Measuring Group Separability in Geometrical Space for Evaluation of Pattern Recognition and Dimension Reduction Algorithms
IEEE ACCESS(2022)
摘要
Evaluating group separability is fundamental to pattern recognition. A plethora of dimension reduction (DR) algorithms has been developed to reveal the emergence of geometrical patterns in a low-dimensional space, where high-dimensional sample similarities are approximated by geometrical distances. However, statistical measures to evaluate the group separability attained by DR representations are missing. Traditional cluster validity indices (CVIs) might be applied in this context, but they present multiple limitations because they are not specifically tailored for DR. Here, we introduce a new rationale called projection separability (PS), which provides a methodology expressly designed to assess the group separability of data samples in a DR geometrical space. Using this rationale, we implemented a new class of indices named projection separability indices (PSIs) based on four statistical measures: Mann-Whitney U-test p-value, Area Under the ROC-Curve, Area Under the Precision-Recall Curve, and Matthews Correlation Coefficient. The PSIs were compared to six representative cluster validity indices and one geometrical separability index using seven nonlinear datasets and six different DR algorithms. The results provide evidence that the implemented statistical-based measures designed on the basis of the PS rationale are more accurate than the other indices and can be adopted not only for evaluating and comparing group separability of DR results but also for fine-tuning DR algorithms' hyperparameters. Finally, we introduce a second methodological innovation termed trustworthiness, a statistical evaluation that accounts for separability uncertainty and associates to the measure of each index a p-value that expresses the significance level in comparison to a null model.
更多查看译文
关键词
Indexes, Dimensionality reduction, Clustering algorithms, Biomedical measurement, Pattern recognition, Shape, Principal component analysis, Pattern recognition, dimension reduction, data embedding, group separability, cluster validity indices
AI 理解论文
溯源树
样例
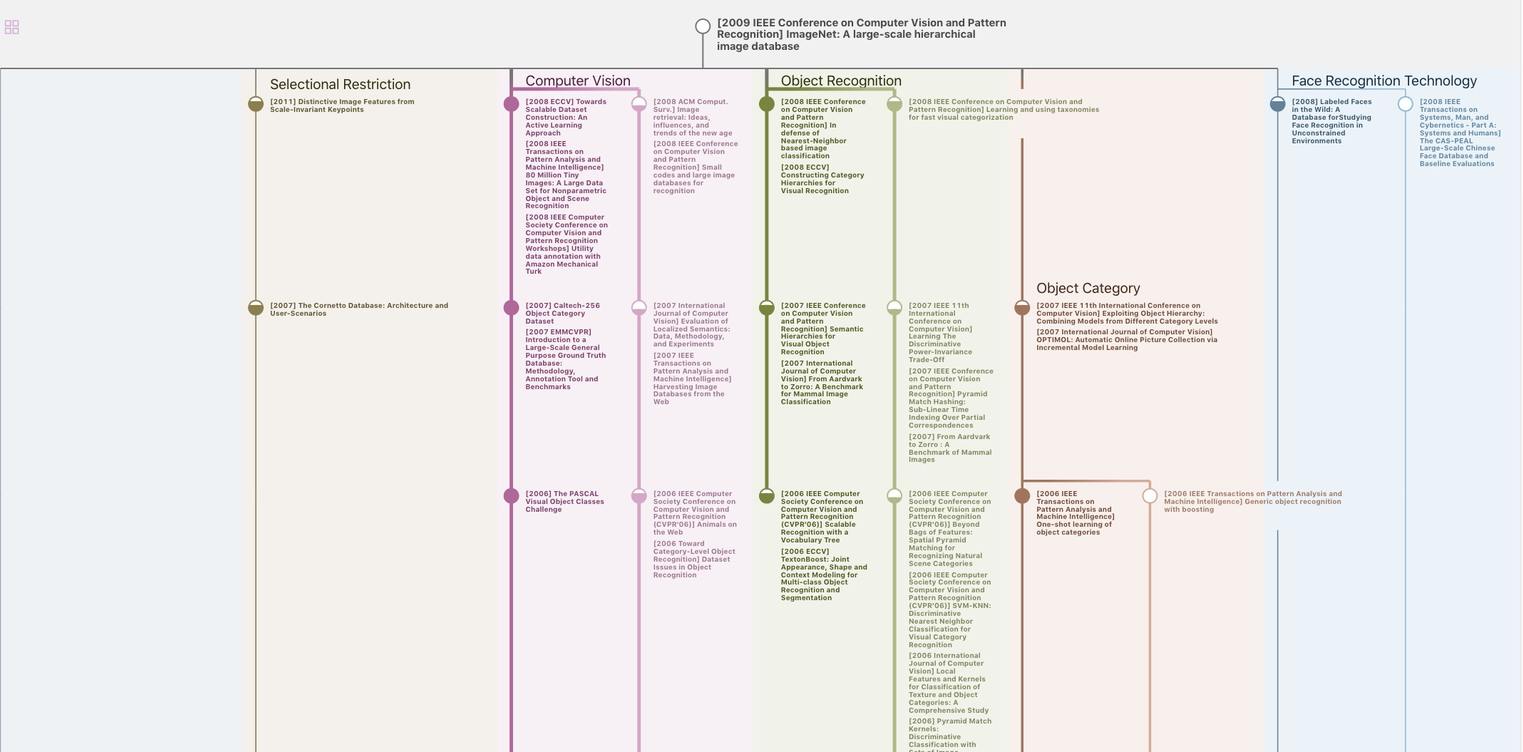
生成溯源树,研究论文发展脉络
Chat Paper
正在生成论文摘要