Defect Detection in Woven Fabrics by Analysis of Co-occurrence Texture Features as a Function of Gray-level Quantization and Window Size
ENGINEER-JOURNAL OF THE INSTITUTION OF ENGINEERS SRI LANKA(2021)
摘要
In this experimental research, the effects of gray-level quantization and tiling window size on 22 gray-level co-occurrence matrix features were investigated in the context of automated woven fabric defect detection. A dataset comprising 1426 128x128 images was used, in which defective and the defect-free images were split in a 50:50 ratio. Experiments were carried out with seven quantization levels (L = 4, 8, 16, 32, 64, 128 and 256) and four window sizes (N = 8, 16, 32, 64). The features were extracted from each image in the training set for eachcombination and thereafter were ranked using the joint mutual information metric. Next, for each combination, a k-nearest neighbour classifier was trained, first with only the highest-ranking feature and thereafter iteratively by adding features of lower ranks. It was observed that a minimum of nine features were needed to achieve an acceptable (>90%) F1 score for any combination, except when N is relatively large. The two features that contribute to improving the F1 score for any combination were found to be Homogeneity I and Homogeneity II. It was also noted that using an 8x8 window on images with 128 gray levels resulted in a practically usable high F1 score (96.39%) with the least number of features (14).
更多查看译文
关键词
Fabric defect detection, GLCM, Gray-level quantization, Window size
AI 理解论文
溯源树
样例
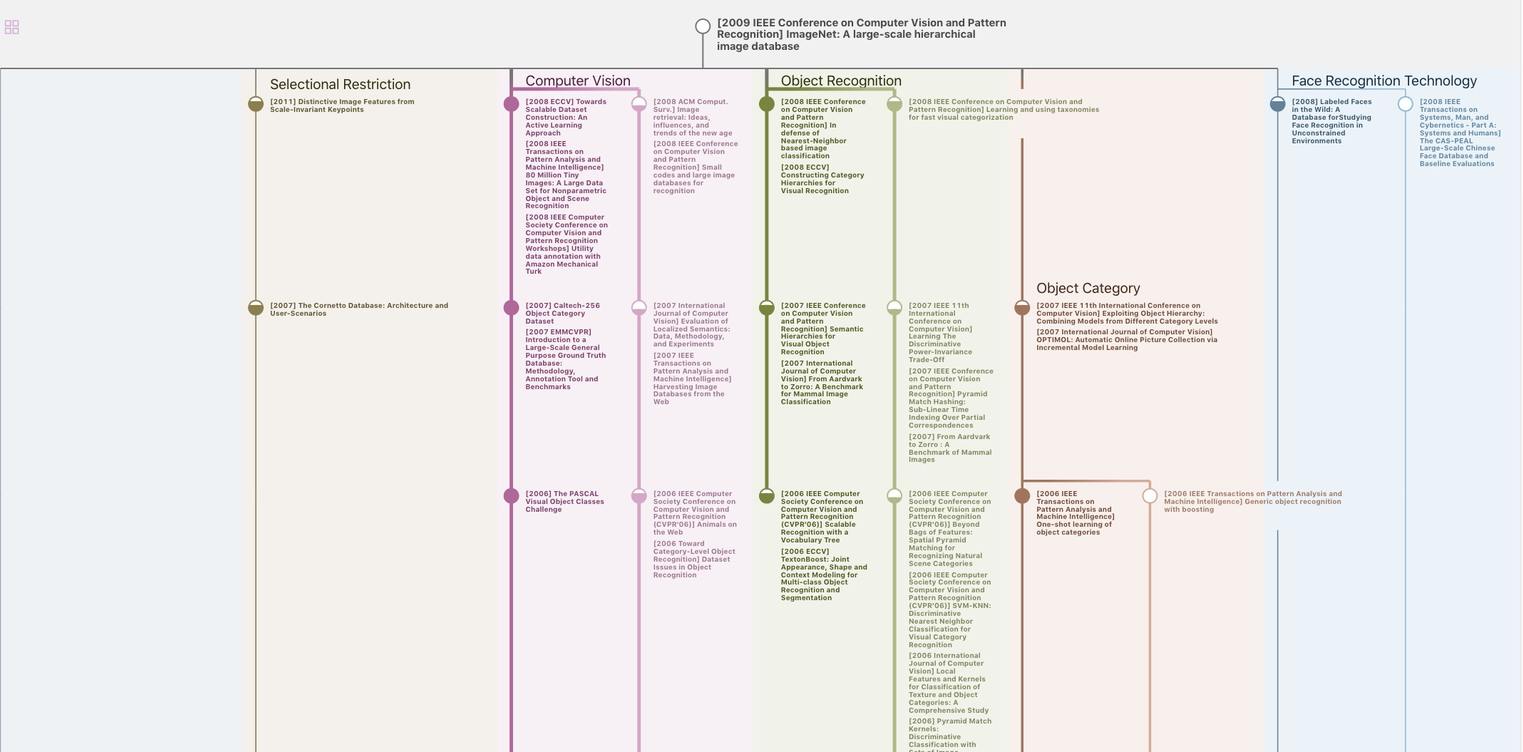
生成溯源树,研究论文发展脉络
Chat Paper
正在生成论文摘要