Identifying discriminatory feature-vectors for fusion-based iris liveness detection
JOURNAL OF AMBIENT INTELLIGENCE AND HUMANIZED COMPUTING(2022)
摘要
In recent, several fusion-based iris liveness detection techniques utilizing multiple feature extraction methods have been proposed, where the resultant feature vectors are combined using an appropriate fusion mechanism. However, such techniques do not follow any specific procedure to select the feature extraction methods; instead, random features are combined. This paper employs an existing Friedman test-based feature selection method to identify best k features out of N, where k < N; to constitute an optimal feature set, whose score-level fusion leads to correct prediction of iris presentation attacks. This feature selection method is utilized in cases where fusion is performed at the classifier’s outputs. In specific, instead of fusing the feature vectors, the outputs of the classifiers trained on such feature vectors are combined. The optimal feature set is validated by investigating their score-level fusion with various fusion-based state-of-the-arts. Further, the choice of score-level fusion over majority voting, feature-level fusion, and rank-level fusion methods is also validated. The experimental outcomes show that the iris liveness detection mechanism presented in this paper exceeds the performance benchmarks on multiple iris datasets.
更多查看译文
关键词
Friedman test, Fusion-based iris PAD, Liveness detection, Optimal feature-set, Score-level fusion
AI 理解论文
溯源树
样例
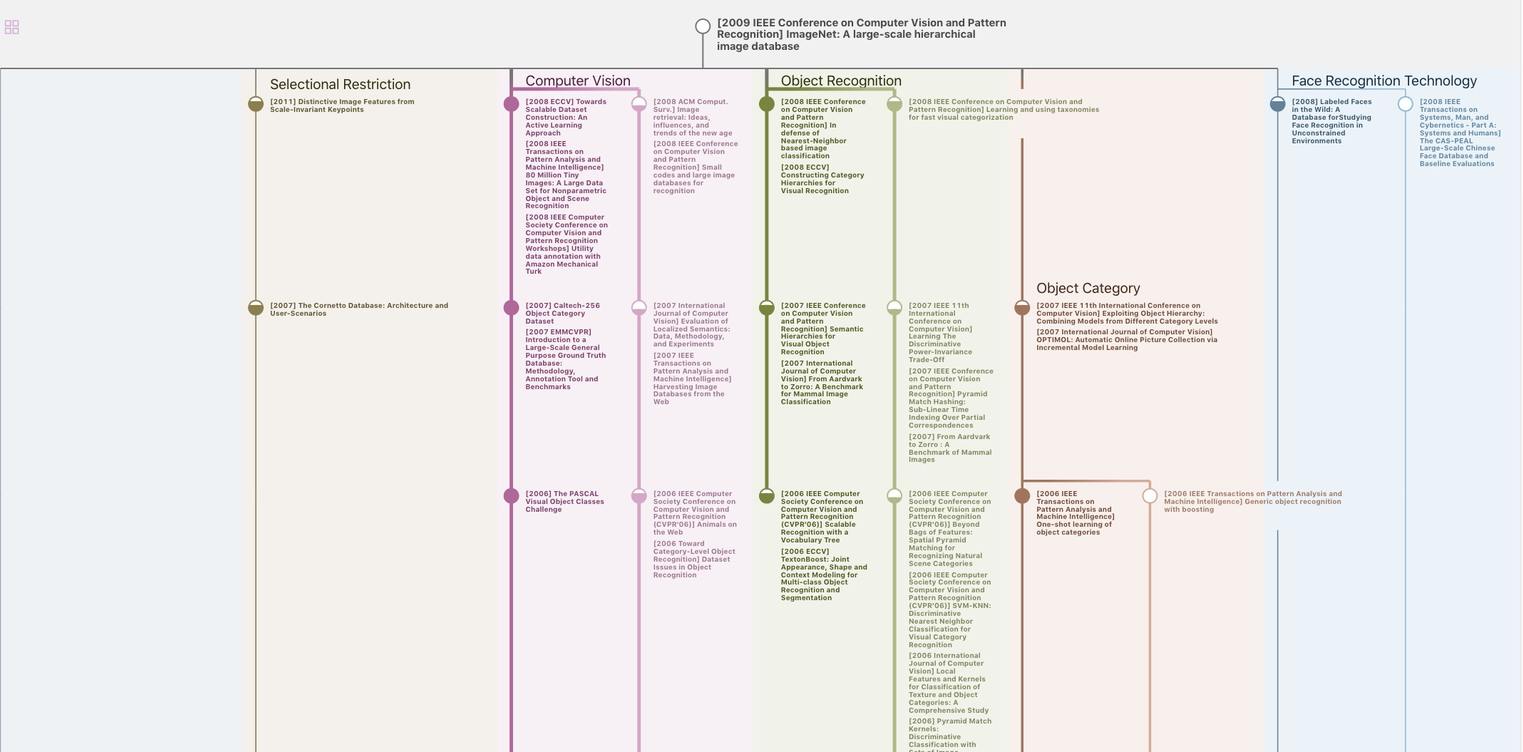
生成溯源树,研究论文发展脉络
Chat Paper
正在生成论文摘要