CLASSIFICATION MODEL FOR BREAST CANCER MAMMOGRAMS
IIUM ENGINEERING JOURNAL(2022)
摘要
Machine learning has been the topic of interest in research related to early detection of breast cancer based on mammogram images. In this study, we compare the performance results from three (3) types of machine learning techniques: 1) Naive Bayes (NB), 2) Neural Network (NN) and 3) Support Vector Machine (SVM) with 2000 digital mammogram images to choose the best technique that could model the relationship between the features extracted and the state of the breast (Normal' or 'Cancer). Grey Level Co-occurrence Matrix (GLCM) which represents the two dimensions of the level variation gray in the image is used in the feature extraction process. Six (6) attributes consist of contrast, variance, standard deviation, kurtosis, mean and smoothness were computed as feature extracted and used as the inputs for the classification process. The data has been randomized and the experiment has been repeated for ten (10) times to check for the consistencies of the performance of all techniques. 70% of the data were used as the training data and another 30% used as testing data. The result after ten (10) experiments show that, Support Vector Machine (SVM) gives the most consistent results in correctly classifying the state of the breast as 'Normal' or 'Cancer', with the accuracy of 99.4%, in training and 98.76% in testing. The SVM classification model has outperformed NN and NB model in the study, and it shows that SVM is a good choice for determining the state of the breast at the early stage.
更多查看译文
关键词
machine learning, breast cancer detection, mammogram images, data mining, data-driven modelling
AI 理解论文
溯源树
样例
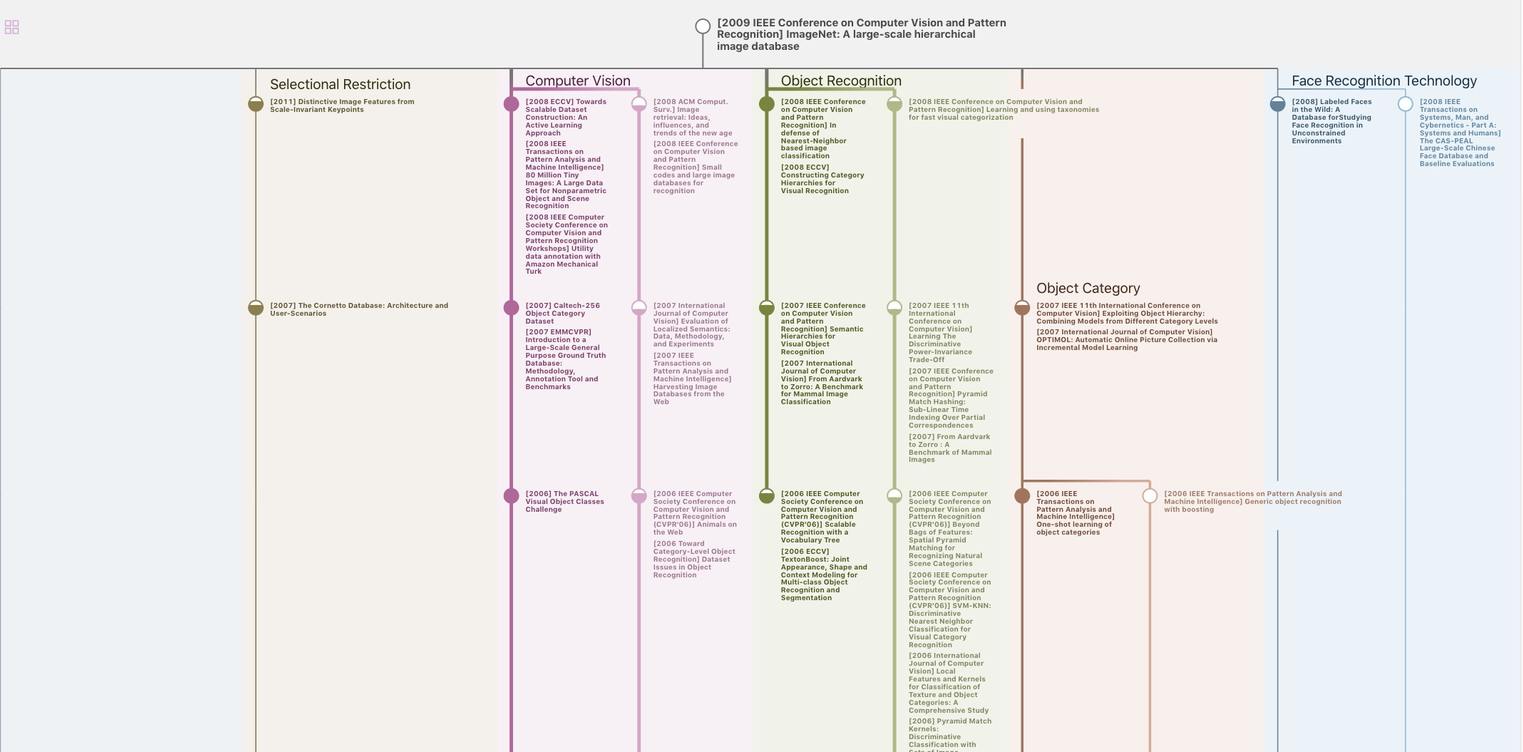
生成溯源树,研究论文发展脉络
Chat Paper
正在生成论文摘要