Bayesian two-part modeling of phytoplankton biomass and occurrence
HYDROBIOLOGIA(2022)
摘要
Phytoplankton biomass data often involve zero outcomes preventing a description by continuous distributions with positive support such as the lognormal distribution commonly used to describe ecological data. Two usual solutions: ignoring the zeroes and adding a small positive number to all outcomes, induce bias and reduce predictive power. To address these shortcomings, we design a Bayesian two-part model with a binary component for presence or absence and a continuous component involving a lognormal model for non-zero biomass. We specify two equations relating species-specific occurrence probabilities and expected log-biomasses when present to potential covariates, with spike-and-slab priors imposed on linear effects to selectively discard the irrelevant predictors. We analyze the biomass data of 74 phytoplankton (57 diatoms and 17 dinoflagellates) recorded weekly at Station L4 (Western English Channel, UK) between April 2003 and December 2009, along with measurements of abiotic covariates. Our results disclose different combinations of environmental predictors for the occurrence and the biomass of individual species. Overall, the occurrence of dinoflagellates is associated with higher temperature and irradiance levels compared to diatoms, with virtually no dependence on nutrient concentrations. Irradiance emerges as the key predictor of biomass when species are present. Optimum temperatures for biomass accumulation and temperature sensitivities vary widely among and within functional types. Compared to one-stage models based on usual zero-handling approaches, our two-part model stands out with higher prediction accuracy. The two-part modeling approach provides a valuable framework for decoupling the predictors of species occurrence and abundance from observational data.
更多查看译文
关键词
Bayesian inference, Delta distribution, Hurdle model, Over dispersion, Semi-continuous data, Stochastic search variable selection
AI 理解论文
溯源树
样例
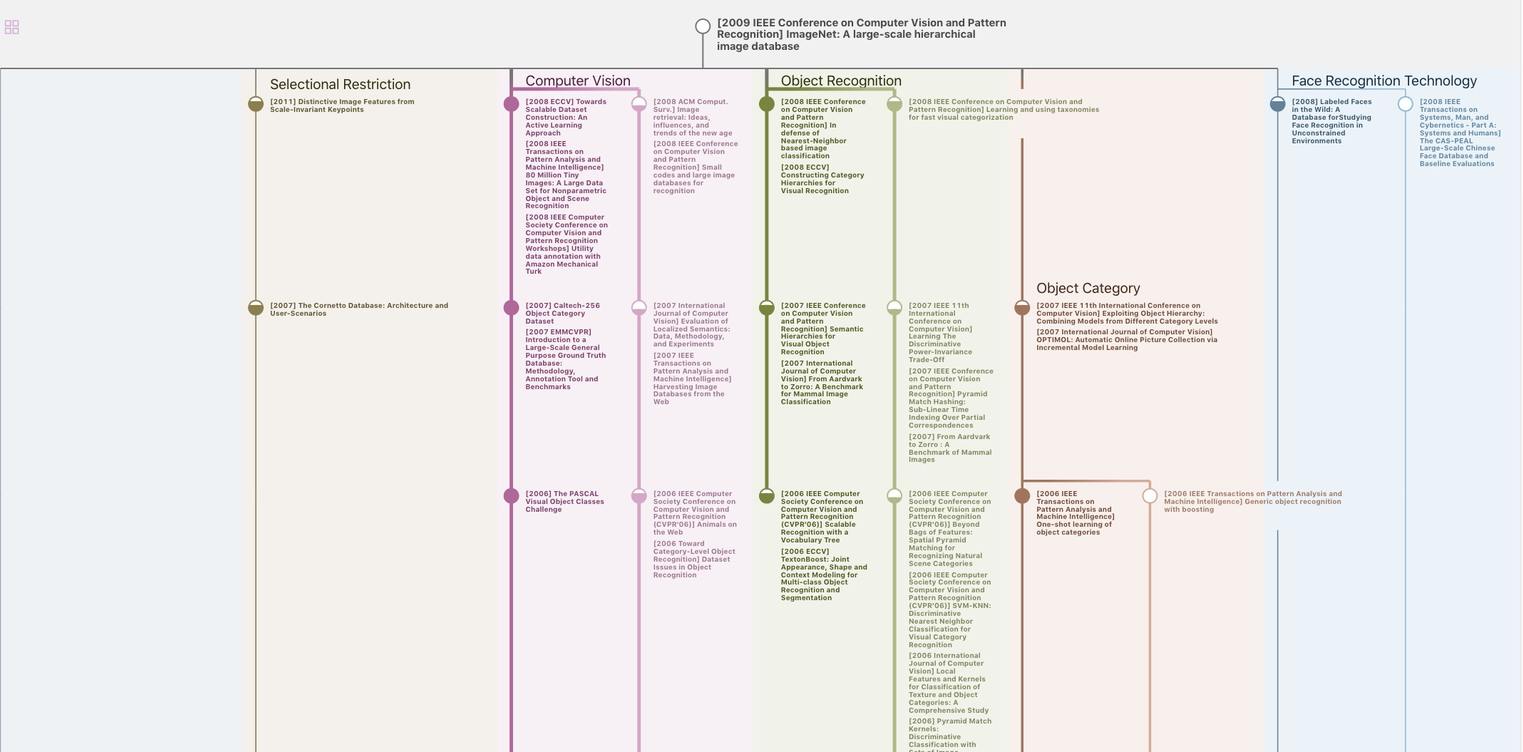
生成溯源树,研究论文发展脉络
Chat Paper
正在生成论文摘要