Neural Network Image Fusion with PCA Preprocessing
BIG DATA III: LEARNING, ANALYTICS, AND APPLICATIONS(2021)
摘要
The techniques for fusion of multi-spectral sensor datasets containing complementary information about the subject of observation facilitate visualization of the data into a form more easily interpreted by both humans and algorithms. Many applications of feature-level fusion seek to combine edges and textures, across the bandwidth of the sensory spectrum. Visualization techniques can be skewed by the introduction of corruption and redundancies induced by harmonics. A majority of image fusion techniques rely on intensity hue saturation (IHS) transforms, principal component analysis (PCA), and Gram Schmidt process. PCA's ability to remove the redundancy from a set of correlated data while preserving the variance and its resistance to color distortion lends itself to this data-fusion application. PCA also has a lower spectral distortion as compared to IHS and has been found to create superior image fusion. The application of neural network control techniques has been shown to more accurately recreate results similar to those found by human inference. Over the years, increased computation power has given rise to the spread of neural networks into roles previously carried out by humans. Select advanced image processing techniques have benefited greatly from their implementation. We propose a novel method of utilizing PCA in conjunction with a neural network to achieve a higher quality of image fusion. Implementation of an autoencoder neural network to fuse this information creates a higher level of data visualization when compared to traditional weighted fusion techniques.
更多查看译文
关键词
Image Fusion, Principal Component Analysis, Autoencoder
AI 理解论文
溯源树
样例
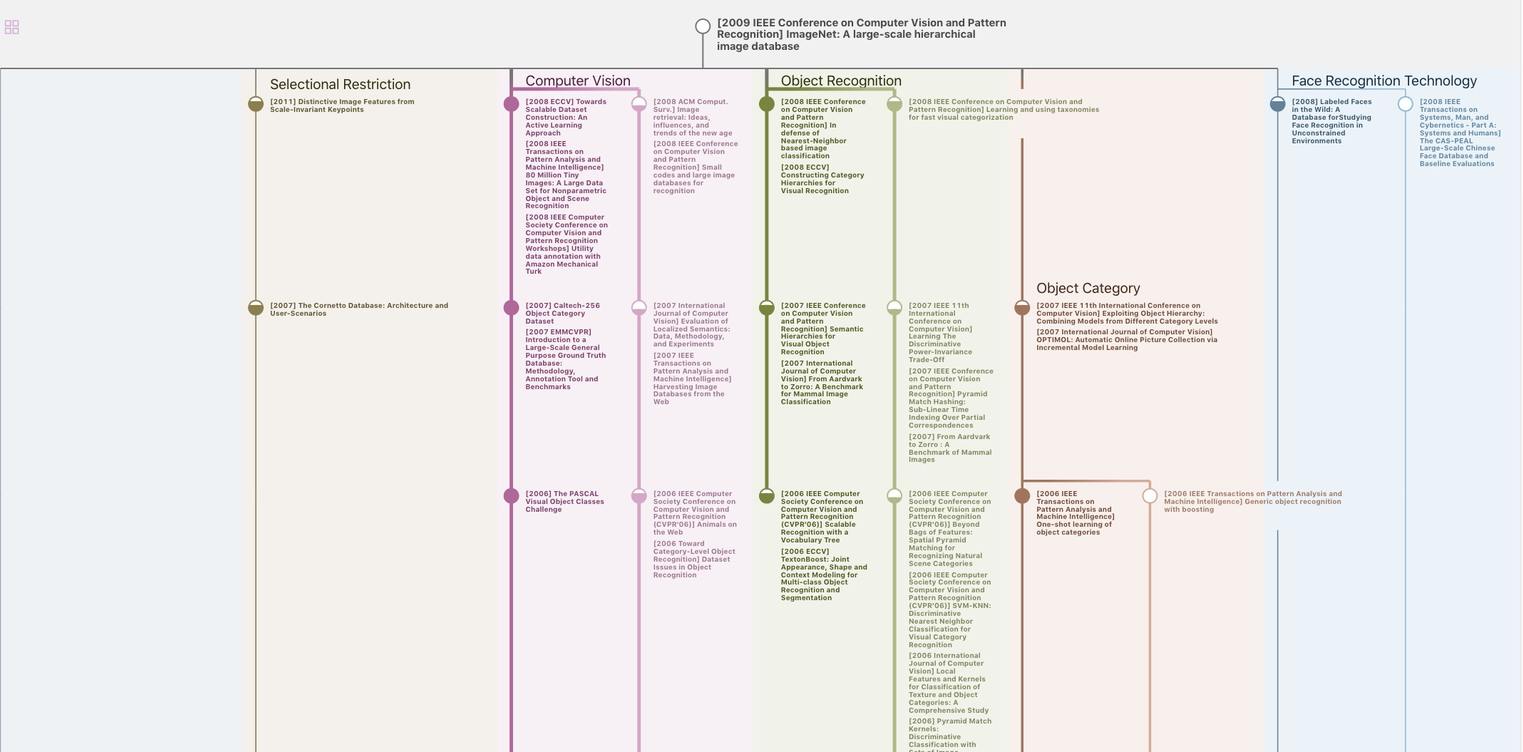
生成溯源树,研究论文发展脉络
Chat Paper
正在生成论文摘要