CNN-GRU network-based force prediction approach for variable working condition milling clamping points of deformable parts
The International Journal of Advanced Manufacturing Technology(2022)
摘要
Improper clamping is one of the major causes of part deformation. Improving the fixture arrangement through force analysis of clamping points is an effective means to suppress or improve machining deformation. However, the existing research focuses on the monitoring and off-line optimization of the clamping point force, which has a certain lag on the machining deformation control, and it is difficult to predict the clamping point force due to the time-varying coupling effect of multiple factors such as process parameters, cutting force, and clamping point force in the machining process. Inspired by the excellent performance of convolutional neural networks and gated recurrent networks in feature extraction and learning of temporal association laws, this paper proposes a CNN-GRU-based method for predicting the force state of clamping points under variable working conditions. Firstly, a force prediction model of clamping point during milling process with variable working conditions is established. Secondly, a convolutional neural network is designed to extract the features of dynamic coupled machining conditions. Then, a network of gated recurrent units is constructed to learn the temporal correlation law between the machining conditions and the forces on the clamping points to achieve force prediction of the clamping points during machining. Finally, it was verified by the milling process of the piston skirt. The results show that CNN-GRU can effectively predict the clamping force. In addition, CNN-GRU has higher computational efficiency and accuracy compared with CNN-LSTM, CNN-RNN and CNN-BP.
更多查看译文
关键词
Clamping point force prediction,Variable working conditions,Convolutional neural network,Gated recurrent unit,Temporal correlation,Deformable parts
AI 理解论文
溯源树
样例
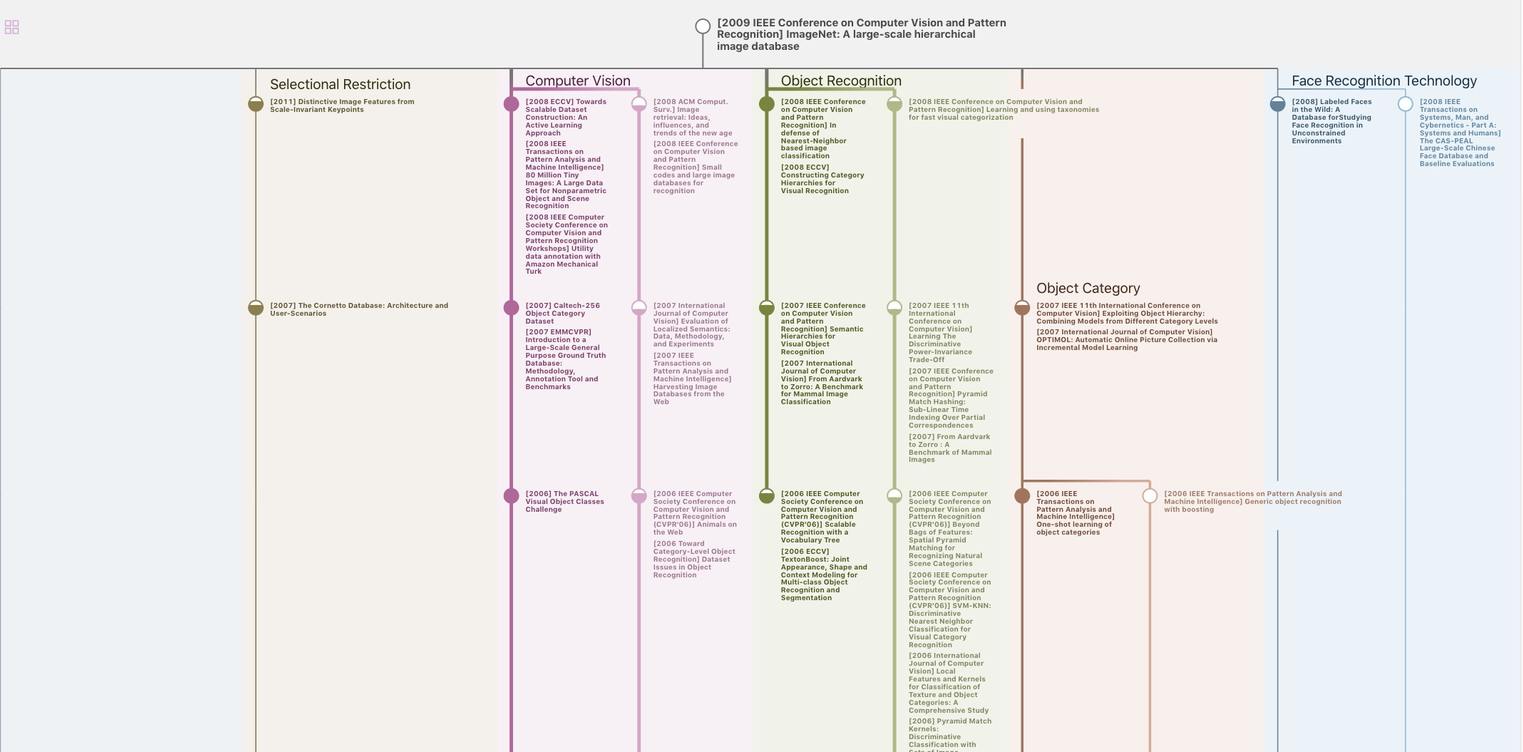
生成溯源树,研究论文发展脉络
Chat Paper
正在生成论文摘要