Small Traffic Sign Detection in Big Images: Searching Needle in a Hay
IEEE ACCESS(2022)
摘要
Traffic sign detection is an essential module of self-driving cars and driver assistance system. The major challenge being, traffic sign appear relatively smaller in road view images. It covers only 1%-2% of the total image area. Hence, its challenging to detect very small traffic sign in a larger image covering huge background of similar shape objects. Thus, we propose YOLOv3 network layers pruning and patch wise training strategy for small sized traffic sign detection. This aids in improving recall percentage and mean Average Precision. We also propose anchor box selection algorithm that uses bounding box dimension density to obtain optimal anchor set for the dataset. This reduces false positives and log-average miss rate. The proposed approach is evaluated on German traffic sign detection benchmark and Swedish traffic sign dataset and proves that it achieved a good balance between mAP and inference time.
更多查看译文
关键词
Detectors, Feature extraction, Roads, Convolutional neural networks, Proposals, Benchmark testing, Reliability, Anchor box algorithm, network pruning, small object detection, YOLOv3
AI 理解论文
溯源树
样例
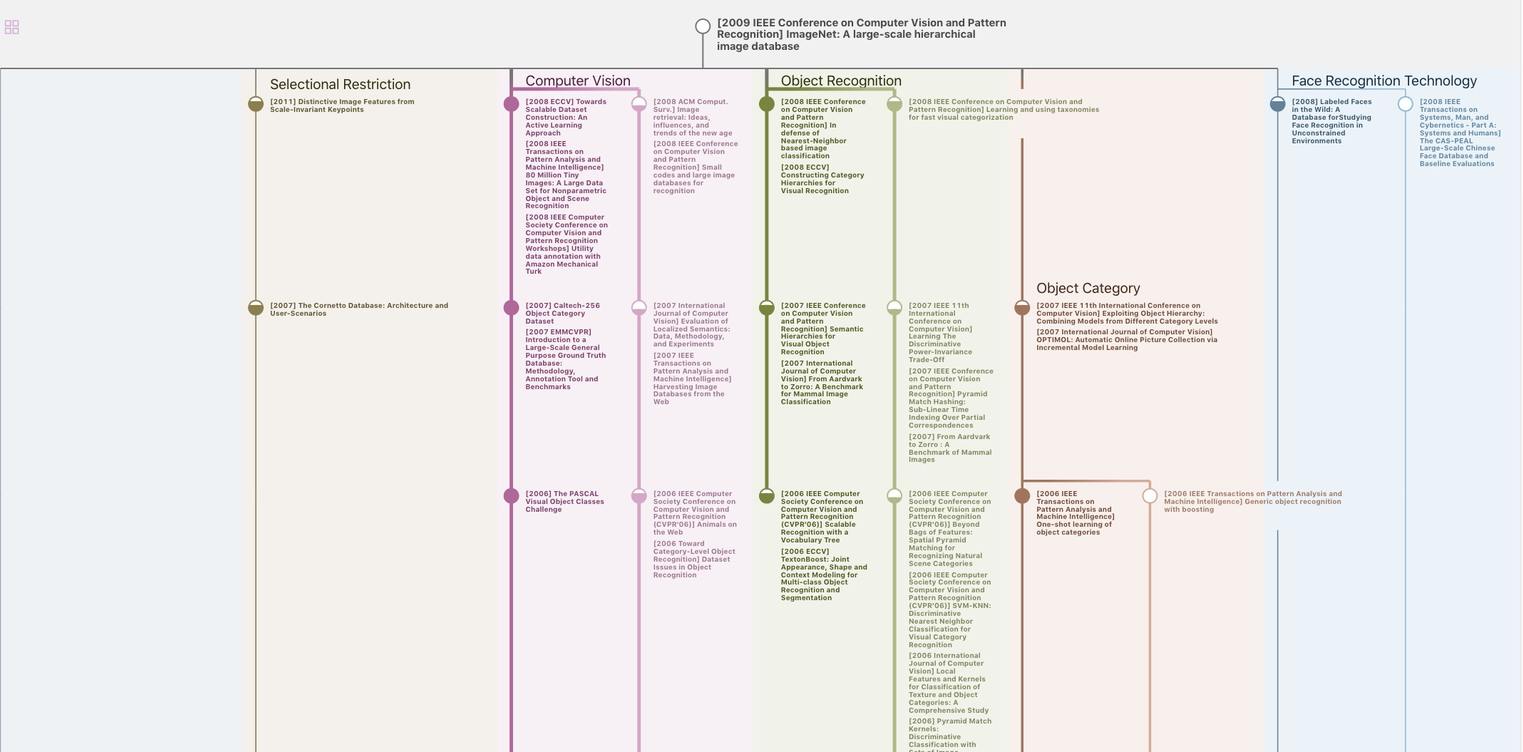
生成溯源树,研究论文发展脉络
Chat Paper
正在生成论文摘要