Searching for explanations: testing social scientific methods in synthetic ground-truthed worlds
COMPUTATIONAL AND MATHEMATICAL ORGANIZATION THEORY(2022)
摘要
A scientific model’s usefulness relies on its ability to explain phenomena, predict how such phenomena will be impacted by future interventions, and prescribe actions to achieve desired outcomes. We study methods for learning causal models that explain the behaviors of simulated “human” populations. Through the Ground Truth project, we solved a series of Challenges where our explanations, predictions and prescriptions were scored against ground truth information. We describe the processes that emerged for applying causal discovery, network analysis, agent-based modeling and other analytical methods to inform solutions to Challenge tasks. We present our team’s overall performance results on these Challenges and discuss implications for future efforts to validate social scientific research using simulation-based challenges.
更多查看译文
关键词
Causal discovery, Network analysis, Social networks
AI 理解论文
溯源树
样例
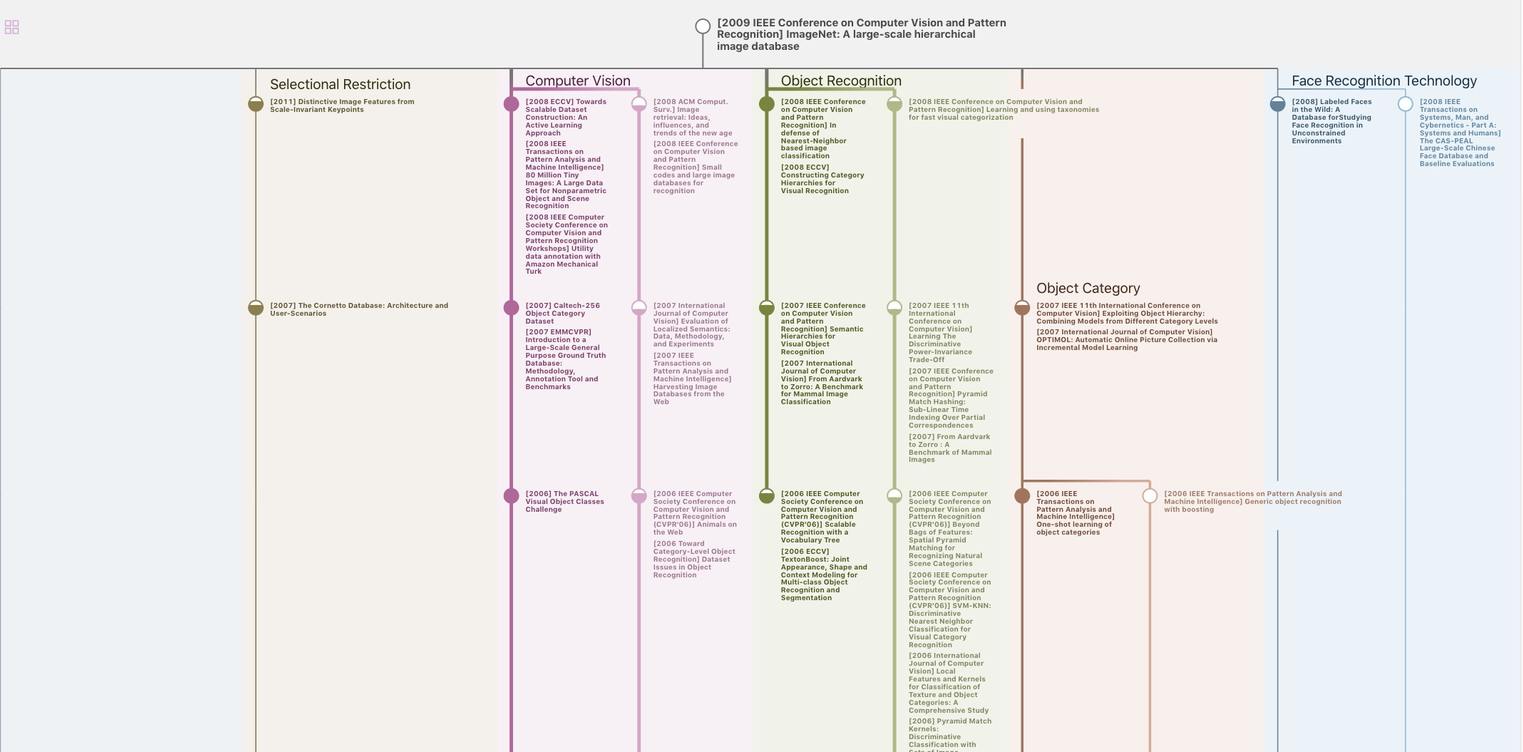
生成溯源树,研究论文发展脉络
Chat Paper
正在生成论文摘要