Predictive Modeling of Diabetes using EMR Data
HEALTHINF: PROCEEDINGS OF THE 15TH INTERNATIONAL JOINT CONFERENCE ON BIOMEDICAL ENGINEERING SYSTEMS AND TECHNOLOGIES - VOL 5: HEALTHINF(2021)
摘要
As the prevalence of diabetes continues to increase globally, an efficient diabetes prediction model based on Electronic Medical Records (EMR) is critical to ensure the well-being of the patients and reduce the burden on the healthcare system. Prediction of diabetes in patients at an early stage and analysis of the risk factors can enable diabetes primary and secondary prevention. The objective of this study is to explore various classification models for identifying diabetes using EMR data. We extracted patient information, disease, health conditions, billing, and medication from EMR data. Six machine learning algorithms including three ensemble and three non-ensemble classifiers were used namely XGBoost, Random Forest, AdaBoost, Logistic Regression, Naive Bayes, and K-Nearest Neighbor (KNN). We experimented with both imbalanced data with the original class distribution and artificially balanced data for training the models. Our results indicate that the Random Forest model overall outperformed other models. When applied to the imbalanced data (112,837 instances), it results in the highest values in specificity (0.99) and F1-score (0.84), and when training with balanced data (35,858 instances) it achieves better values in sensitivity (1.00) and AUC (0.96). Analyzing feature importance, we identified a set of features that are more impactful in deciding the outcome including a number of comorbid conditions such as hypertension, dyslipidemia, osteoarthritis, CKD. and depression as well as a number of medication codes such as A10, D08, C10, and C09.
更多查看译文
关键词
Machine Learning, EMR Data, Diabetes, Ensemble Models, Classification Algorithms, Imbalanced Data
AI 理解论文
溯源树
样例
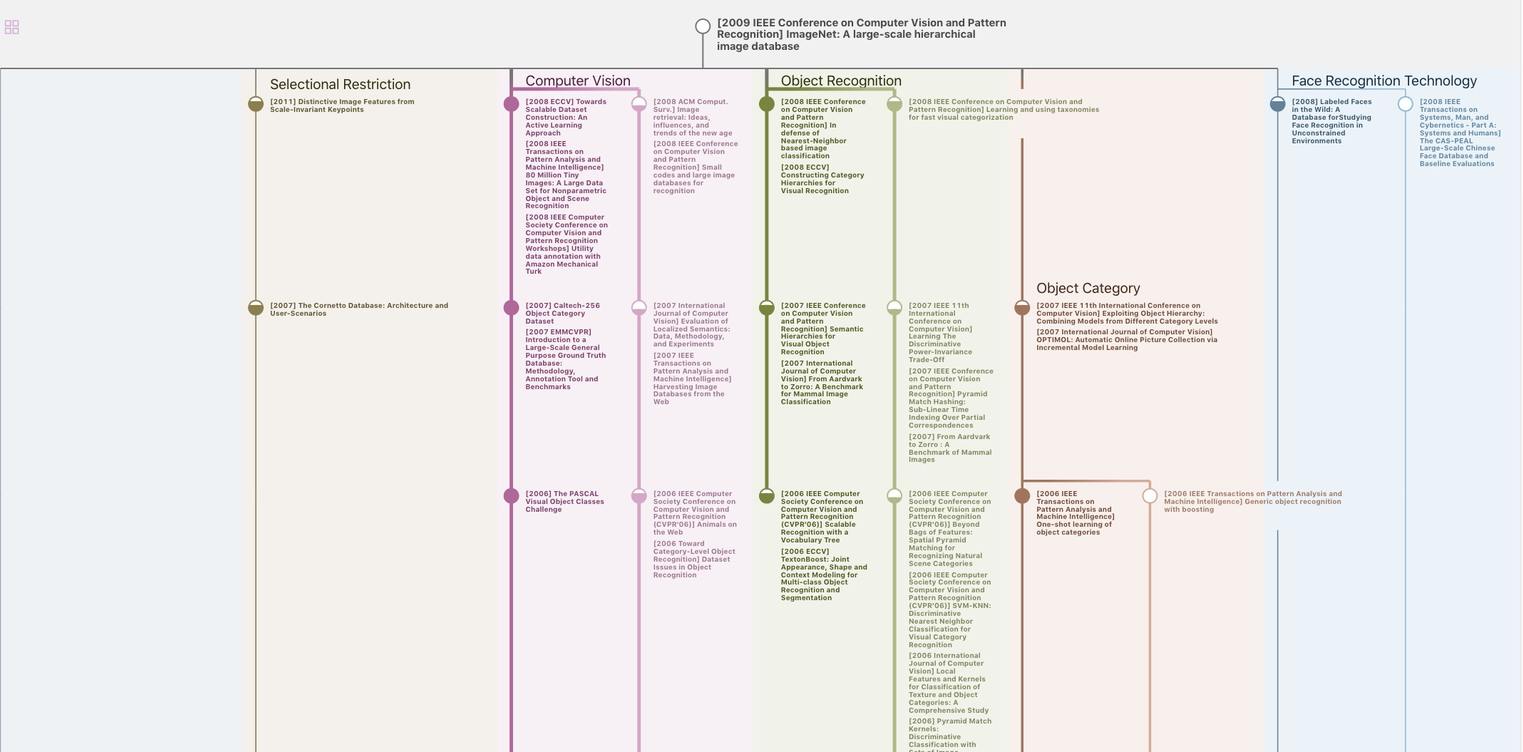
生成溯源树,研究论文发展脉络
Chat Paper
正在生成论文摘要