Multimodal image sensor fusion in a cascaded framework using optimized dual channel pulse coupled neural network
JOURNAL OF AMBIENT INTELLIGENCE AND HUMANIZED COMPUTING(2022)
摘要
Medical image fusion plays an important role in the clinical diagnosis of several critical diseases by merging the complementary information present in multimodal images and also assists the radiologists. Therefore, in this paper, a cascaded multimodal medical image fusion scheme is proposed using an optimized dual-channel biologically-inspired spiking neural model in two-scale hybrid ℓ _1 − ℓ _0 layer decomposition (HLD) and nonsubsampled shearlet transform (NSST) domain. The different features of HLD and NSST in a cascade framework are utilized to preserve more structural and textural information available in the source images with the suppression of significant noise and artifacts. At stage 1, the source images are subjected to HLD, the base layer is fused by choose max rule to preserve the local luminescence information, and detail layers at scale-1 and 2 are further decomposed by NSST. At stage 2, fusion rule based on laws of texture energy features is utilized to fuse low-frequency coefficients to highlight the local energy, contrast, and textures of the source images, while to fuse high-frequency coefficients, an optimized dual-channel biologically-inspired spiking neural model is utilized to maximize the retention of sharp edges and enhance the visual quality of fused images. Differential evolution is used for optimization which uses a fitness function based on the edge index of the resultant fused image. To analyze the fusion performance, extensive experiments are conducted on CT-MR-T2, MR-T2-SPECT neurological image dataset. Experimental results show that the proposed method provides better-fused images and outperforms the other existing fusion method in both visual and quantitative assessments.
更多查看译文
关键词
Image fusion, Multimodal, Medical imaging, Hybrid layer decomposition, Nonsubsampled shearlet decomposition, Optimization, Differential evolution
AI 理解论文
溯源树
样例
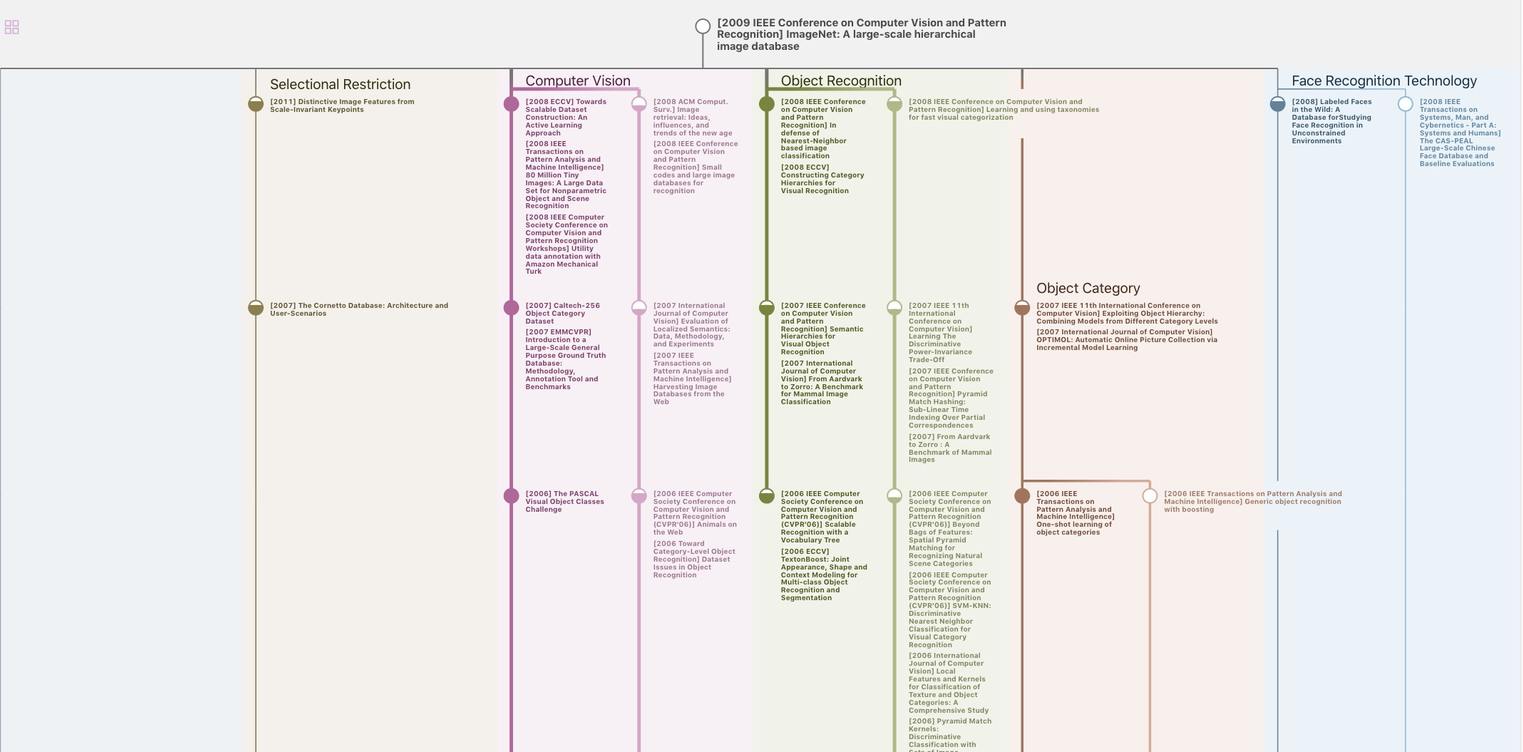
生成溯源树,研究论文发展脉络
Chat Paper
正在生成论文摘要