Semi-Supervised Semantic Segmentation of Remote Sensing Images With Iterative Contrastive Network
IEEE GEOSCIENCE AND REMOTE SENSING LETTERS(2022)
摘要
With the development of deep learning, semantic segmentation of remote sensing images has made great progress. However, segmentation algorithms based on deep learning usually require a huge number of labeled images for model training. For remote sensing images, pixel-level annotation usually consumes expensive resources. To alleviate this problem, this letter proposes a semi-supervised segmentation method of remote sensing images based on an iterative contrastive network. This method combines few labeled images and more unlabeled images to significantly improve the model performance. First, contrastive networks continuously learn more potential information by using better pseudo labels. Then, the iterative training method keeps the differences between models to better improve the segmentation performance. The semi-supervised experiments on different remote sensing datasets prove that this method has a better performance than the related methods. Code is available at
https://github.com/VCISwang/ICNet
.
更多查看译文
关键词
Training, Image segmentation, Predictive models, Remote sensing, Data models, Iterative methods, Semantics, Contrastive network, remote sensing, semantic segmentation, semi-supervised learning
AI 理解论文
溯源树
样例
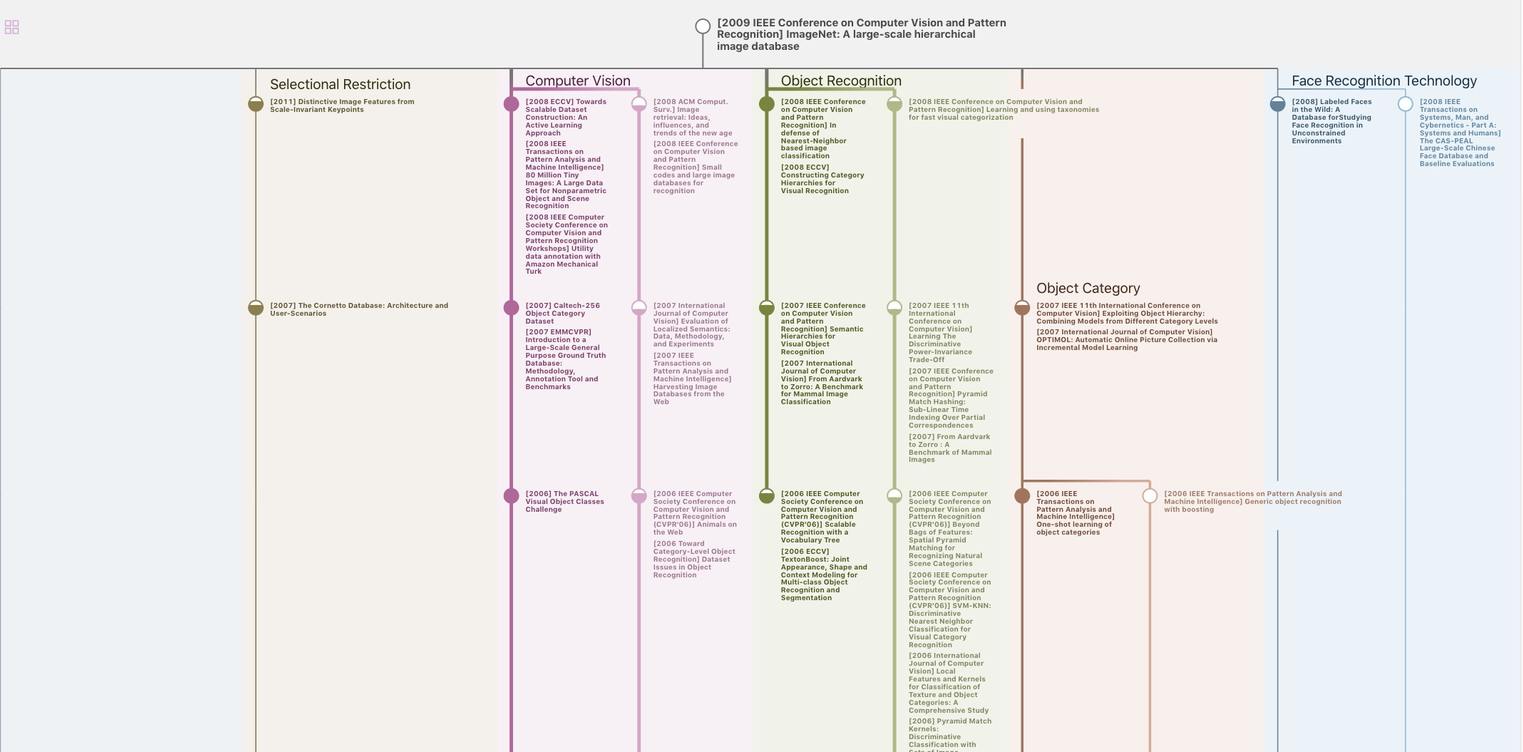
生成溯源树,研究论文发展脉络
Chat Paper
正在生成论文摘要