A Novel Spatial-Temporal Multi-Scale Alignment Graph Neural Network Security Model for Vehicles Prediction
IEEE TRANSACTIONS ON INTELLIGENT TRANSPORTATION SYSTEMS(2023)
摘要
Traffic flow forecasting is indispensable in today's society and regarded as a key problem for Intelligent Transportation Systems (ITS), as emergency delays in vehicles can cause serious traffic security accidents. However, the complex dynamic spatial-temporal dependency and correlation between different locations on the road make it a challenging task for security in transportation. To date, most existing forecasting frames make use of graph convolution to model the dynamic spatial-temporal correlation of vehicle transportation data, ignoring semantic similarity between nodes and thus, resulting in accuracy degradation. In addition, traffic data does not strictly follow periodicity and hard to be captured. To solve the aforementioned challenging issues, we propose in this article CRFAST-GCN, a multi-branch spatial-temporal attention graph convolution network. First, we capture the multi-scale (e.g., hour, day, and week) long- short-term dependencies through three identical branches, then introduce conditional random field (CRF) enhanced graph convolution network to capture the semantic similarity globally, so then we exploit the attention mechanism to captures the periodicity. For model evaluation using two real-world datasets, performance analysis shows that the proposed CRFAST-GCN successfully handles the complex spatial-temporal dynamics effectively and achieves improvement over the baselines at 50% (maximum), outperforming other advanced existing methods.
更多查看译文
关键词
Convolution, Correlation, Vehicle dynamics, Forecasting, Roads, Predictive models, Time series analysis, Conditional random field, graph conventional network, smart city, security frame, vehicle prediction
AI 理解论文
溯源树
样例
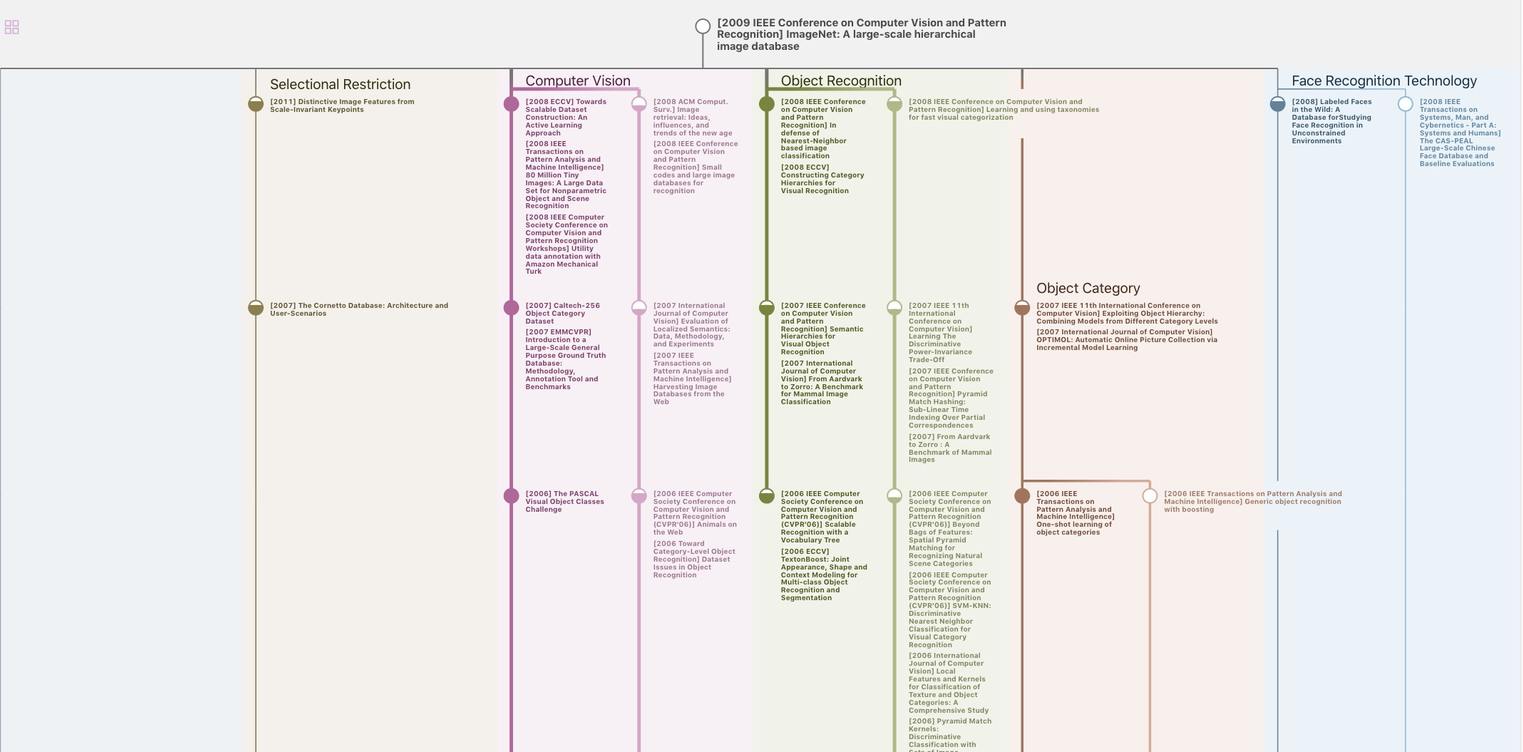
生成溯源树,研究论文发展脉络
Chat Paper
正在生成论文摘要