New incremental SVM algorithms for human activity recognition in smart homes
JOURNAL OF AMBIENT INTELLIGENCE AND HUMANIZED COMPUTING(2022)
摘要
Smart homes are equipped with several sensor networks to keep an eye on both residents and their environment, to interpret the current situation and to react immediately. Handling large scale dataset of sensory events on real time to enable efficient interventions is challenging and very difficult. To deal with these data flows and challenges, traditional streaming data classification approaches can be boosted by use of incremental learning. In this paper, we presented two new Incremental SVM methods to improve the performance of SVM classification in the context of human activity recognition tasks. Two feature extraction methods elaborated by refining dependency sensor extraction feature and focusing on the last sensor event only have been suggested. On the other hand, a clustering based approach and a similarity based approach have been suggested to boost learning performance of the incremental SVM algorithms capitalizing on the relationship between data chunk and support vectors of previous chunk. We demonstrate through several simulations on two major publicly available data sets (Aruba and Tulum), the feasibility and improvements in learning and classification performances in real time achieved by our proposed methods over the state-of-the-art. For instance, we have shown that the introduced similarity-based incremental learning is 5 to 9 times faster than other methods in terms of training performances. Similarly, the introduced Last-state sensor feature method induces at least 5% improvement in terms of F1-score when using baseline SVM classifier.
更多查看译文
关键词
Smart home, Activity recognition, Incremental learning, Incremental SVM
AI 理解论文
溯源树
样例
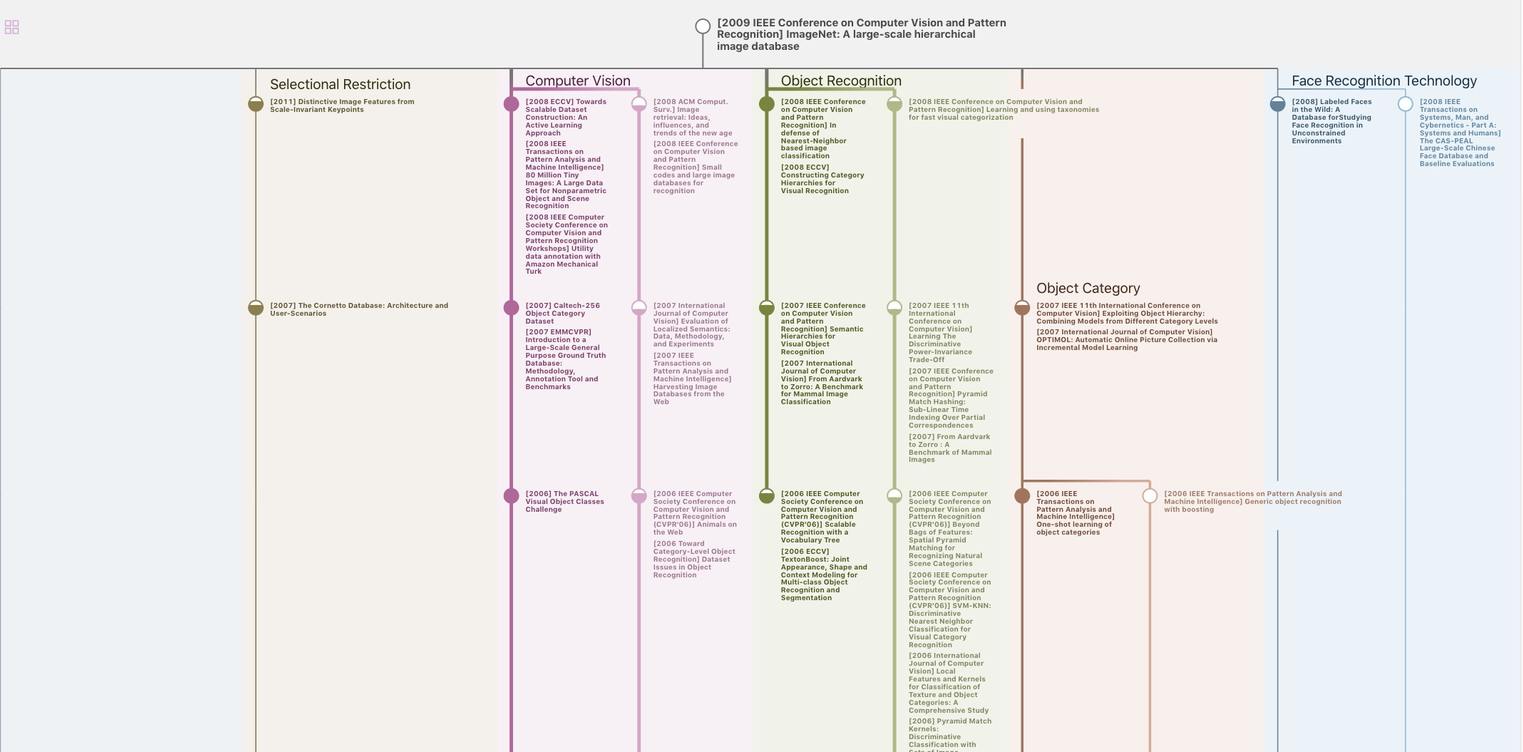
生成溯源树,研究论文发展脉络
Chat Paper
正在生成论文摘要