RESHAPE: Reverse-Edited Synthetic Hypotheses for Automatic Post-Editing
IEEE ACCESS(2022)
摘要
Synthetic training data has been extensively used to train Automatic Post-Editing (APE) models in many recent studies because the quantity of human-created data has been considered insufficient. However, the most widely used synthetic APE dataset, eSCAPE, overlooks respecting the minimal editing property of genuine data, and this defect may have been a limiting factor for the performance of APE models. This article suggests adapting back-translation to APE to constrain edit distance, while using stochastic sampling in decoding to maintain the diversity of outputs, to create a new synthetic APE dataset, RESHAPE. Our experiments show that (1) RESHAPE contains more samples resembling genuine APE data than eSCAPE does, and (2) using RESHAPE as new training data improves APE models' performance substantially over using eSCAPE.
更多查看译文
关键词
Decoding, Data models, Training data, Training, Limiting, Feeds, Transformers, Automatic post-editing, back-translation, decoding strategy, machine translation, synthetic data generation
AI 理解论文
溯源树
样例
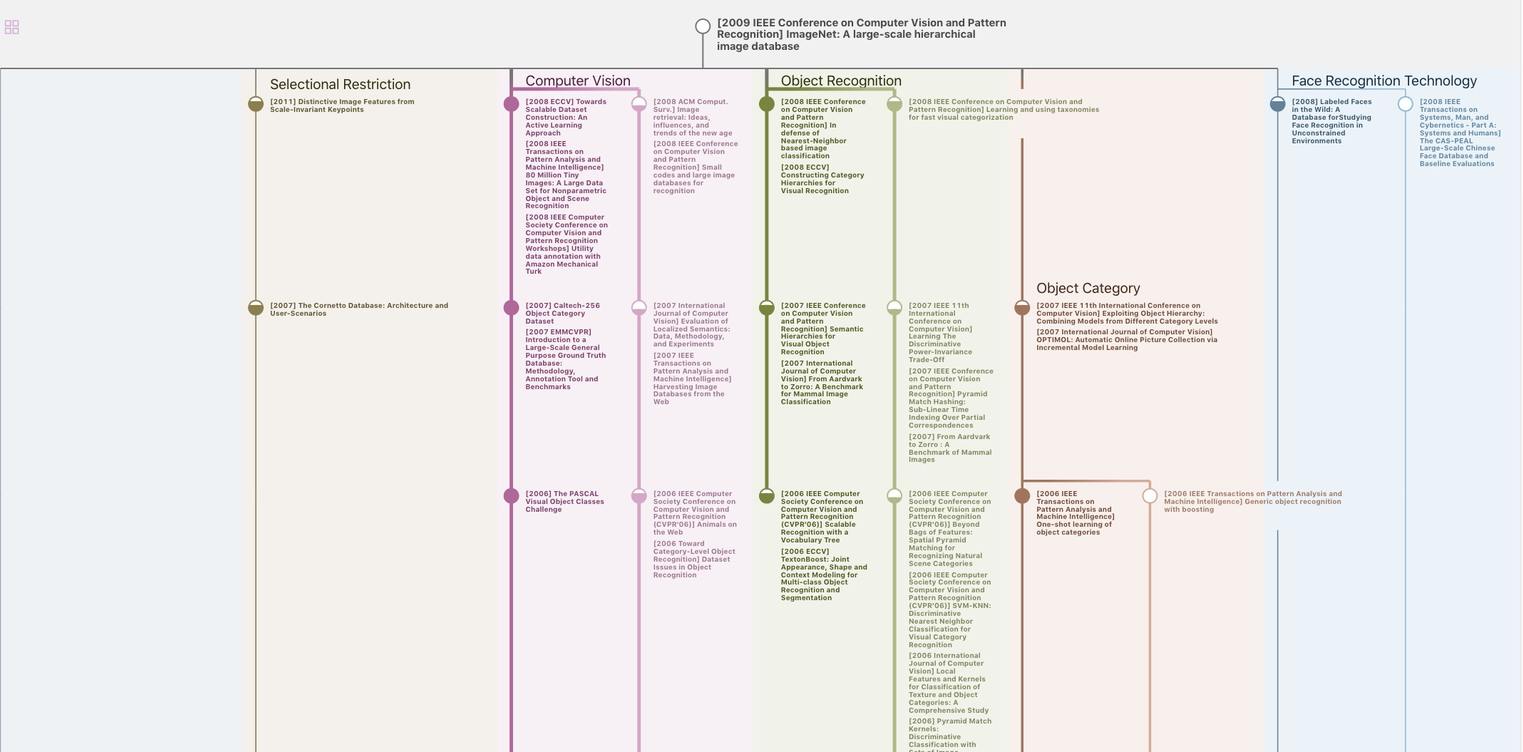
生成溯源树,研究论文发展脉络
Chat Paper
正在生成论文摘要