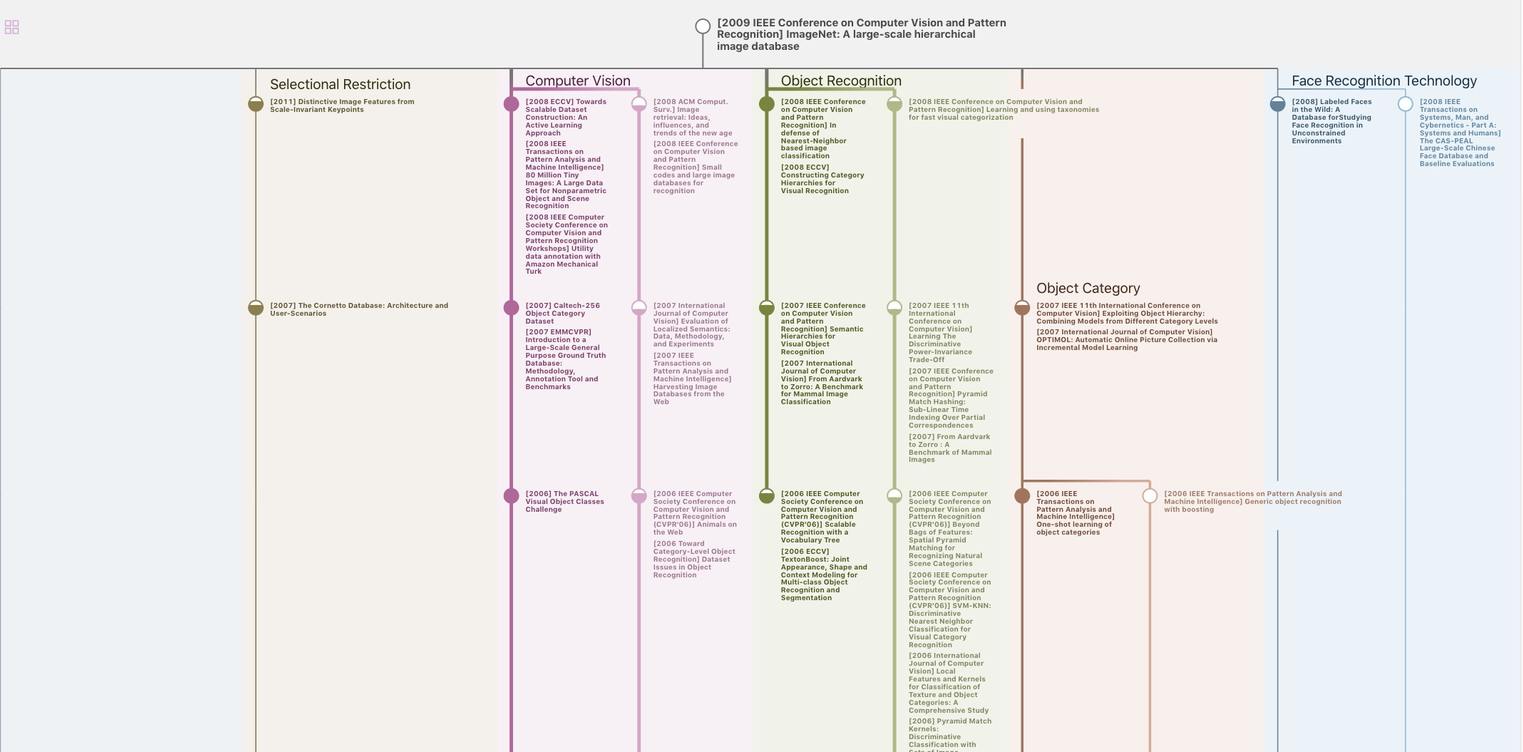
生成溯源树,研究论文发展脉络
Chat Paper
正在生成论文摘要