Data-Driven Prediction of Turbulent Flow Statistics Past Bridge Piers in Large-Scale Rivers Using Convolutional Neural Networks
WATER RESOURCES RESEARCH(2022)
摘要
Prediction of statistical properties of the turbulent flow in large-scale rivers is essential for river flow analysis. The large-eddy simulation (LES) provides a powerful tool for such predictions; however, it requires a very long sampling time and demands significant computing power to calculate the turbulence statistics of riverine flows. In this study, we developed encoder-decoder convolutional neural networks (CNNs) to predict the first- and second-order turbulence statistics of the turbulent flow of large-scale meandering rivers using instantaneous LES results. We train the CNNs using a data set obtained from LES of the flood flow in a large-scale river with three bridge piers-a training testbed. Subsequently, we employed the trained CNNs to predict the turbulence statistics of the flood flow in two different meandering rivers and bridge pier arrangements-validation testbed rivers. The CNN predictions for the validation testbed river flow were compared with the simulation results of a separately done LES to evaluate the performance of the developed CNNs. We show that the trained CNNs can successfully produce turbulence statistics of the flood flow in the large-scale rivers, that is, the validation testbeds.
更多查看译文
关键词
convolutional neural network, flood flow predictions, large-scale rivers, large-eddy simulation
AI 理解论文
溯源树
样例
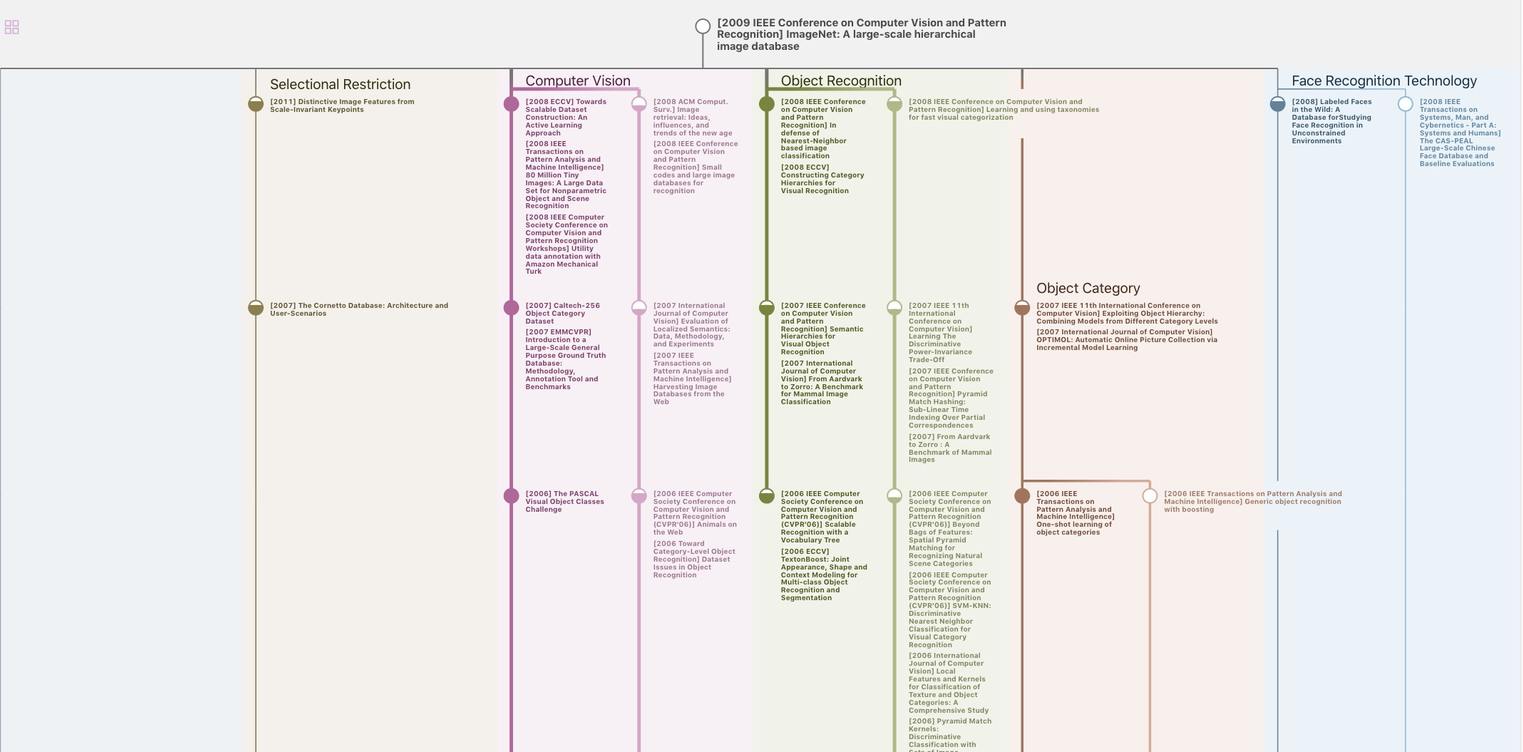
生成溯源树,研究论文发展脉络
Chat Paper
正在生成论文摘要