Multilevel surrogate modeling of an amine scrubbing process for CO(2)capture
AICHE JOURNAL(2022)
摘要
Surrogate models provide a powerful method for simplifying calculations within complex simulations. While surrogate models are broadly applied within chemical engineering, little research exists investigating the level of surrogacy's impact on a simplified process model. In this work, artificial neural networks (ANN) and Kriging models are used as surrogate models at the process, process unit, and thermodynamic levels for a CO2 amine scrubbing process. The surrogated models are evaluated against an Aspen Plus simulation for accuracy, convergence behavior, computational cost, and ability to extrapolate. The thermodynamic and process unit models can better handle discontinuous, non-smooth behavior, and convergence issues in the surrogated truth model, but poor conditioning in the final system of equations results in a lower accuracy and convergence rate than the process level surrogate. Beyond model accuracy, availability of diverse data, intended re-usability, and the desired outputs must be considered when selecting a level of abstraction.
更多查看译文
关键词
artificial neural networks, black-box model, Kriging, shortcut model, simulation
AI 理解论文
溯源树
样例
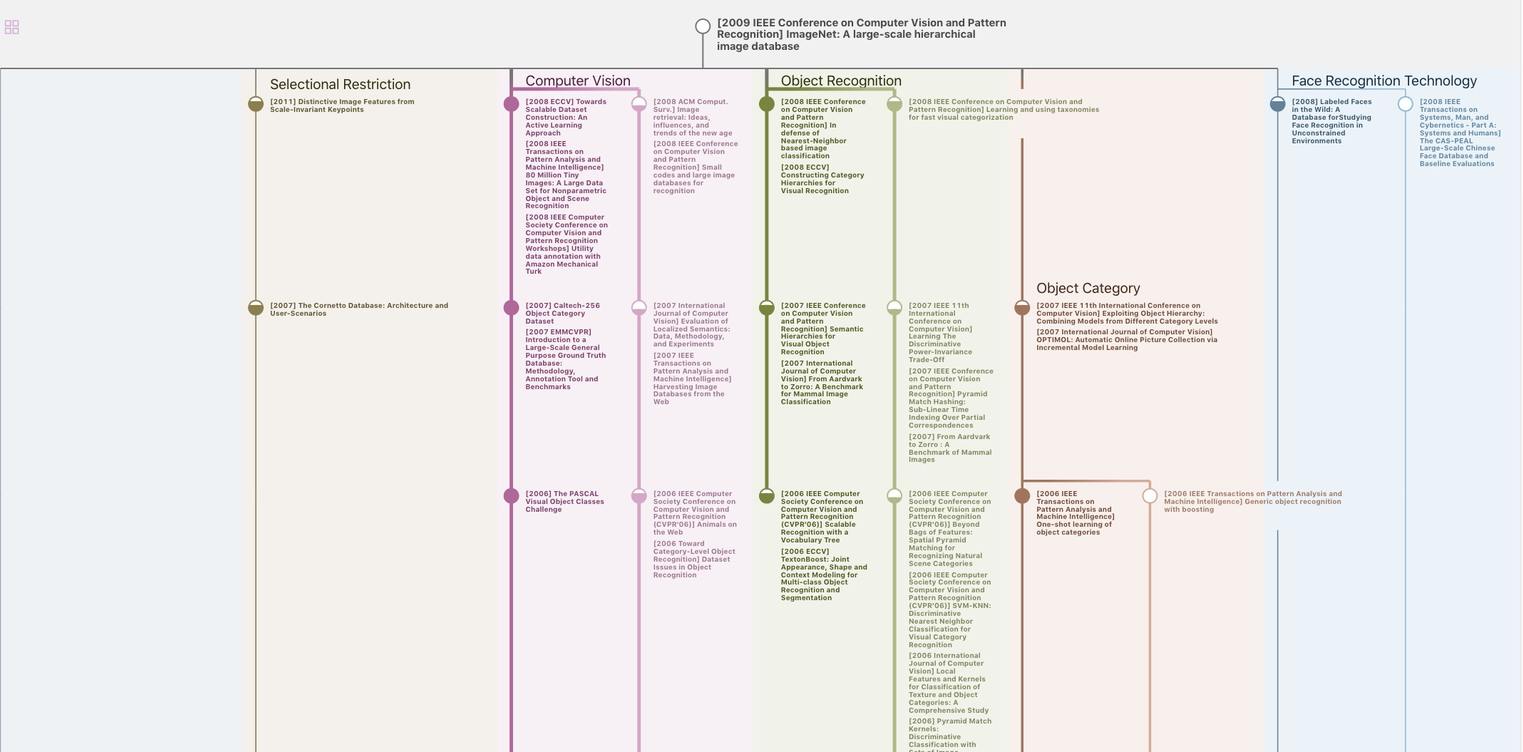
生成溯源树,研究论文发展脉络
Chat Paper
正在生成论文摘要