Dynamic System Modeling of a Hybrid Neural Network with Phase Space Reconstruction and a Stability Identification Strategy
MACHINES(2022)
摘要
Focusing on the identification of dynamic system stability, a hybrid neural network model is proposed in this research for the rotating stall phenomenon in an axial compressor. Based on the data fusion of the amplitude of the spatial mode, the nonlinear property is well characterized in the feature extraction of the rotating stall. This method of data processing can effectively avoid the inaccurate recognition of single or multiple measuring sensors only depending on pressure. With the analysis on the spatial mode, a chaotic characteristic was shown in the development of the amplitude with the first-order spatial mode. With the prerequisite of revealing the essence of this dynamic system, a hybrid radial basis function (RBF) neural network was adopted to represent the properties of the system by artificial intelligence learning. Combining the advantages of the methods of K-means and Gradient Descent (GD), the Chaos-K-means-GD-RBF fusion model was established based on the phase space reconstruction of the chaotic sequence. Compared with the two methods mentioned above, the calculation accuracy was significantly improved in the hybrid neural network model. By taking the strategy of global sample entropy and difference quotient criterion identification, a warning of inception can be suggested in advance of 12.3 revolutions (296 ms) with a multi-step prediction before the stall arrival.
更多查看译文
关键词
dynamic system stability, hybrid neural network, spatial modal amplitude, chaotic phase space reconstruction, global sample entropy, identification strategy
AI 理解论文
溯源树
样例
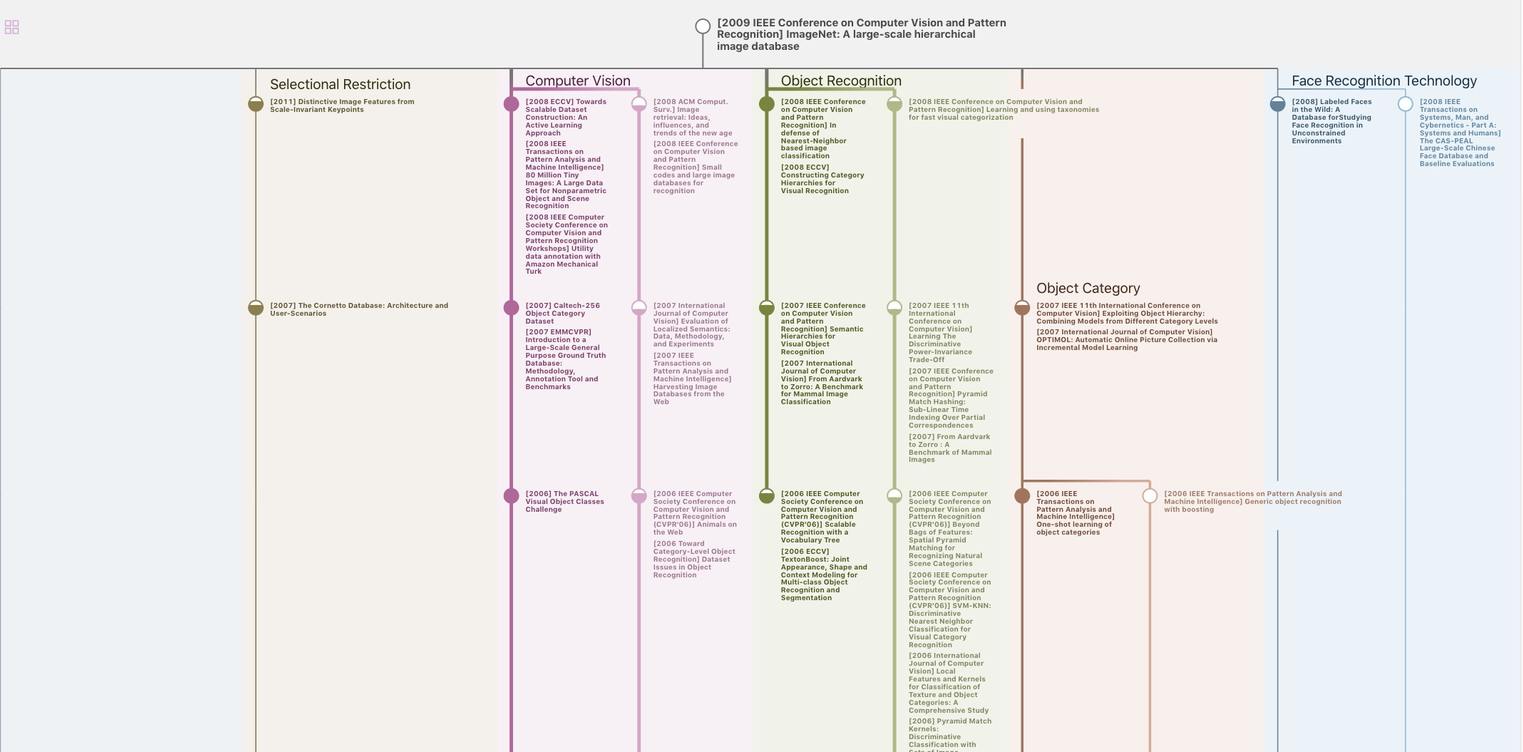
生成溯源树,研究论文发展脉络
Chat Paper
正在生成论文摘要