An Efficient Email Spam Detection Utilizing Machine Learning Approaches
INNOVATIVE DATA COMMUNICATION TECHNOLOGIES AND APPLICATION, ICIDCA 2021(2022)
摘要
In this paper, email information was categorized as ham email and spam email by utilizing directed learning calculations. The issues of email spam discovery framework are related with high dimensionality in element determination cycle and low exactness of spam email characterization. On the other hand, feature extraction is emerging as a globalized enhancement with the implication of artificial intelligence [AI] techniques to reduce the superfluous and repetitive data and generates satisfactory outcomes with high precision. With reference to these technologies, this paper presents an element determination calculation depending on the particle swarm optimization (PSO) with three ML approaches such as decision tree, support vector machine [SVM], and Naive Bayes. The proposed PSO-based component choice calculation looks through the element space for obtaining the best element subsets. The classifier execution and length of the selected highlight vector as a classifier input are considered for executing the assessment by utilizing UCI spambase dataset. Exploratory outcomes show that the PSO-based element choice calculation was introduced to create incredible component determination results with the insignificant arrangement of selected features, which has to be brought about by a high exactness of spam email order depending on three ML classifiers. The outcomes show that the SVM-PSO-based element determination technique can obtain the most elevated precision from 93.62 to 95.97%.
更多查看译文
关键词
PSO, ML, Spam, SVM, Decision tree, Naive Bayes
AI 理解论文
溯源树
样例
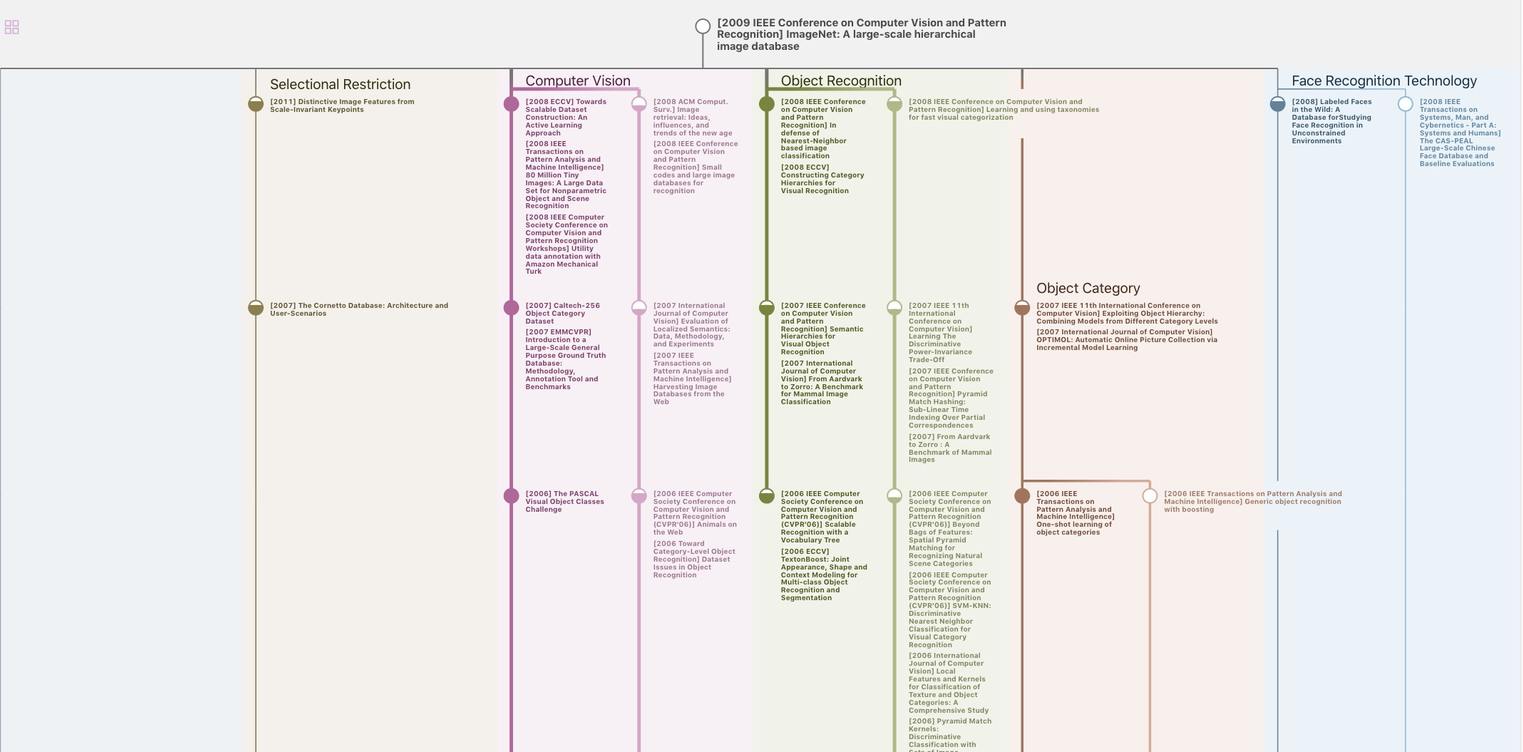
生成溯源树,研究论文发展脉络
Chat Paper
正在生成论文摘要