Object Detection in Autonomous Driving Scenarios Based on an Improved Faster-RCNN
APPLIED SCIENCES-BASEL(2021)
摘要
Object detection is one of the key algorithms in automatic driving systems. Aiming at addressing the problem of false detection and the missed detection of both small and occluded objects in automatic driving scenarios, an improved Faster-RCNN object detection algorithm is proposed. First, deformable convolution and a spatial attention mechanism are used to improve the ResNet-50 backbone network to enhance the feature extraction of small objects; then, an improved feature pyramid structure is introduced to reduce the loss of features in the fusion process. Three cascade detectors are introduced to solve the problem of IOU (Intersection-Over-Union) threshold mismatch, and side-aware boundary localization is applied for frame regression. Finally, Soft-NMS (Soft Non-maximum Suppression) is used to remove bounding boxes to obtain the best results. The experimental results show that the improved Faster-RCNN can better detect small objects and occluded objects, and its accuracy is 7.7% and 4.1% respectively higher than that of the baseline in the eight categories selected from the COCO2017 and BDD100k data sets.
更多查看译文
关键词
autonomous driving, object detection, small objects, occluded objects, Faster-RCNN
AI 理解论文
溯源树
样例
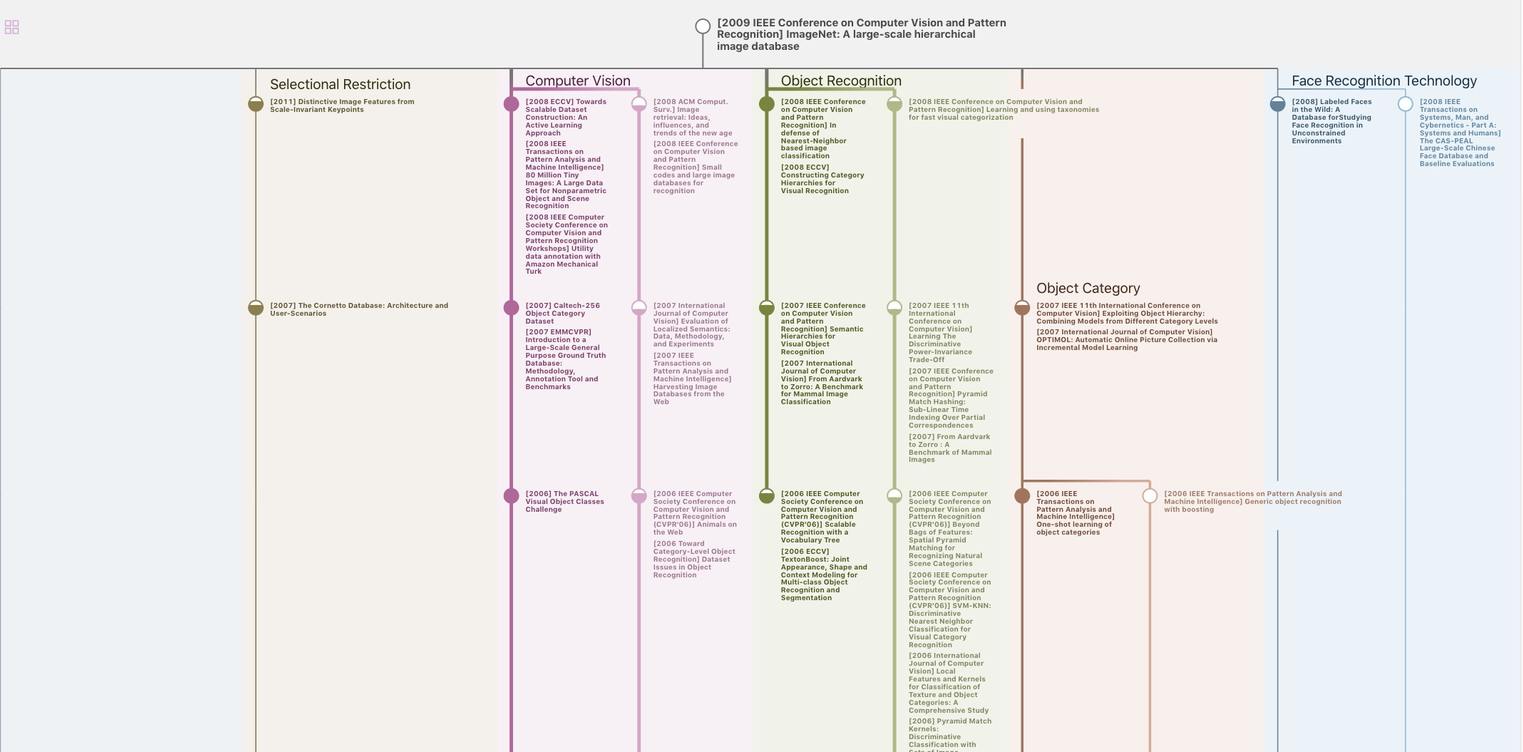
生成溯源树,研究论文发展脉络
Chat Paper
正在生成论文摘要