Few-shot RUL estimation based on model-agnostic meta-learning
JOURNAL OF INTELLIGENT MANUFACTURING(2022)
摘要
Data-driven remaining useful life (RUL) estimation has been a research hotspot in the prognostic and health management (PHM) of industrial equipment and systems. It can achieve predictive maintenance of machinery and rarely require prior expertise in prognostics and signal processing. However, the data-driven methods require sufficient training data, which is difficult to acquire. In this paper, we employ the model-agnostic meta-learning (MAML) algorithm to seek suitable model parameter initialization that can rapidly adapt to the given test sample with few-shot training samples. We also propose to build pseudo-meta-RUL task sets for meta-learning by calculating time sequence similarities. To further improve the applicability of the model, we extend the proposed method from few-shot conditions to general conditions. We conduct experiments on the C-MAPSS dataset and the results show that the proposed algorithm can improve the prediction performance and enhance the generalization ability of the model in the context of few-shot conditions and general conditions.
更多查看译文
关键词
Remaining useful life estimation, Similarity matching, Meta-Learning
AI 理解论文
溯源树
样例
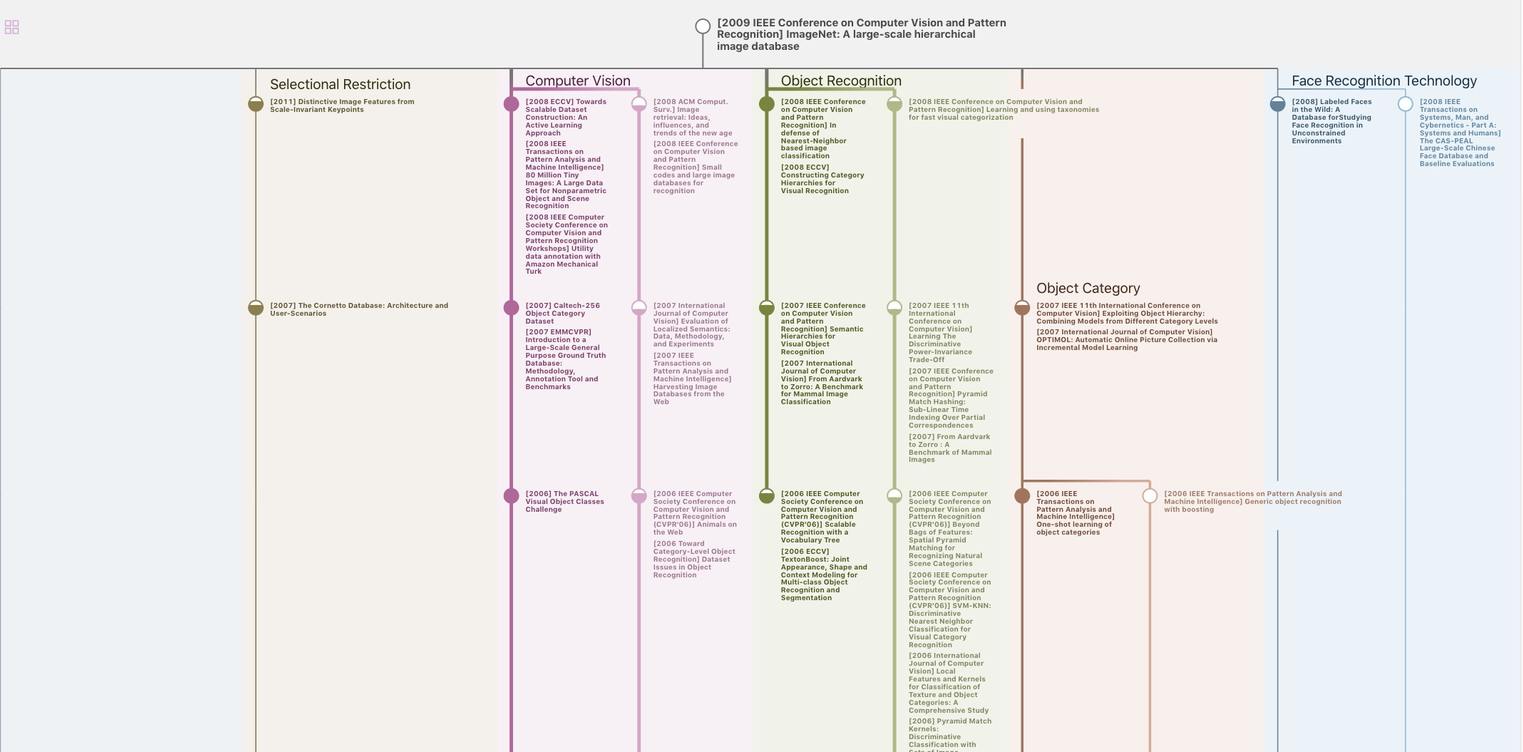
生成溯源树,研究论文发展脉络
Chat Paper
正在生成论文摘要