Seamless and automated rapeseed mapping for large cloudy regions using time-series optical satellite imagery
ISPRS Journal of Photogrammetry and Remote Sensing(2022)
摘要
Accurate field-level mapping of rapeseed spatial distributions using remote sensing technology supports agricultural planning, food and edible oil security, and ecological system management. The phenological and spectral methods for rapeseed mapping mainly rely on optical images collected during the flowering period. However, these images are often occluded by clouds. Moreover, the scarcity of well-represented training samples with various phenology patterns constrains large-scale supervised classification and extraction of rapeseed. To solve these problems, we propose a novel Seamless and Automated Rapeseed Mapping (SARM) method using time-series optical satellite imagery for large cloudy regions to generate seamless rapeseed maps at field scale without any manually collected samples. First, a Winter Rapeseed Index (WRI) was designed to automatically extract rapeseed in cloud-free areas by enhancing the phenological separability of rapeseed. Second, a multiple random forest-based fusion mapping framework was developed using WRI-based training samples, and was applied for the cloudy regions to map rapeseed seamlessly across the whole study area. The proposed SARM method was validated under different years, sensors, and cropping patterns at four study sites both in China and the USA. Extensive experimental results and analysis suggest that the SARM method outperforms other traditional methods on all four study sites with an average overall accuracy varying from 88.58% to 98.78%. These results also reveal that our proposed approach is capable of expanding the time windows for rapeseed mapping from the significant flowering period to the insignificant flowering period. More importantly, the spatial incompleteness of rapeseed maps caused by cloud contamination was mitigated to some degree. The SARM method robustly delivers seamless and automated rapeseed mapping by using time-series optical satellite imagery, thus offering new insights for crop investigation, especially in large cloudy regions.
更多查看译文
关键词
Rapeseed mapping,Time-series optical satellite imagery,Large cloudy region,Winter Rapeseed Index,Phenology,Machine learning
AI 理解论文
溯源树
样例
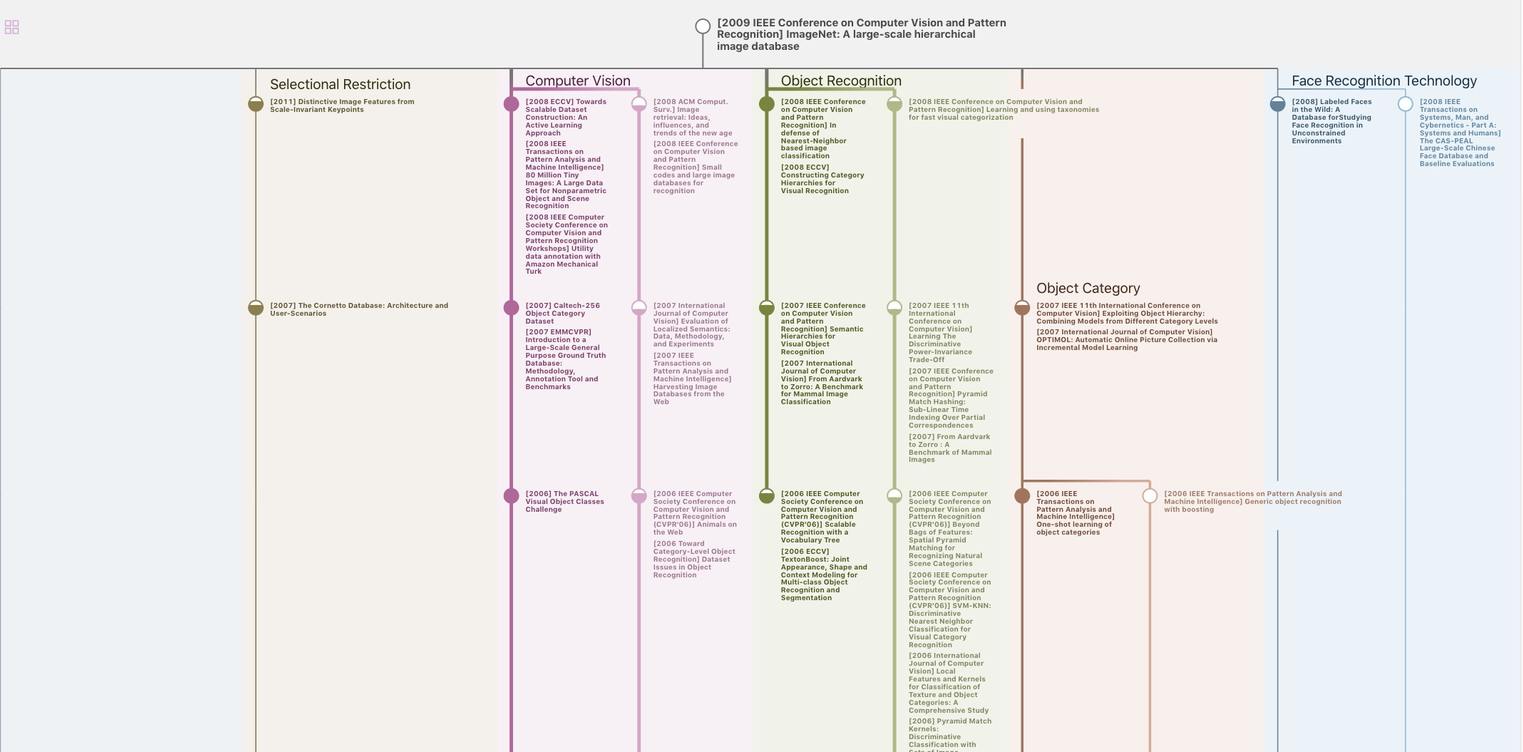
生成溯源树,研究论文发展脉络
Chat Paper
正在生成论文摘要