Simplicial and Minimal-Variance Distances in Multivariate Data Analysis
JOURNAL OF STATISTICAL THEORY AND PRACTICE(2022)
摘要
In this paper, we study the behaviour of the so-called k -simplicial distances and k -minimal-variance distances between a point and a sample. The family of k -simplicial distances includes the Euclidean distance, the Mahalanobis distance, Oja’s simplex distance and many others. We give recommendations about the choice of parameters used to calculate the distances, including the size of the sub-sample of simplices used to improve computation time, if needed. We introduce a new family of distances which we call k -minimal-variance distances. Each of these distances is constructed using polynomials in the sample covariance matrix, with the aim of providing an alternative to the inverse covariance matrix, that is applicable when data is degenerate. We explore some applications of the considered distances, including outlier detection and clustering, and compare how the behaviour of the distances is affected for different parameter choices.
更多查看译文
关键词
Mahalanobis distance,Scatter,Generalized inverse,Simplices
AI 理解论文
溯源树
样例
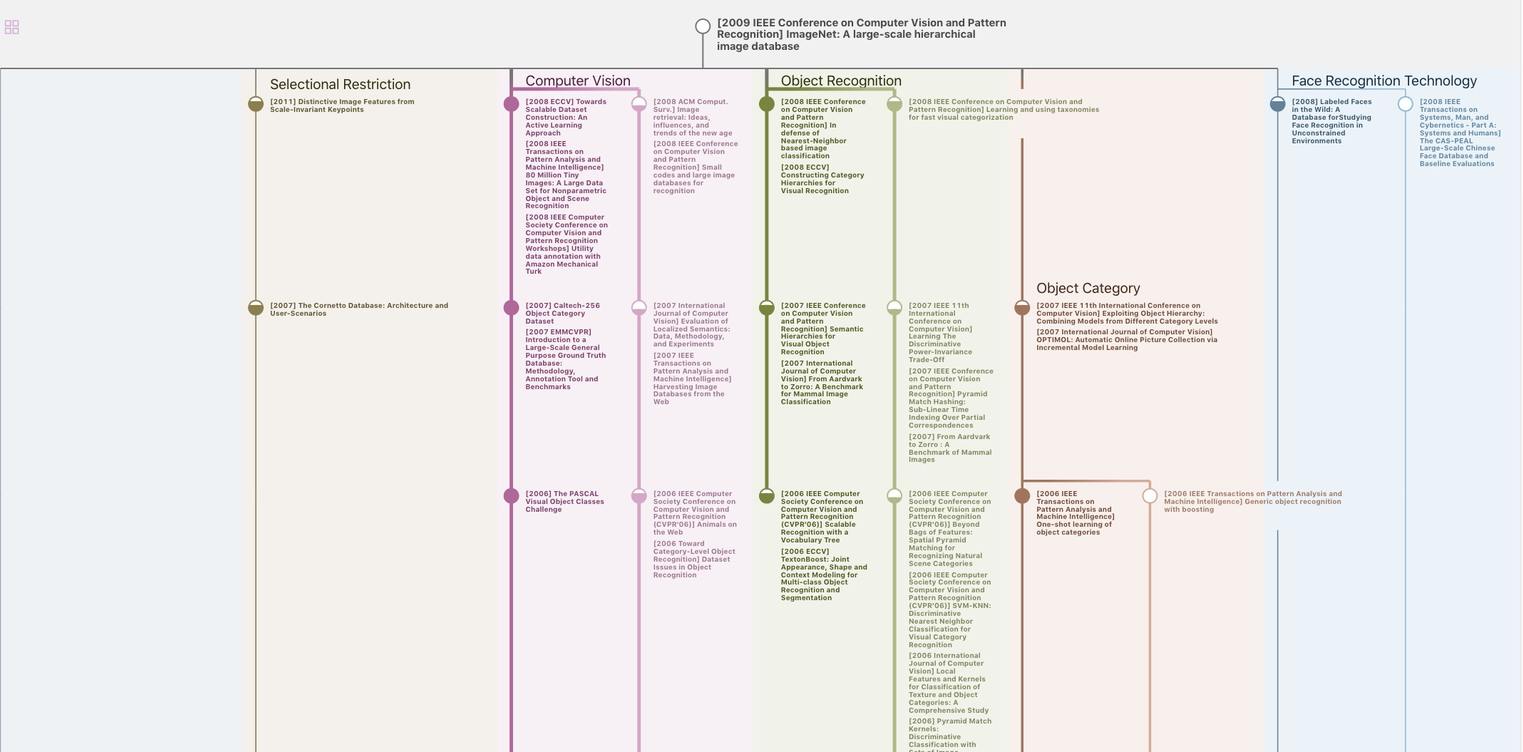
生成溯源树,研究论文发展脉络
Chat Paper
正在生成论文摘要