Human Gait Data Augmentation and Trajectory Prediction for Lower-Limb Rehabilitation Robot Control Using GANs and Attention Mechanism
MACHINES(2021)
摘要
To date, several alterations in the gait pattern can be treated through rehabilitative approaches and robot assisted therapy (RAT). Gait data and gait trajectories are essential in specific exoskeleton control strategies. Nevertheless, the scarcity of human gait data due to the high cost of data collection or privacy concerns can hinder the performance of controllers or models. This paper thus first creates a GANs-based (Generative Adversarial Networks) data augmentation method to generate synthetic human gait data while still retaining the dynamics of the real gait data. Then, both the real collected and the synthesized gait data are fed to our constructed two-stage attention model for gait trajectories prediction. The real human gait data are collected with the five healthy subjects recruited from an optical motion capture platform. Experimental results indicate that the created GANs-based data augmentation model can synthesize realistic-looking multi-dimensional human gait data. Also, the two-stage attention model performs better compared with the LSTM model; the attention mechanism shows a higher capacity of learning dependencies between the historical gait data to accurately predict the current values of the hip joint angles and knee joint angles in the gait trajectory. The predicted gait trajectories depending on the historical gait data can be further used for gait trajectory tracking strategies.
更多查看译文
关键词
gait prediction, attention, lower-limb rehabilitation robot, LSTM
AI 理解论文
溯源树
样例
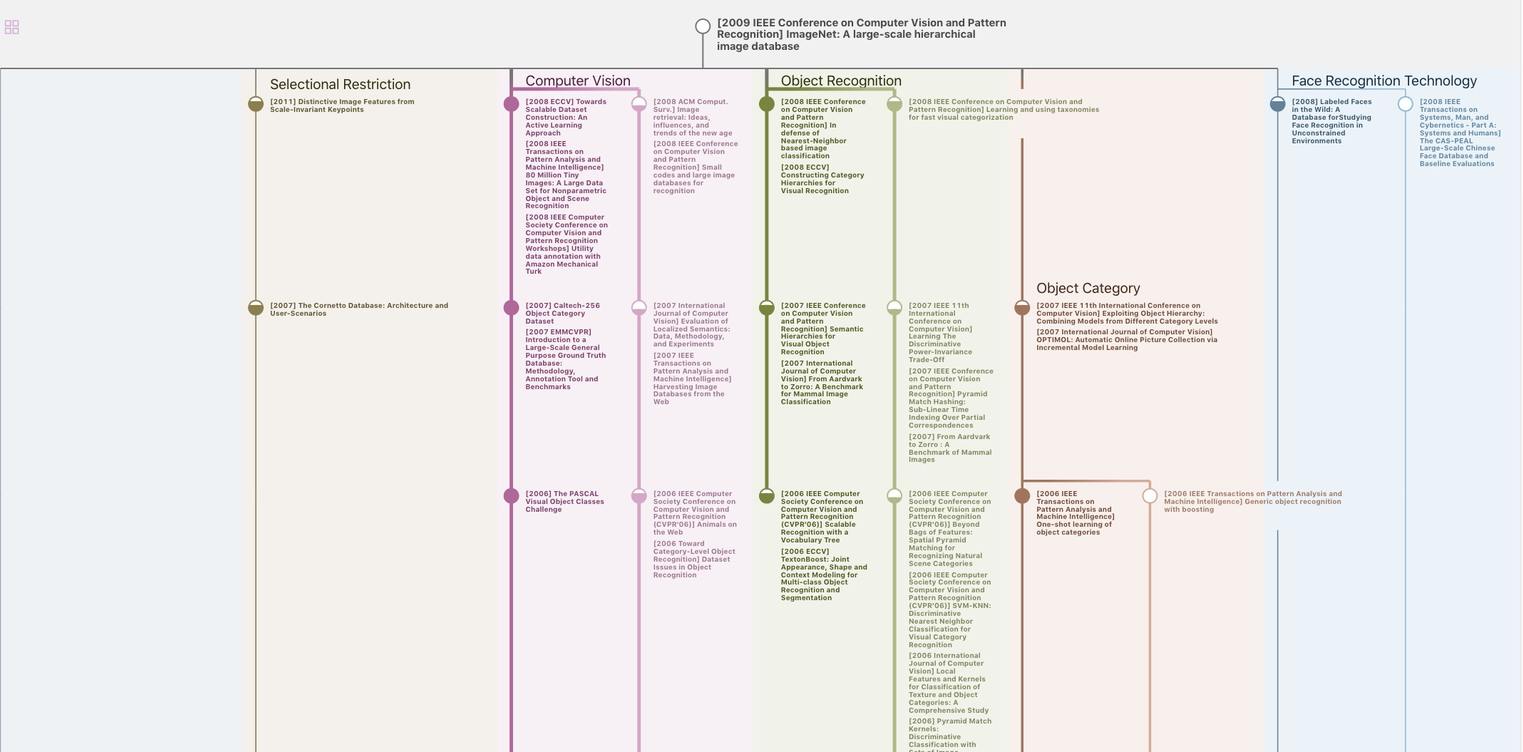
生成溯源树,研究论文发展脉络
Chat Paper
正在生成论文摘要