A Novel Framework Based on CNN-LSTM Neural Network for Prediction of Missing Values in Electricity Consumption Time-Series Datasets
JOURNAL OF INFORMATION PROCESSING SYSTEMS(2022)
摘要
Adopting Internet of Things (IoT)-based technologies in smart homes helps users analyze home appliances electricity consumption for better overall cost monitoring. The IoT application like smart home system (SHS) could suffer from large missing values gaps due to several factors such as security attacks, sensor faults, or connection errors. In this paper, a novel framework has been proposed to predict large gaps of missing values from the SHS home appliances electricity consumption time-series datasets. The framework follows a series of steps to detect, predict and reconstruct the input time-series datasets of missing values. A hybrid convolutional neural network-long short term memory (CNN-LSTM) neural network used to forecast large missing values gaps. A comparative experiment has been conducted to evaluate the performance of hybrid CNN-LSTM with its single variant CNN and LSTM in forecasting missing values. The experimental results indicate a performance superiority of the CNN-LSTM model over the single CNN and LSTM neural networks.
更多查看译文
关键词
CNN-LSTM Neural Network, Electricity Consumption Prediction, Large Gaps of Missing Values, Prediction of Missing Values in Time-Series Data, Smart Home System
AI 理解论文
溯源树
样例
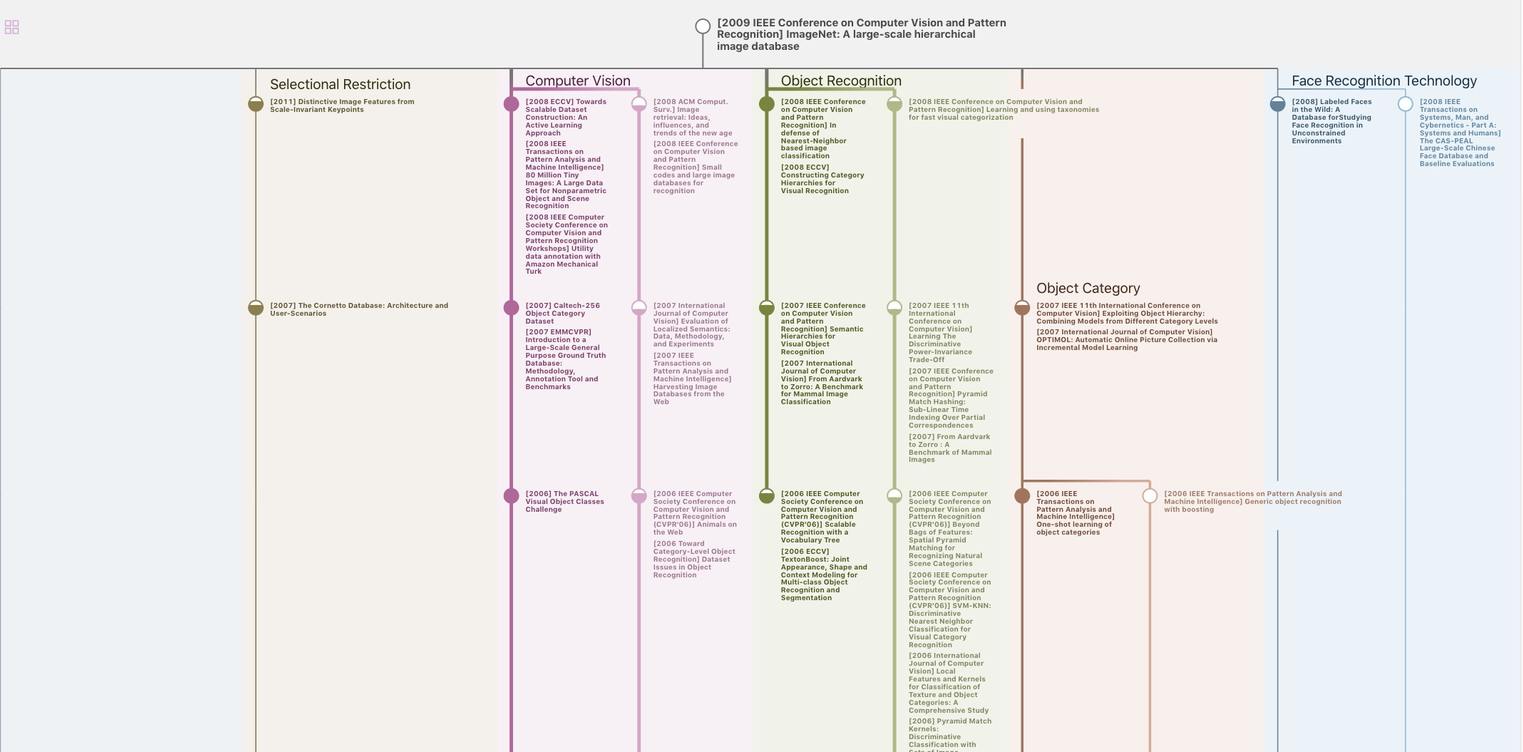
生成溯源树,研究论文发展脉络
Chat Paper
正在生成论文摘要